Fast optimization of PNN based on center neighbor and KLT
ICMLC(2010)
Abstract
Probabilistic Neural Networks (PNN) learn quickly from examples in one pass and asymptotically achieve the Bayes-optimal decision boundaries. The major disadvantage of PNN is that it requires one node or neuron for each training sample. Various clustering techniques have been proposed to reduce this requirement to one node per cluster center. A new fast optimization of PNN is investigated here using iteratively computing the centers of each class samples unrecognized and add their nearest neighbors to pattern layer. For fast constructing the classification model, weight and incremental technique is introduced to improve the learning speed. To further decrease the structure of PNN, KL transform is adopted to compress feature dimension. The approach proposed here decreases redundancy not only in samples using nearest neighbor but also in features using KL transformation. Experiments on UCI show the appropriate tradeoff in training time and generalization ability.
MoreTranslated text
Key words
optimisation,bayes methods,learning (artificial intelligence),karhunen-loeve transforms,pattern classification,feature dimension,sample selection,bayes optimal decision boundary,kl transform,fast optimization,probabilistic neural networks,probabilistic neural network,incremental learning,incremental technique,clustering technique,neural nets,cybernetics,accuracy,classification algorithms,clustering algorithms,learning artificial intelligence,machine learning,probabilistic logic,nearest neighbor
AI Read Science
Must-Reading Tree
Example
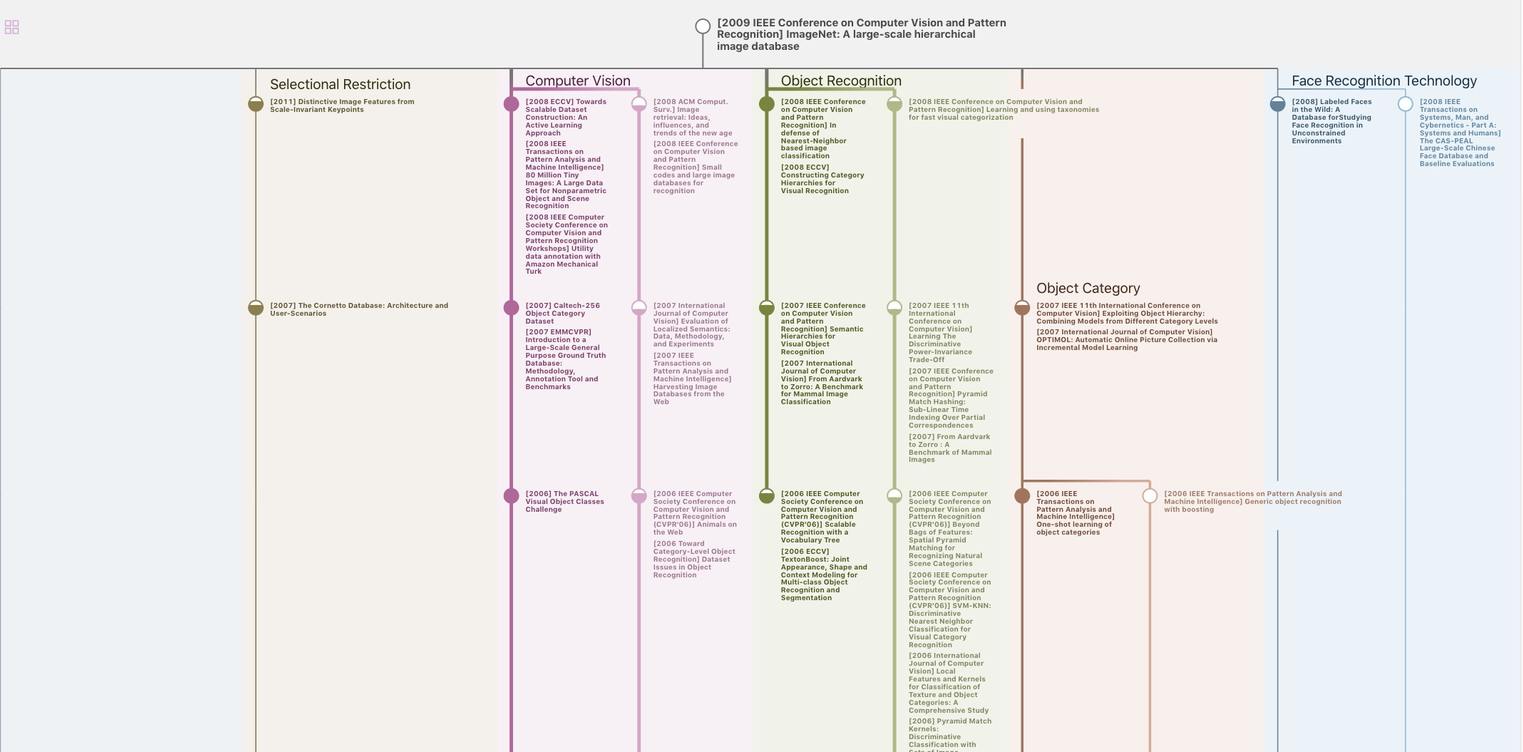
Generate MRT to find the research sequence of this paper
Chat Paper
Summary is being generated by the instructions you defined