Cherry-Picking Gradients - Learning Low-Rank Embeddings of Visual Data via Differentiable Cross-Approximation.
ICCV(2021)
摘要
We propose an end-to-end trainable framework that processes large-scale visual data tensors by looking \emph{at a fraction of their entries only}. Our method combines a neural network encoder with a \emph{tensor train decomposition} to learn a low-rank latent encoding, coupled with cross-approximation (CA) to learn the representation through a subset of the original samples. CA is an adaptive sampling algorithm that is native to tensor decompositions and avoids working with the full high-resolution data explicitly. Instead, it actively selects local representative samples that we fetch out-of-core and on-demand. The required number of samples grows only logarithmically with the size of the input. Our implicit representation of the tensor in the network enables processing large grids that could not be otherwise tractable in their uncompressed form. The proposed approach is particularly useful for large-scale multidimensional grid data (e.g., 3D tomography), and for tasks that require context over a large receptive field (e.g., predicting the medical condition of entire organs). The code will be available at https://github.com/aelphy/c-pic
更多查看译文
关键词
Machine learning architectures and formulations,Efficient training and inference methods,Optimization and learning methods
AI 理解论文
溯源树
样例
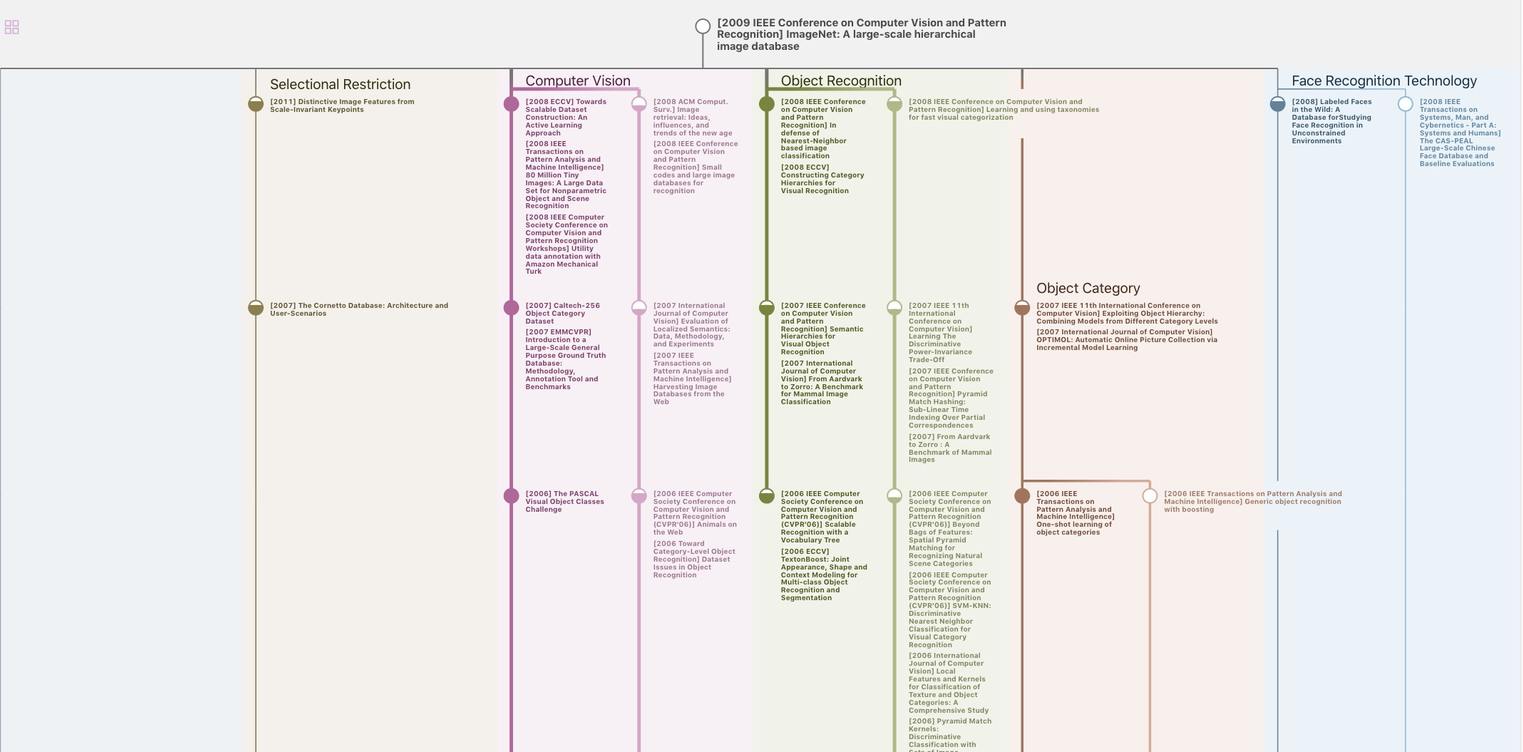
生成溯源树,研究论文发展脉络
Chat Paper
正在生成论文摘要