Partially Observed Dynamic Tensor Response Regression.
Unknown Journal(2023)
摘要
In modern data science, dynamic tensor data prevail in numerous applications. An important task is to characterize the relationship between dynamic tensor datasets and external covariates. However, the tensor data are often only partially observed, rendering many existing methods inapplicable. In this article, we develop a regression model with a partially observed dynamic tensor as the response and external covariates as the predictor. We introduce the low-rankness, sparsity, and fusion structures on the regression coefficient tensor, and consider a loss function projected over the observed entries. We develop an efficient nonconvex alternating updating algorithm, and derive the finite-sample error bound of the actual estimator from each step of our optimization algorithm. Unobserved entries in the tensor response have imposed serious challenges. As a result, our proposal differs considerably in terms of estimation algorithm, regularity conditions, as well as theoretical properties, compared to the existing tensor completion or tensor response regression solutions. We illustrate the efficacy of our proposed method using simulations and two real applications, including a neuroimaging dementia study and a digital advertising study.
更多查看译文
关键词
Alzheimer's disease,Digital advertising,Neuroimaging analysis,Nonconvex optimization,Tensor completion,Tensor regression
AI 理解论文
溯源树
样例
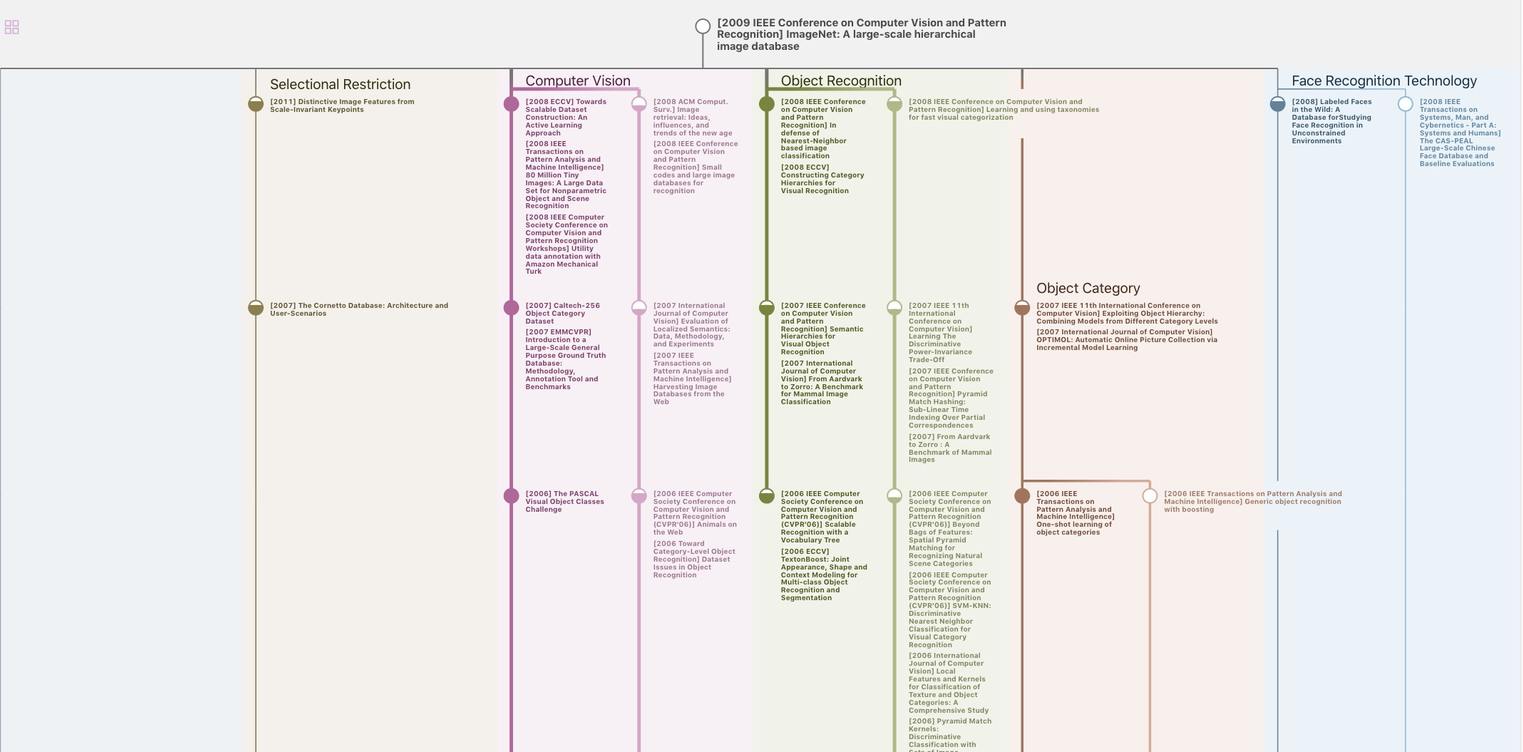
生成溯源树,研究论文发展脉络
Chat Paper
正在生成论文摘要