Algorithmic Connections between Active Learning and Stochastic Convex Optimization.
Algorithmic Learning Theory (ALT 2013)(2015)
摘要
Interesting theoretical associations have been established by recent papers between the fields of active learning and stochastic convex optimization due to the common role of feedback in sequential querying mechanisms. In this paper, we continue this thread in two parts by exploiting these relations for the first time to yield novel algorithms in both fields, further motivating the study of their intersection. First, inspired by a recent optimization algorithm that was adaptive to unknown uniform convexity parameters, we present a new active learning algorithm for one-dimensional thresholds that can yield minimax rates by adapting to unknown noise parameters. Next, we show that one can perform d-dimensional stochastic minimization of smooth uniformly convex functions when only granted oracle access to noisy gradient signs along any coordinate instead of real-valued gradients, by using a simple randomized coordinate descent procedure where each line search can be solved by 1-dimensional active learning, provably achieving the same error convergence rate as having the entire real-valued gradient. Combining these two parts yields an algorithm that solves stochastic convex optimization of uniformly convex and smooth functions using only noisy gradient signs by repeatedly performing active learning, achieves optimal rates and is adaptive to all unknown convexity and smoothness parameters.
更多查看译文
关键词
Active Learning, Line Search, Coordinate Descent, Uniform Convexity, Strong Convexity
AI 理解论文
溯源树
样例
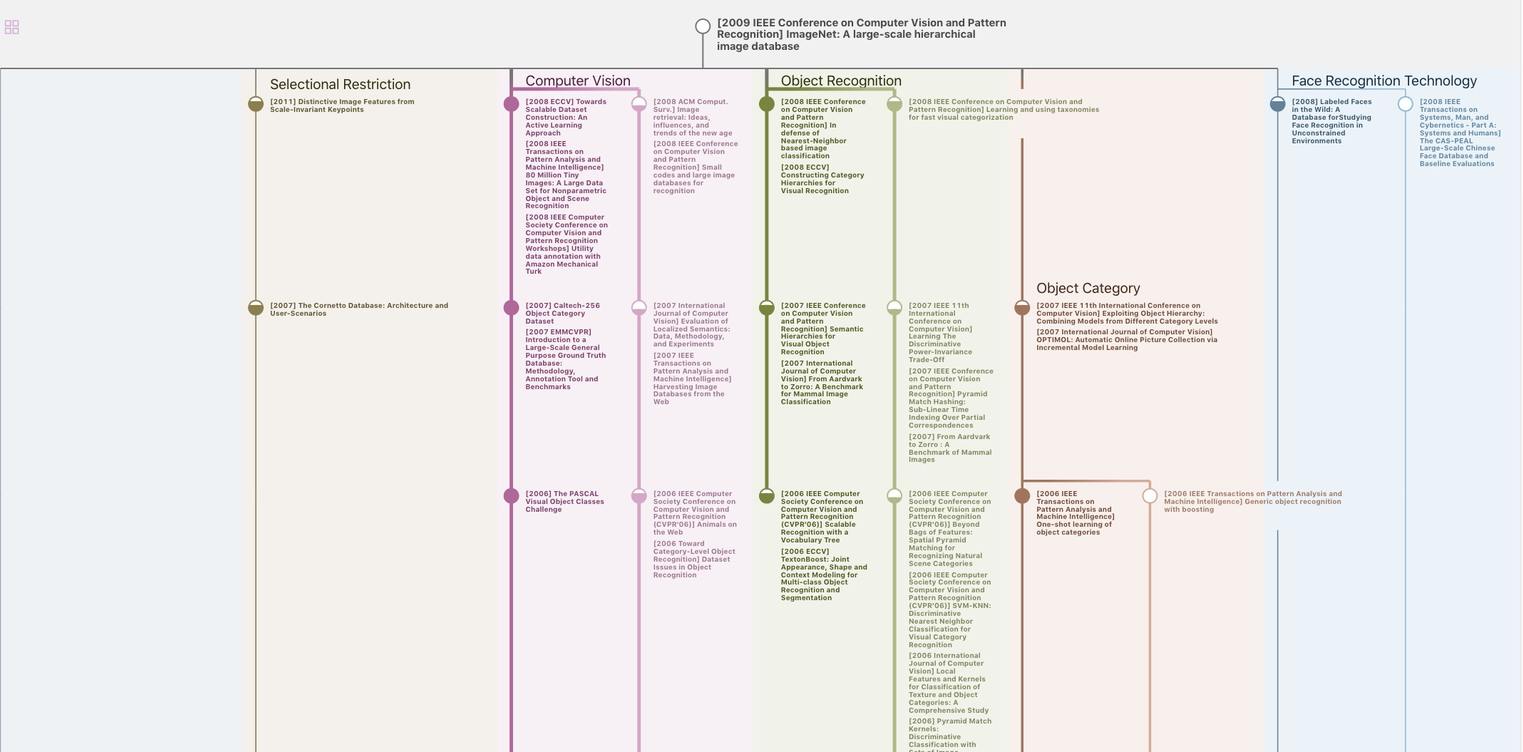
生成溯源树,研究论文发展脉络
Chat Paper
正在生成论文摘要