Hierarchical Incomplete Multi-source Feature Learning for Spatiotemporal Event Forecasting
KDD(2016)
摘要
Forecasting significant societal events is an interesting and challenging problem as it taking into consideration multiple aspects of a society, including its economics, politics, and culture. Traditional forecasting methods based on a single data source find it hard to cover all these aspects comprehensively, thus limiting model performance. Multi source event forecasting has proven promising but still suffers from several challenges, including 1) geographical hierarchies in multi-source data features, 2) missing values, and 3) characterization of structured feature sparsity. This paper proposes a novel feature learning model that concurrently addresses all the above challenges. Specifically, given multi-source data from different geographical levels, we design a new forecasting model by characterizing the lower-level features' dependence on higher-level features. To handle the correlations amidst structured feature sets and deal with missing values among the coupled features, we propose a novel feature learning model based on an $N$th-order strong hierarchy and fused-overlapping group Lasso. An efficient algorithm is developed to optimize model parameters and ensure global optima. Extensive experiments on 10 datasets in different domains demonstrate the effectiveness and efficiency of the proposed model.
更多查看译文
关键词
Event forecasting,multiple data sources,feature selection
AI 理解论文
溯源树
样例
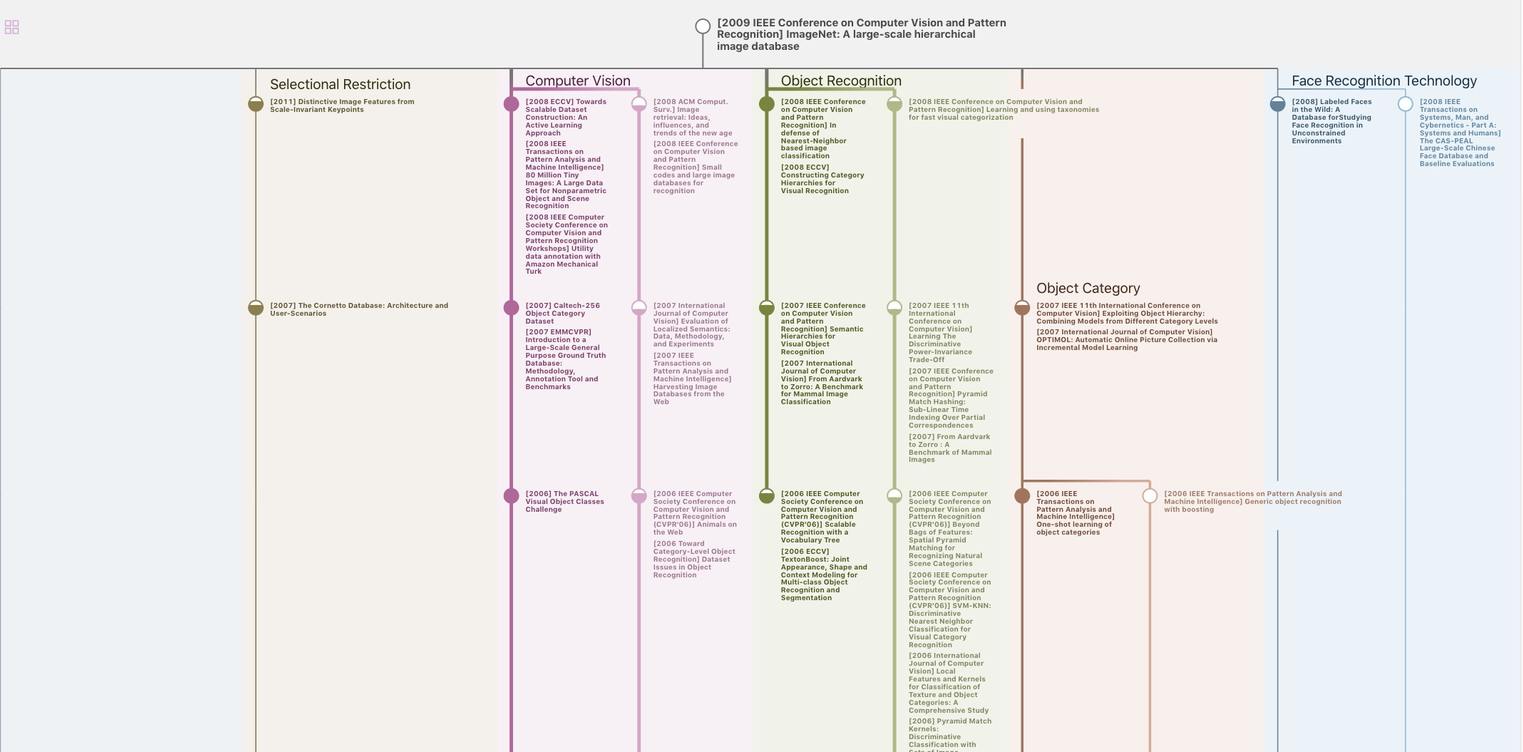
生成溯源树,研究论文发展脉络
Chat Paper
正在生成论文摘要