Self-training with unlabeled regions for NBI image recognition
ICPR(2012)
摘要
In this paper, we propose a self-training method which uses unlabeled regions in the original images obtained from a colorectal Narrow Band Imaging (NBI) zoom-video endoscope. The proposed method first trims a number of patches from unlabeled regions in the original images and uses them as unlabeled training samples. Classifiers are trained with the available labeled samples, as well as with those unlabeled training samples, using a newly-proposed rejection condition which takes into account the class asymmetry of the NBI images. Experimental results demonstrate that the proposed method improves performance with a statistically significant difference.
更多查看译文
关键词
nbi zoom-videoendoscope,video signal processing,endoscopes,class asymmetry,colorectal narrow band imaging zoom-videoendoscope,newly-proposed rejection condition,statistical analysis,unlabeled training samples,nbi image recognition unlabeled regions,classifier training,image sampling,cancer,image classification,self-training method,unlabeled regions,medical image processing,statistically significant difference
AI 理解论文
溯源树
样例
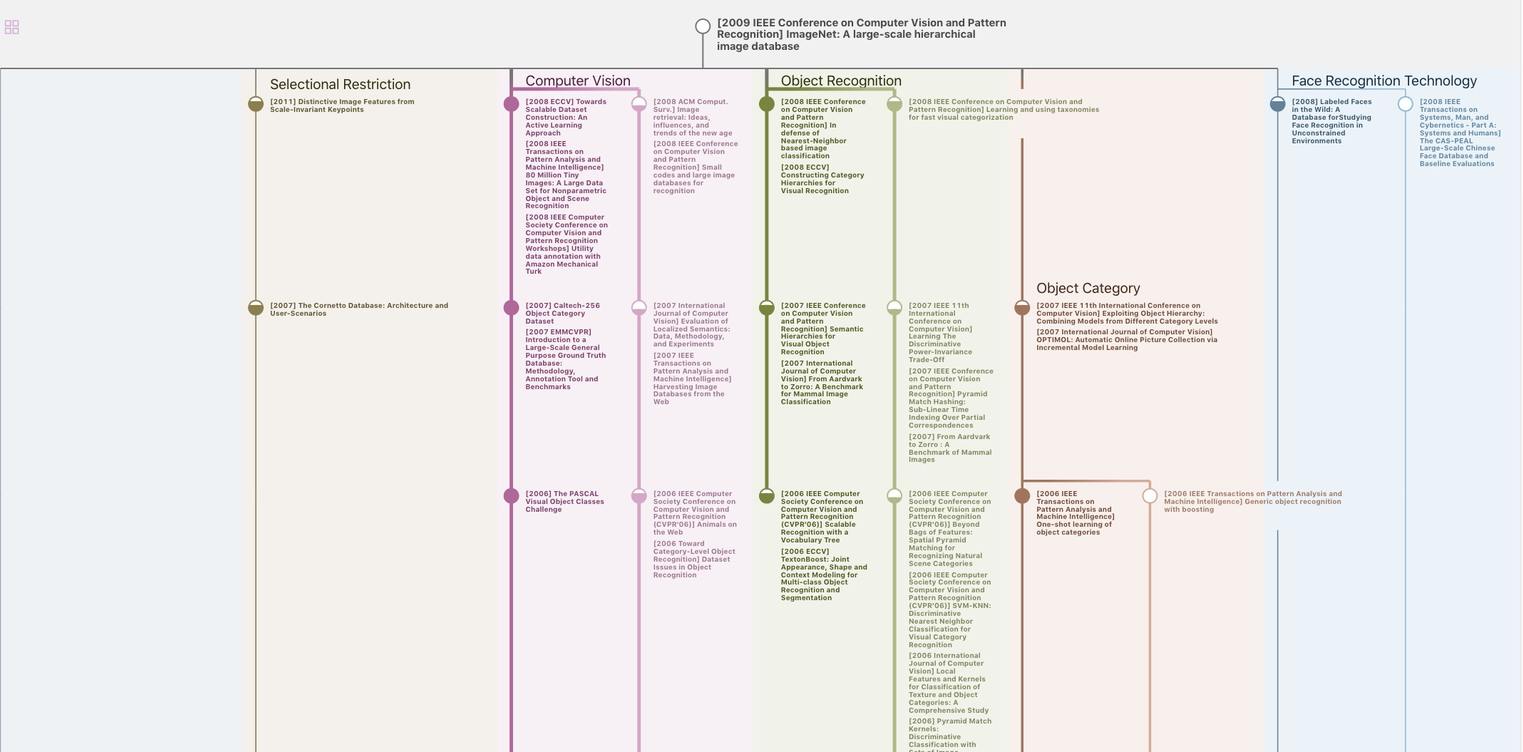
生成溯源树,研究论文发展脉络
Chat Paper
正在生成论文摘要