Sensitivity of climate data assimilation to transport models and observational networks
msra(2009)
Abstract
This paper investigates the sensitivity of carbon fluxes inferred from the assimilation system CCDAS (Carbon Cycle Data Assimilation System) to two observational operators. CCDAS is built around the terrestrial biosphere model BETHY (Biosphere Energy Transfer Hydrology) and coupled to atmospheric transport models. We test the sensitivity of CCDAS carbon fluxes to two representations of the transport model TM (TM2 and TM3) and two networks (38 and 68 stations). Results show that the patterns of the net ecosystem productivity produced by CCDAS are more sensitive to the behaviour of the transport model while those of gross primary productivity (GPP) depend more on the observing network. Further investigations are being made by coupling CCDAS with an on-line transport model (LMDZt) together with high frequency continuous data in order to quantify the information added by the use of such a system. INTRODUCTION Direct inverse modelling of atmospheric data has been widely used to infer carbon sources and sinks. However estimates have shown large spread. This is attributable to different set-up (prior estimates, data etc) and transport models [1,2]. At the same time, data assimilation systems using process models to interpret atmospheric data are gaining prominence [3,4]. This study, using the CCDAS system [4] investigates the sensitivity of such a system to the transport model and atmospheric network. CCDAS SYSTEM AND SET UP CCDAS is a versatile assimilation system built around the terrestrial biosphere model BETHY (Biosphere Energy Transfer Hydrology), which can be coupled to various atmospheric transport models. CCDAS consists of a biosphere model BETHY [5] and an atmospheric transport model, together with CO2 fluxes representing ocean flux, land use change and fossil fuel emission [4,6]. CCDAS has two main modes of operation. The optimization/calibration mode, which allows calibrating the physical process parameters of BETHY via a comparison of observed and modelled CO2 concentrations. The optimized model is then used to calculate diagnostic [4] and prognostic [6] quantities. BETHY is a process-based model of the terrestrial biosphere which simulates carbon assimilation and plant and soil respiration, embedded within a full energy and water balance [3]. It uses 13 plant functional types. The version here is implemented on a 2°x2° grid and is driven by climate and radiation data. In this study, the sensitivities at 41 and 68 sites for CO2 concentration observations over 1980-2000 time period using the models TM2 [7] (8° latitude by 10° longitude) and TM3 [8] (3.75° latitude by 5° longitude), are considered. Three sets of optimized parameters of BETHY are computed and defined as follows. TM2/41: TM2 and 41sites, TM3/38*: TM3 and 38 sites, TM3/68: TM3 and 68 sites. SOME RESULTS Figure 1 shows NEP for the 3 cases. Largest differences are driven by the choice of transport model and are seen in the Northern Hemisphere. Figure 2 shows GPP for the 3 cases. The network drives the biggest differences. The largest differences are found in the tropics, i.e., where more additional stations were considered. To see whether tropical stations were sensitive to the change, CO2 _________________ *The data for the two models is computed slightly differently but we repeated TM3/38 using an exact subset of the TM2/41 data and produced very similar results to TM3/38. concentrations were simulated at the 30 additional sites of TM3/68 but using parameters from TM3/38. Results are found to be very close to the simulation using TM3/68 parameters. Thus