Supporting Computer-Interpretable Guidelines' Modeling By Automatically Classifying Clinical Actions
Lecture Notes in Computer Science(2013)
摘要
Modeling computer-interpretable clinical practice guidelines is a complex and tedious task that has been of interest for several attempts to automate parts of this process. When modeling guidelines one of the tasks is to specify common actions in everyday's practical medicine (e.g., drug prescription, observation) in order to link them with clinical information systems (e.g., an orderentry system). In this paper we compare a rule-based and a machine-learning method to classify activities according to the Clinical Actions Palette used in the Hybrid-Asbru ontology. We use syntactic and semantic features, such as the Semantic Types of the UMLS to classify the activities. Furthermore, we extend our methods by using 2-step classification and combining machine learning and rule-based approaches. Results show that machine learning performs better than the rule-based method on the classification task. They also show that the 2-step classification method improves the categorization of activities.
更多查看译文
关键词
Clinical Practice Guidelines,Hybrid-Asbru,Common Clinical Actions,Natural Language Processing,Classification
AI 理解论文
溯源树
样例
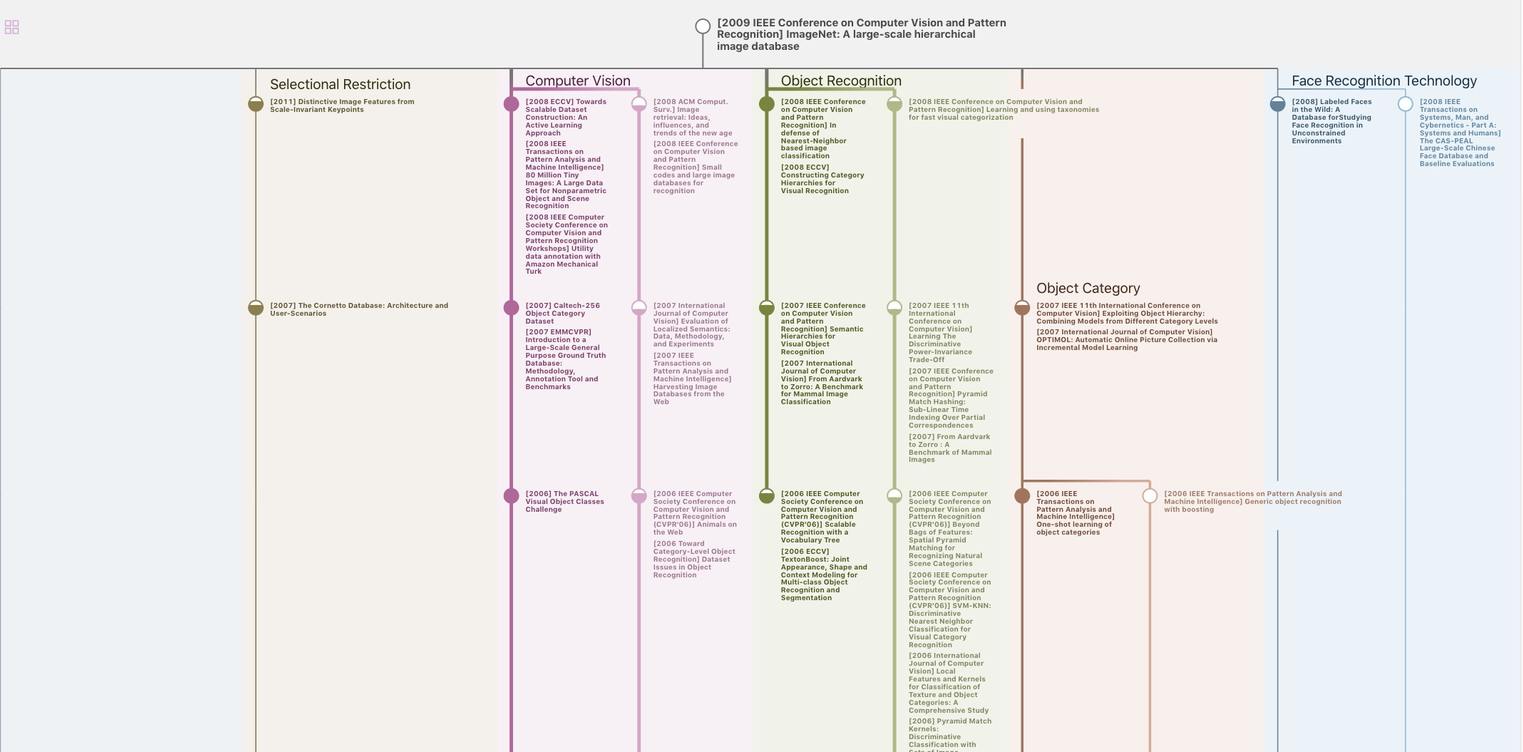
生成溯源树,研究论文发展脉络
Chat Paper
正在生成论文摘要