Stable and Efficient Gaussian Process Calculations
Journal of Machine Learning Research(2009)
摘要
The use of Gaussian processes can be an effective approach to prediction in a supervised learning environment. For large data sets, the standard Gaussian process approach requires solving very large systems of linear equations and approximations are required for the calculations to be practical. We will focus on the subset of regressors approximation technique. We will demonstrate that there can be numerical instabilities in a well known implementation of the technique. We discuss alternate implementations that have better numerical stability properties and can lead to better predictions. Our results will be illustrated by looking at an application involving prediction of galaxy redshift from broadband spectrum data.
更多查看译文
关键词
large system,photometric red- shift,standard gaussian process approach,subset of regressors method,numerical instability,regressors approximation technique,effective approach,gaussian process,large data set,numerical stability property,gaussian processes,numerical stability,better prediction,low rank approximations,broadband spectrum data,efficient gaussian process calculations,linear equations,low rank approximation,spectrum,supervised learning
AI 理解论文
溯源树
样例
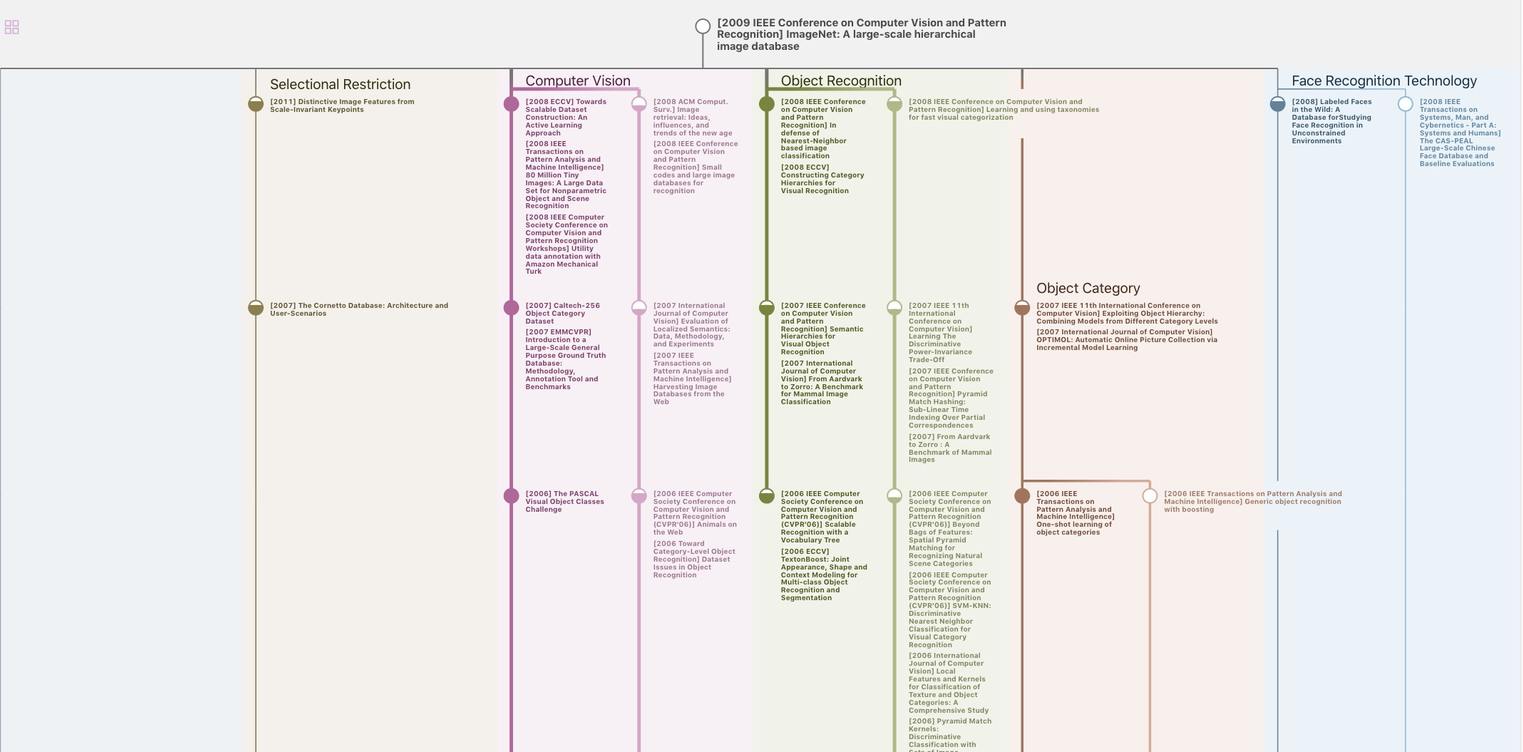
生成溯源树,研究论文发展脉络
Chat Paper
正在生成论文摘要