the extra 30 sites do not bring much extra information on GPP. This is also consistent with the posterior uncertainty. Finally, surface CO2 concentrations were again calculated with the global climate model LMDZ-INCA [9] (3.75° latitude by 2.5° longitude) using NEP from TM3/38 and TM3/68. Results show only small differences at the stations (consistent with small change in cost function), but large differences elsewhere (Figure 3). In summary, in CCDAS, NEP is more sensitive to the choice of transport model while GPP responds to changing observing networks. The optimal measurement networks to constrain productivity are different from those to constrain net flux. CONCLUSIONS AND PERSPECTIVES Our investigations show that patterns of NEP produced by CCDAS are more sensitive to the behaviour of the transport model while those of GPP depend more on the atmospheric observing network. Thus, a network that can constrain the NEP is not necessary optimized to constrain the GPP. A denser atmospheric network, e.g., satellite CO2 measurements, may be used to constrain the GPP. Finally, the direct measurement of CO2 fluxes in some sensitive areas such as the Tropics may help to better constrain the GPP. Further investigations are being made by coupling CCDAS with an on-line transport model (LMDZt) together with high frequency continuous data in order to quantify the information added by the use of such a system. Preliminary results will be shown. REFERENCES [1] Gurney, K. R., et al., (2004). TransCom 3 inversion intercomparison : Model mean results for the estimation of seasonal carbon sources and sinks, Global Biogechem. Cycles, 18, GB1010, doi:10.1029/2003GB002111 [2] Baker et al., (2006), TransCom 3 inversion intercomparison: Impact of transport model errors on the interannual variability of regional C02 fluxes, 1988-2003, Global Biogechem. Cycles, 20, GB1002, doi:10.1029/2004GB002439 [3] Kaminski T. et al. (2002), Assimilating atmospheric data into a terrestrial biosphere model: A case study of the seasonal cycle, Global Biogechen. Cycles, 16(4), a1066 doi:10.1029/2001GB001463 [4] Rayner, P.J. et al. (2005), Two decades of terrestrial carbon fluxes from a carbon cycle data assimilation system (CCDAS), Global Biogechem. Cycles, 19, GB2026, Doi:10..1029/2004GB002254 [5] Knorr W. (2000), Annual and interannual CO2 exchanges of the terrestrial biosphere: process based simulations and uncertainties, Glob. Ecol. And Biogeogr., 9:225-252 [6] Scholze, M. et al. (2007), Propagating uncertainty through prognostic carbon cycle data assimilation system simulations. J. Geophys. Res., 112, D17305, doi:10.1029/2007JD008642 [7] Heimann, M (1995). The global atmospheric tracer model TM2. Technical Report No. 10, Max-Planck-Intitut für Meteorologie, Hamburg, Germany, 1995, 9, 11 [8] Heimann M. and, S. Körner (2003): The global atmospheric tracer model TM3. In: Max-Planck-Institut für Biogeochemie (Eds.): Technical Report. Vol. 5. Max-PlanckInstitut für Biogeochemie, Jena. pp. 131 [BGC0601; ECO140/036+5] [9] Hauglustaine, D. A. et al. (2004), Interactive chemistry in the Laboratoire de Météorologie Dynamique general circulation model: Description and background tropospheric chemistry evaluation, J. Geophys. Res., 109, D04314, doi:10.1029/2003JD003957. Figure 1: Map of Net Ecosystem Production (NEP in kgC/m2.yr) for simulations using TM2 (left), TM3 with 68 stations (TM3/68: centre) and TM3 using 38 stations (TM3/38: right)) 10
MoreTranslated text
AI Read Science
Must-Reading Tree
Example
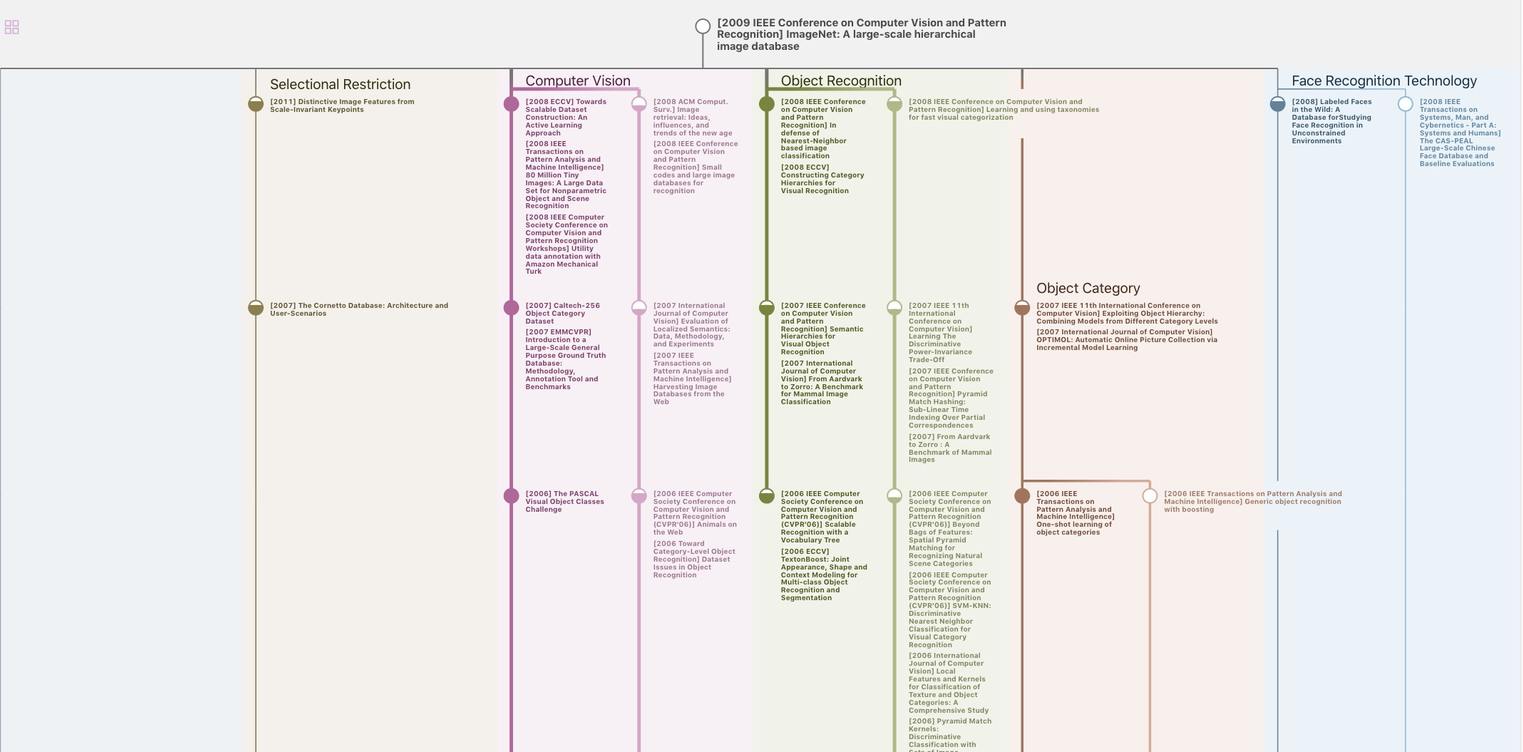
Generate MRT to find the research sequence of this paper
Chat Paper
Summary is being generated by the instructions you defined