Knowledge graph embedding by logical-default attention graph convolution neural network for link prediction
Information Sciences(2022)
摘要
The purpose of link prediction is to predict missing edges of the knowledge graph, or edges that may appear in the future. An abundance of data-driven methods exist, which rely on many labels and only consider the structural information about the graph. To address these problems, we have developed a logical-default attention graph convolution neural network model (DA-GCN). The model consists of three parts. First, the confidence of first-order logic inference with Default (DFLIN) was calculated by defining rules. Second, a local relational attention model was defined to represent self information, which includes first order, second order neighbor node information, and to train a graph convolution neural network based on iterative attention (IAGCN) by using centralized training distributed execution model. Third, iteratively update DFLIN and IAGCN using the Wasserstein distance to express the relation between entities. Finally, we conduct comparative experiments between the DA-GCN model and other models on a self-built dataset and three public datasets. The results show that the Mean Reciprocal Rank (MRR) value is about 36.48% higher than that of the R-GCN model. Our results show that DA-GCN achieves state-of-the-art performance compared to other specific or general integrated methods, especially on small-scale organized knowledge graphs.
更多查看译文
关键词
Link prediction,Default logic,GCN,Wasserstein distance,Iterative
AI 理解论文
溯源树
样例
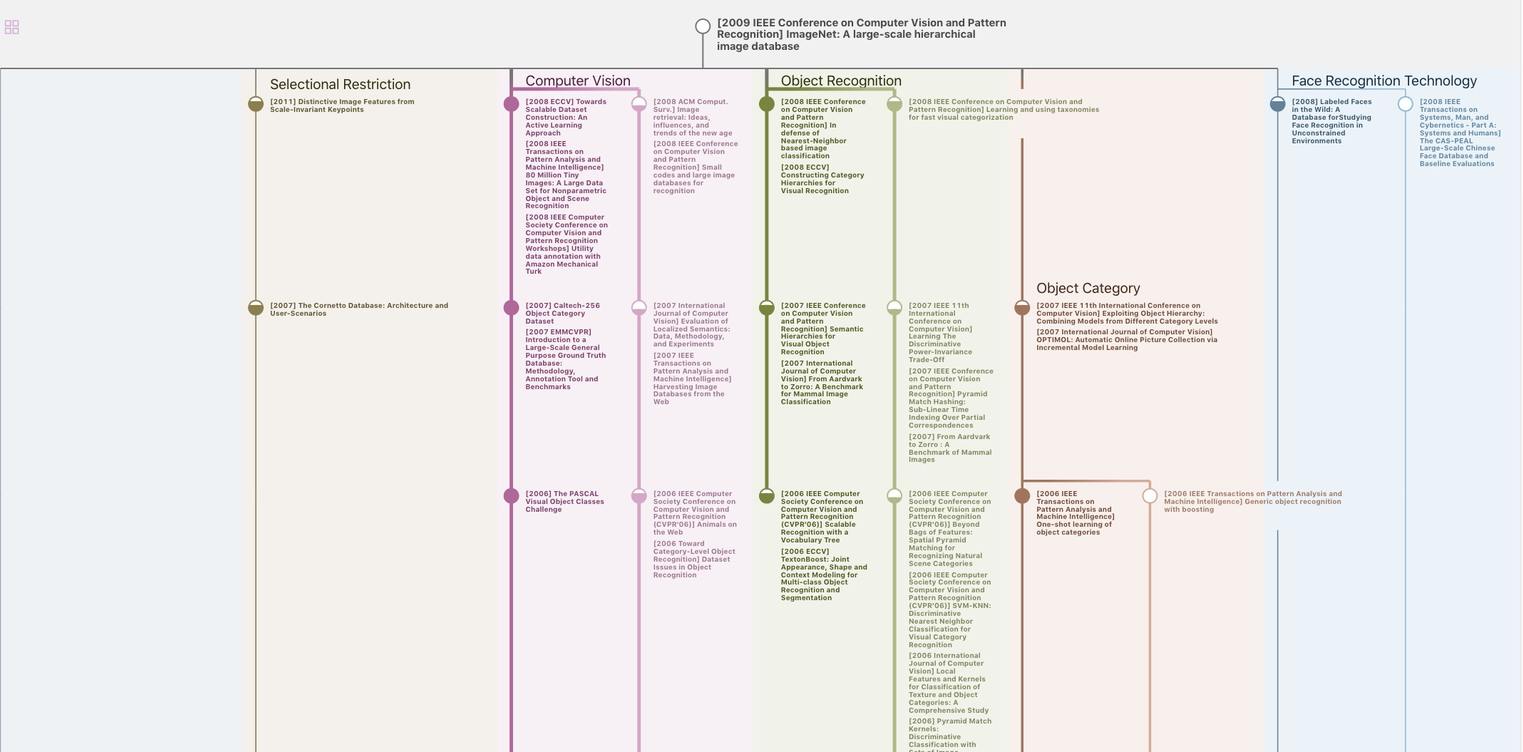
生成溯源树,研究论文发展脉络
Chat Paper
正在生成论文摘要