Thompson Sampling for Contextual Bandit Problems with Auxiliary Safety Constraints
CoRR(2019)
Abstract
Recent advances in contextual bandit optimization and reinforcement learning have garnered interest in applying these methods to real-world sequential decision making problems. Real-world applications frequently have constraints with respect to a currently deployed policy. Many of the existing constraint-aware algorithms consider problems with a single objective (the reward) and a constraint on the reward with respect to a baseline policy. However, many important applications involve multiple competing objectives and auxiliary constraints. In this paper, we propose a novel Thompson sampling algorithm for multi-outcome contextual bandit problems with auxiliary constraints. We empirically evaluate our algorithm on a synthetic problem. Lastly, we apply our method to a real world video transcoding problem and provide a practical way for navigating the trade-off between safety and performance using Bayesian optimization.
MoreTranslated text
AI Read Science
Must-Reading Tree
Example
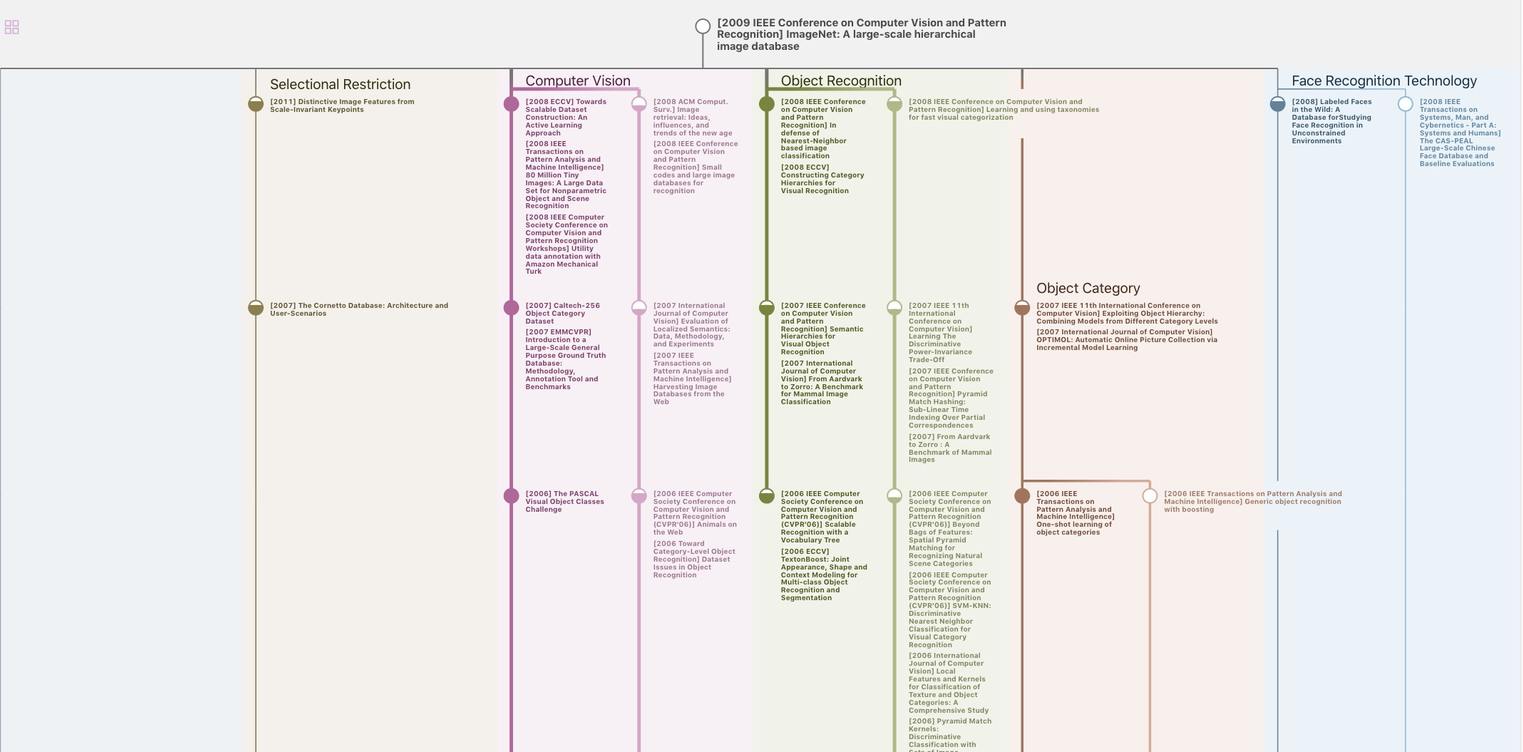
Generate MRT to find the research sequence of this paper
Chat Paper
Summary is being generated by the instructions you defined