Two methods for reliable classification of network traffic
Progress in Artificial Intelligence(2012)
摘要
Accurate classification of network traffic can offer substantial benefits to service differentiation, enforcement of security policies and traffic engineering for network operators and service providers. Machine learning algorithms have been used to classify network traffic with good results. However, not knowing the confidence of these classifications makes it difficult to measure and control the risk of error using a decision rule. Modern network resource management systems are becoming increasingly complex and as such require high quality, reliable predictions with confidence measures. These reliability measures allow service provider and network carrier to effectively perform a cost-benefit evaluation of alternative actions and optimise network performance such as delay and information loss. In this paper, we consider the problem of reliable network traffic classification. Two recently developed machine learning methods, namely Conformal Predictor and Venn Probability Machine, are presented for application in network traffic classification. These two methods are based on the identically independently distributed sequence of data instances assumption. Experiments on publicly available real network traffic datasets in the on-line setting show these two methods can perform well and produce reliable classifications. Comparison is also made between these two methods.
更多查看译文
关键词
Network traffic classification,Reliable classification,Conformal predictors,Venn probability machines,Validity,Efficiency
AI 理解论文
溯源树
样例
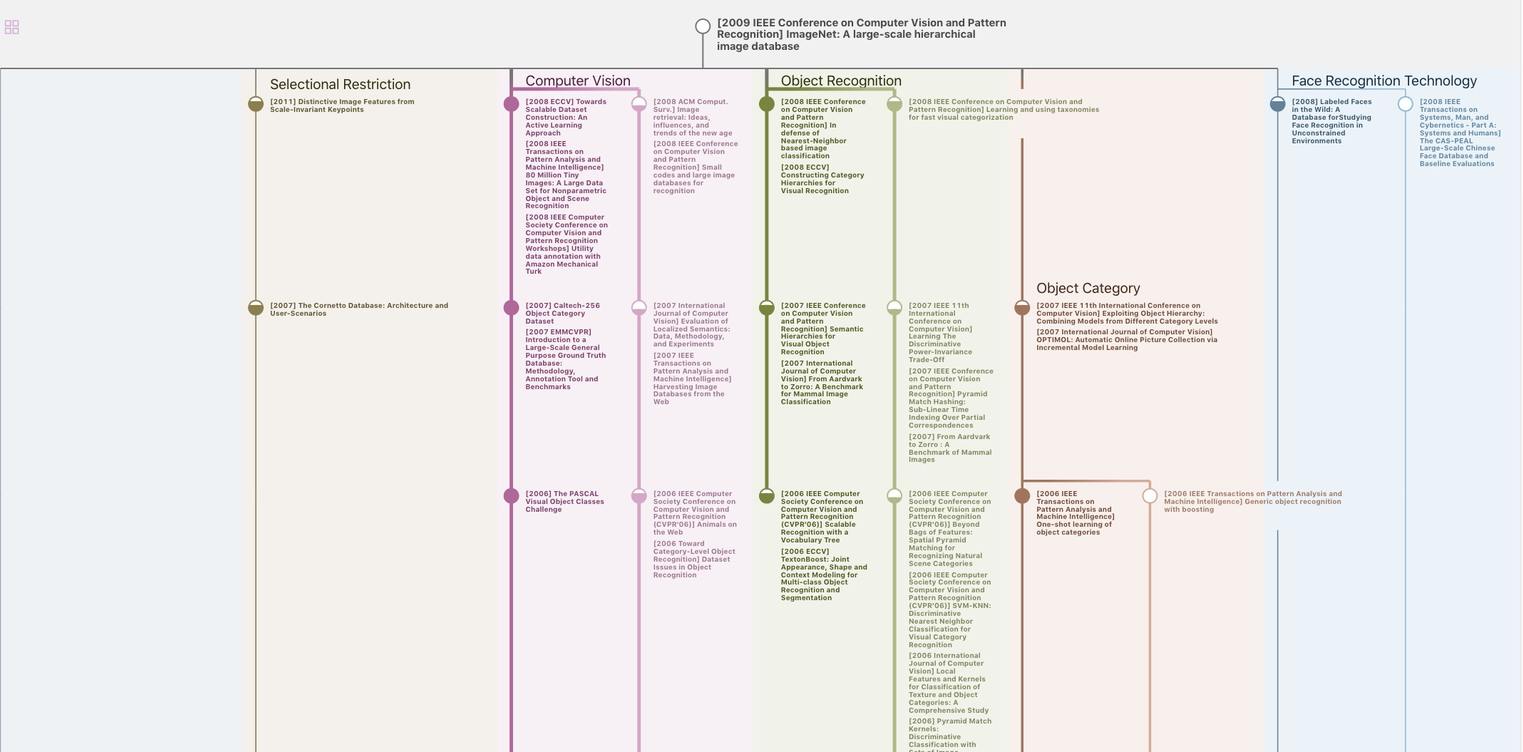
生成溯源树,研究论文发展脉络
Chat Paper
正在生成论文摘要