Box-Supervised Instance Segmentation with Level Set Evolution
COMPUTER VISION, ECCV 2022, PT XXIX(2022)
摘要
In contrast to the fully supervised methods using pixel-wise mask labels, box-supervised instance segmentation takes advantage of the simple box annotations, which has recently attracted a lot of research attentions. In this paper, we propose a novel single-shot box-supervised instance segmentation approach, which integrates the classical level set model with deep neural network delicately. Specifically, our proposed method iteratively learns a series of level sets through a continuous Chan-Vese energy-based function in an end-to-end fashion. A simple mask supervised SOLOv2 model is adapted to predict the instance-aware mask map as the level set for each instance. Both the input image and its deep features are employed as the input data to evolve the level set curves, where a box projection function is employed to obtain the initial boundary. By minimizing the fully differentiable energy function, the level set for each instance is iteratively optimized within its corresponding bounding box annotation. The experimental results on four challenging benchmarks demonstrate the leading performance of our proposed approach to robust instance segmentation in various scenarios.
更多查看译文
关键词
Instance segmentation,Level set,Box supervision
AI 理解论文
溯源树
样例
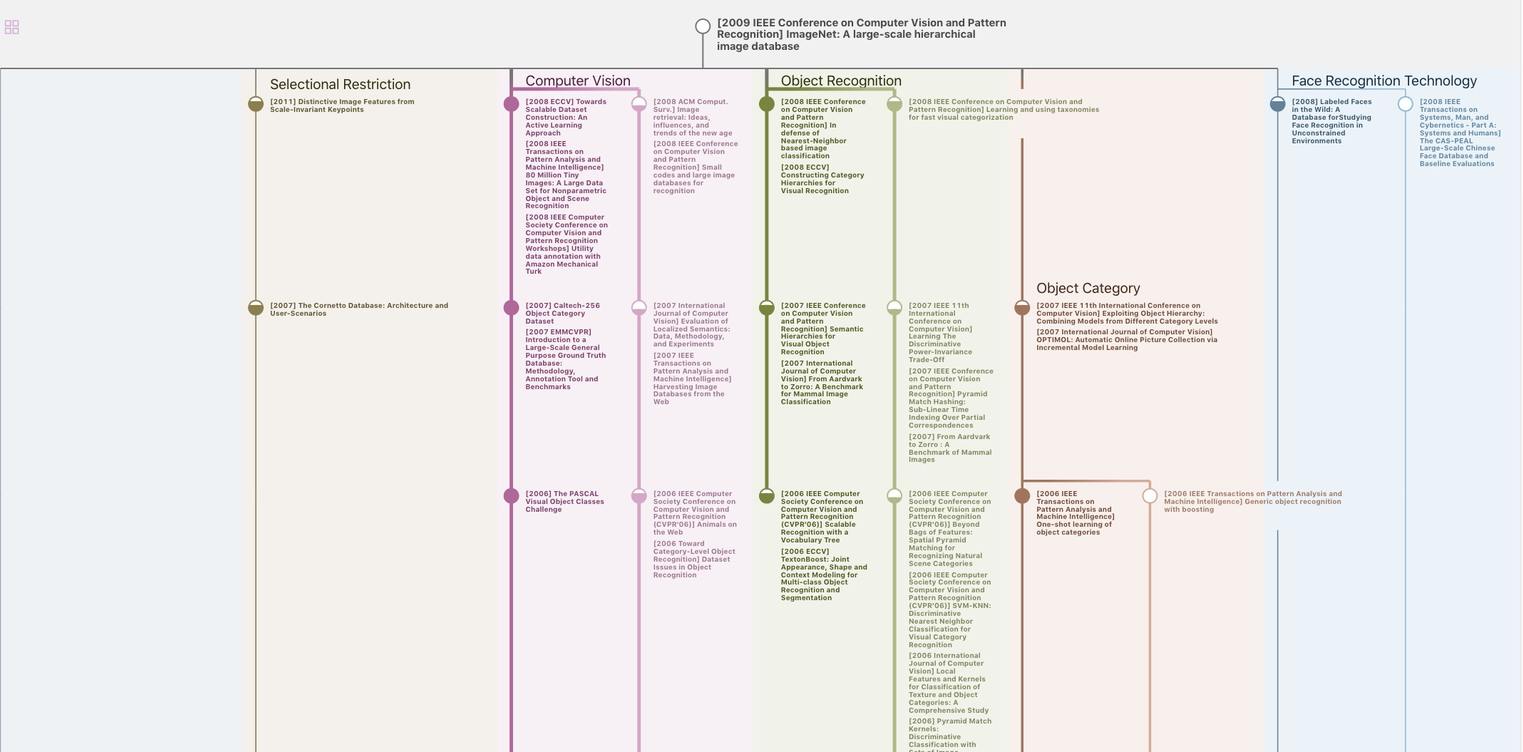
生成溯源树,研究论文发展脉络
Chat Paper
正在生成论文摘要