Semi-Supervised Feature Selection Via Spectral Analysis
PROCEEDINGS OF THE SEVENTH SIAM INTERNATIONAL CONFERENCE ON DATA MINING(2007)
摘要
Feature selection is an important task in effective data mining. A new challenge to feature selection is the so-called "small labeled-sample problem" in which labeled data is small and unlabeled data is large. The paucity of labeled instances provides insufficient information about the structure of the target concept, and can cause supervised feature selection algorithms to fail. Unsupervised feature selection algorithms can work without labeled data. However, these algorithms ignore label information, which may lead to performance deterioration. In this work, we propose to use both (small) labeled and (large) unlabeled data in feature selection, which is a topic has not yet been addressed in feature selection research. We present a semi-supervised feature selection, algorithm based on spectral analysis. The algorithm exploits both labeled and unlabeled data through a regularization framework, which provides an effective way to address the "small labeled-sample" problem. Experimental results demonstrated the efficacy of our approach and confirmed that small labeled samples can help feature selection with unlabeled data.
更多查看译文
关键词
Feature Selection,Semi-supervised Learning,Machine Learning,Spectral Analysis
AI 理解论文
溯源树
样例
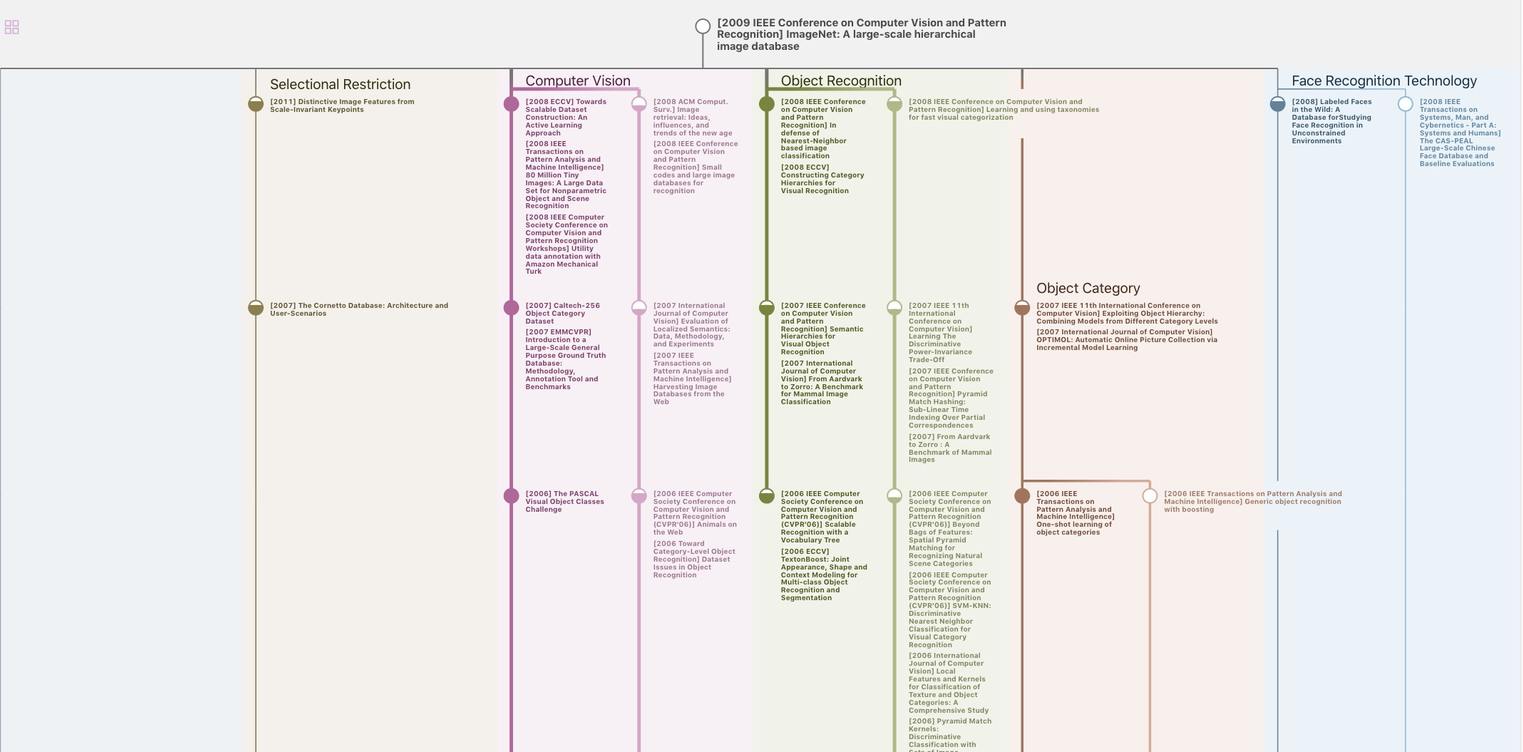
生成溯源树,研究论文发展脉络
Chat Paper
正在生成论文摘要