WSN06-5: Distributed Bayesian Fault diagnosis in Collaborative Wireless Sensor Networks.
GLOBECOM 2006 - 2006 IEEE GLOBAL TELECOMMUNICATIONS CONFERENCE(2006)
摘要
In this contribution, we propose an efficient collaborative strategy for online change detection, in a distributed sensor network. The collaborative strategy ensures the efficiency and the robustness of the data processing, while limiting the required communication bandwidth. The observed systems are assumed to have each a finite set of states, including the abrupt change behavior. For each discrete state, an observed system is assumed to evolve according to a linear state-space model. An efficient Rao-Blackwellized collaborative particle filter (RB-CPF) is proposed to estimate the a posteriori probability of the discrete states of the observed systems. The Rao-Blackwellization procedure combines a sequential Monte Carlo filter with a bank of distributed Kalman filters. Only sufficient statistics are communicated between smart nodes. The spatio-temporal selection of the leader node and its collaborators is based on a trade-off between error propagation, communication constraints and information content complementarity of distributed data.
更多查看译文
关键词
Bayes methods,Kalman filters,Monte Carlo methods,fault diagnosis,maximum likelihood estimation,particle filtering (numerical methods),probability,spatiotemporal phenomena,state-space methods,wireless sensor networks,Rao-Blackwellized collaborative particle filter,collaborative wireless sensor networks,data processing,discrete state,distributed Bayesian fault diagnosis,distributed Kalman filters,error propagation,linear state-space model,maximum a posteriori probability,online change detection,sequential Monte Carlo filter,smart nodes,spatio-temporal selection,
AI 理解论文
溯源树
样例
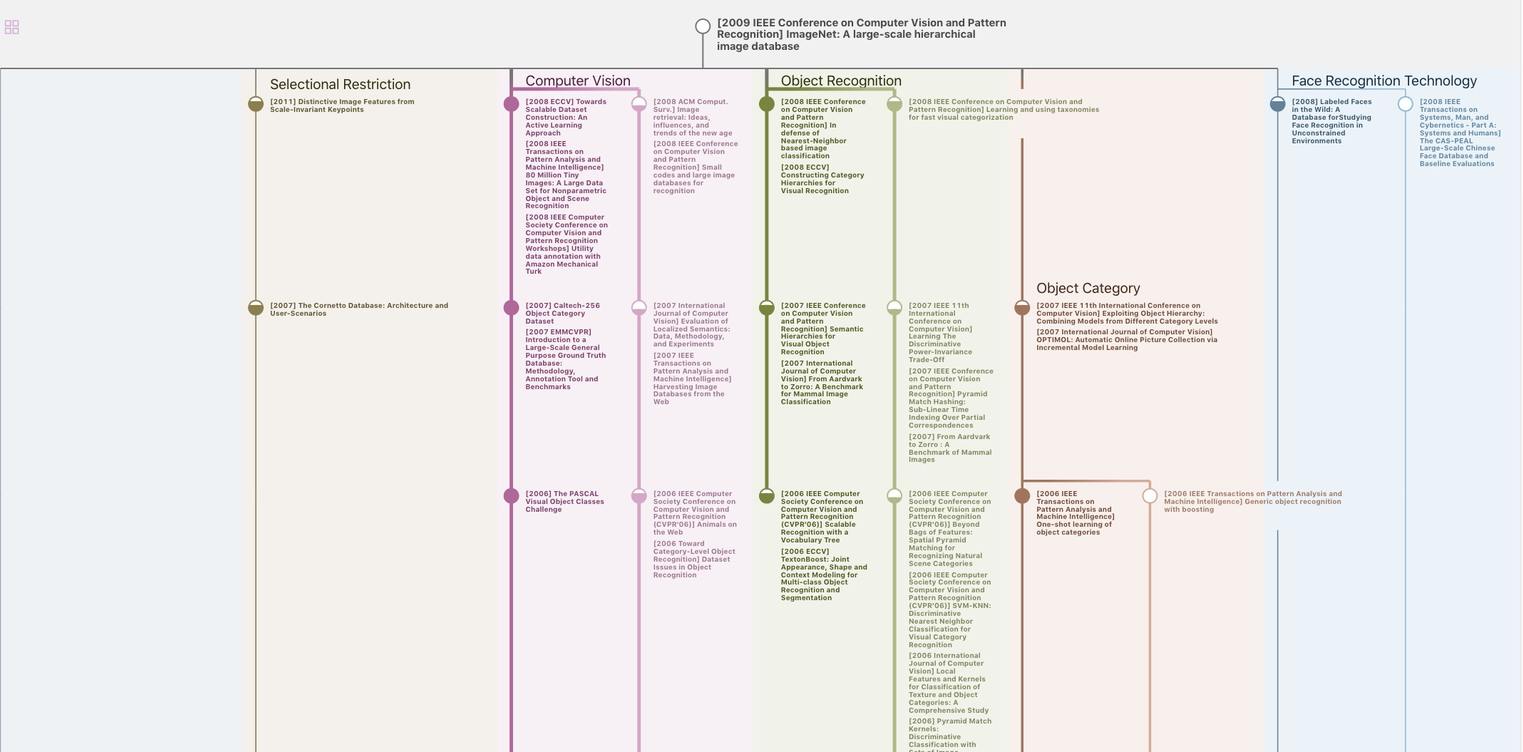
生成溯源树,研究论文发展脉络
Chat Paper
正在生成论文摘要