PLS for designed experiments
CHEMOMETRICS AND INTELLIGENT LABORATORY SYSTEMS(2023)
摘要
In many emerging new areas of research, for example in -omics sciences, complex data sets are generated implementing well-designed experiments with the aim to study the effects of factors on responses measured by high-through analytical platforms. The data sets are multivariate in their nature and require suitable data analysis techniques to be investigated. Partial Least Squares (PLS) regression has been developed and successfully applied to solve multivariate regression problems where data are correlated, redundant and noisy, but it is not able to take explicitly into account the experimental design used to generate the data. In this study, we adapt PLS to model data generated by crossed factorial designs. A new method based on constrained PLS2 regression and a type III sums of squares-like approach based on PLS2 are developed to treat orthogonal and non-orthogonal designs, respectively. Post-transformation and Procrustes analysis are applied to discover the 'latent' structure useful to investigate the relationships between factors and responses. The mathematical properties of the data decomposition, the inference process to assess the reliability of the model and some basic tools for model interpretation are presented and discussed. One simulated and two experimental data sets, one generated implementing an orthogonal design and one obtained by a non-orthogonal design, are investigated to show how the methodology works in practice.
更多查看译文
关键词
PLS regression,Designed experiments,Latent variables
AI 理解论文
溯源树
样例
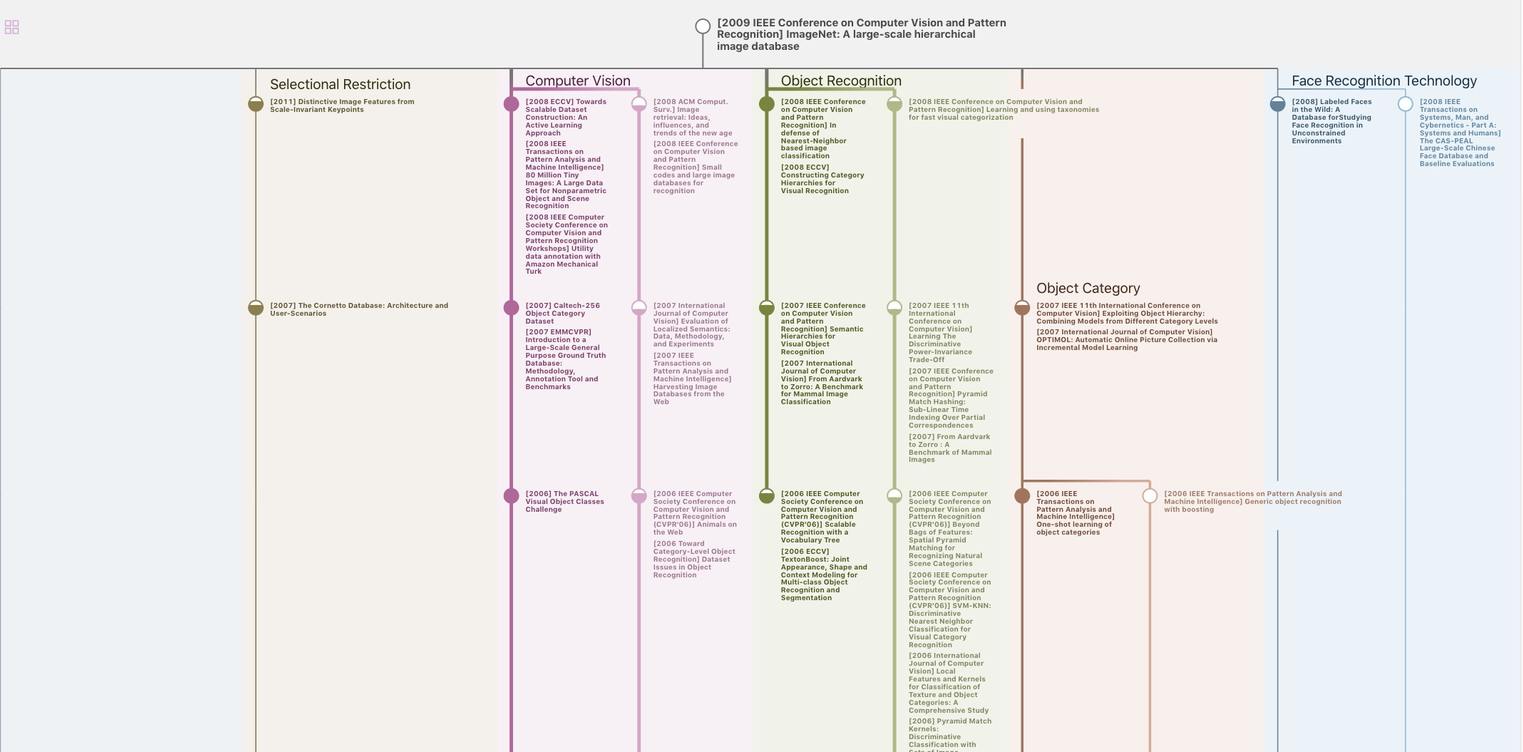
生成溯源树,研究论文发展脉络
Chat Paper
正在生成论文摘要