Sidekick agents for sequential planning problems
Sidekick agents for sequential planning problems(2013)
摘要
Effective AI sidekicks must solve the interlinked problems of understanding what their human collaborator's intentions are and planning actions to support them. This thesis explores a range of approximate but tractable approaches to planning for AI sidekicks based on decision-theoretic methods that reason about how the sidekick's actions will effect their beliefs about unobservable states of the world, including their collaborator's intentions. In doing so we extend an existing body of work on decision-theoretic models of assistance to support information gathering and communication actions. We also apply Monte Carlo tree search methods for partially observable domains to the problem and introduce an ensemble-based parallelization strategy. These planning techniques are demonstrated across a range of video game domains. (Copies available exclusively from MIT Libraries, libraries.mit.edu/docs - docs@mit.edu)
更多查看译文
关键词
Monte Carlo tree search,human collaborator,existing body,decision-theoretic model,sequential planning problem,Effective AI sidekick,communication action,MIT Libraries,ensemble-based parallelization strategy,decision-theoretic method,sidekick agent,planning technique
AI 理解论文
溯源树
样例
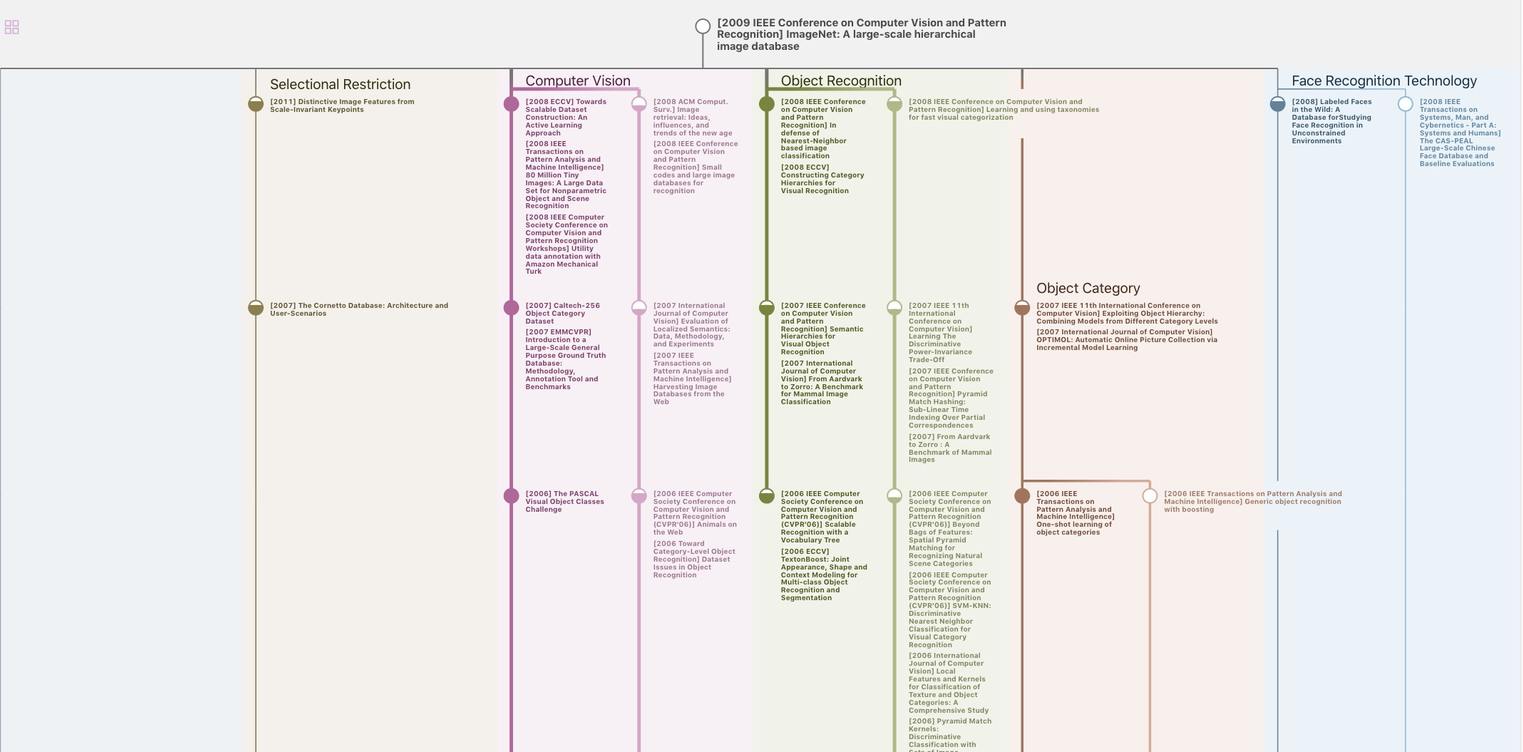
生成溯源树,研究论文发展脉络
Chat Paper
正在生成论文摘要