Point Cloud Attacks in Graph Spectral Domain: When 3D Geometry Meets Graph Signal Processing
IEEE TRANSACTIONS ON PATTERN ANALYSIS AND MACHINE INTELLIGENCE(2024)
摘要
With the increasing attention in various 3D safety-critical applications, point cloud learning models have been shown to be vulnerable to adversarial attacks. Although existing 3D attack methods achieve high success rates, they delve into the data space with point-wise perturbation, which may neglect the geometric characteristics. Instead, we propose point cloud attacks from a new perspective-the graph spectral domain attack, aiming to perturb graph transform coefficients in the spectral domain that correspond to varying certain geometric structures. Specifically, leveraging on graph signal processing, we first adaptively transform the coordinates of points onto the spectral domain via graph Fourier transform (GFT) for compact representation. Then, we analyze the influence of different spectral bands on the geometric structure, based on which we propose to perturb the GFT coefficients via a learnable graph spectral filter. Considering the low-frequency components mainly contribute to the rough shape of the 3D object, we further introduce a low-frequency constraint to limit perturbations within imperceptible high-frequency components. Finally, the adversarial point cloud is generated by transforming the perturbed spectral representation back to the data domain via the inverse GFT. Experimental results demonstrate the effectiveness of the proposed attack in terms of both the imperceptibility and attack success rates.
更多查看译文
关键词
3D geometry,graph Fourier transform (GFT),graph spectral domain,point cloud attack
AI 理解论文
溯源树
样例
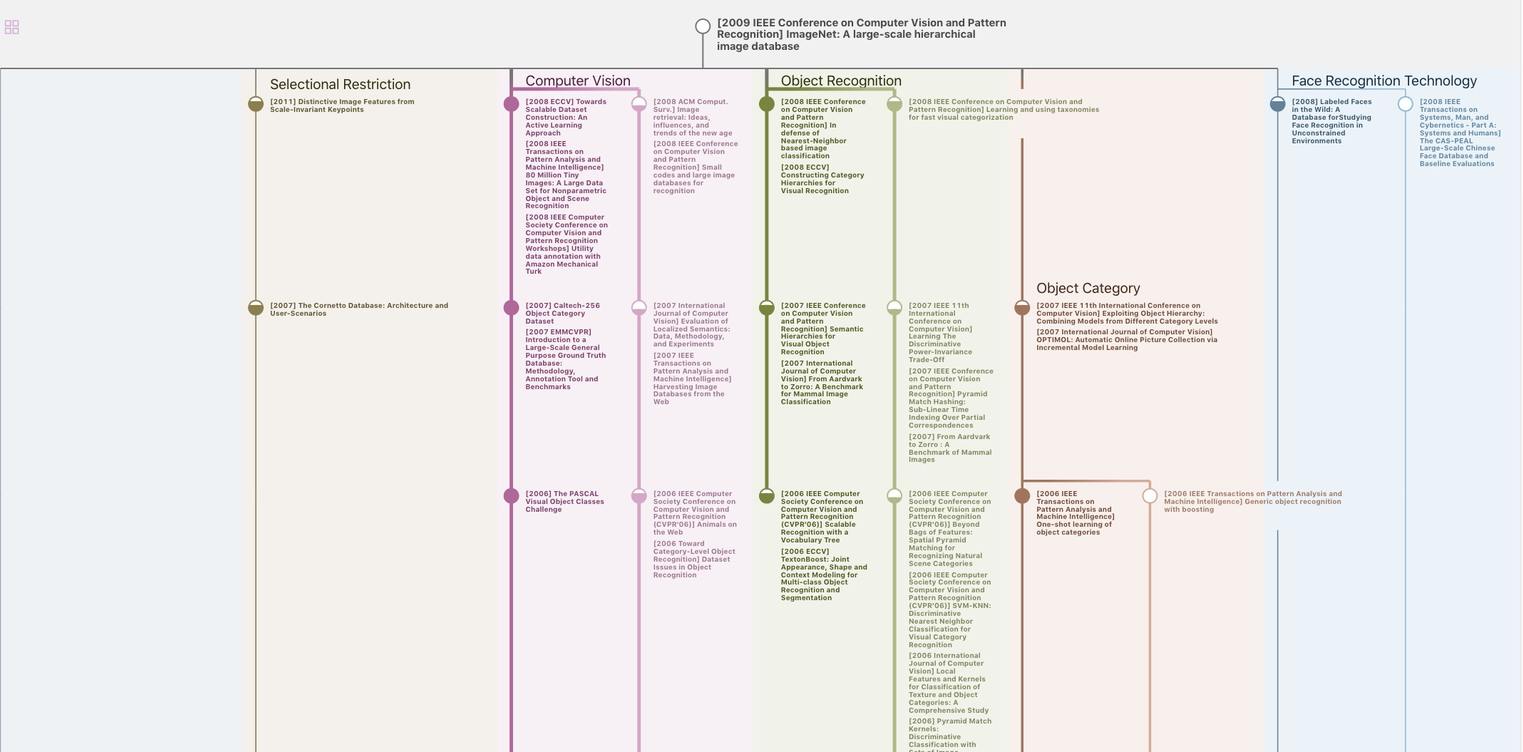
生成溯源树,研究论文发展脉络
Chat Paper
正在生成论文摘要