Speed and Sparsity of Regularized Boosting
AISTATS(2009)
摘要
Boosting algorithms withl1-regularization are of interest becausel1 regularization leads to sparser composite classifiers. Moreover, Rosset et al. have shown that for separable data, standard lp- regularized loss minimization results in a margin maximizing classifier in the limit as regulariza- tion is relaxed. For the case p = 1, we ex- tend these results by obtaining explicit conver- gence bounds on the regularization required to yield a margin within prescribed accuracy of the maximum achievable margin. We derive simi- lar rates of convergence for the"-AdaBoost algo- rithm, in the process providing a new proof that "-AdaBoost is margin maximizing as" converges to 0. Because both of these known algorithms are computationally expensive, we introduce a new hybrid algorithm, AdaBoost+L1, that combines the virtues of AdaBoost with the sparsity of l1- regularization in a computationally efficient fash- ion. We prove that the algorithm is margin maxi- mizing and empirically examine its performance on five datasets.
更多查看译文
关键词
hybrid algorithm,rate of convergence
AI 理解论文
溯源树
样例
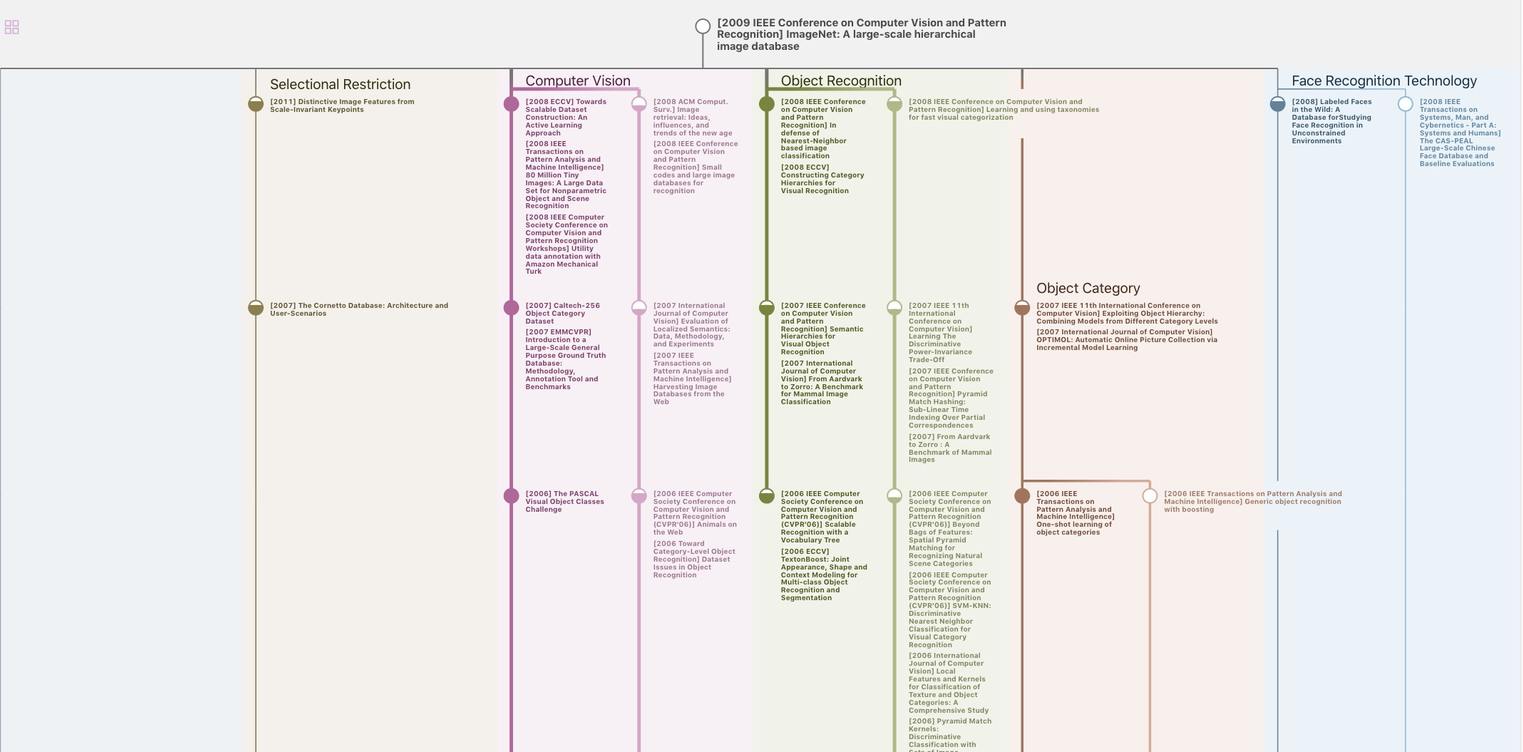
生成溯源树,研究论文发展脉络
Chat Paper
正在生成论文摘要