An improved YOLOv5-based vegetable disease detection method
Computers and Electronics in Agriculture(2022)
摘要
The vegetable is the most dynamic cash crop in the cultivation industry, and vegetable diseases are closely related to food security. Due to the characteristics of different diseases being similar and interference from the external environment, it makes difficult to detect vegetable diseases. Therefore, we propose an improved algorithm for vegetable disease detection based on YOLOv5s. The CSP, FPN and NMS modules in YOLOv5s are improved to eliminate the influence of the external environment, enhance the extraction capability of multi-scale features, and improve the detection range and detection performance. To verify the effectiveness and generalization of the model, we collected and labeled 1000 images of five diseases. Experiments show that the mAP of vegetable disease detection reached 93.1%, effectively reducing missed detection and wrong detection caused by the complex background and improving detection and localization effects on the small-scale disease. Compared with nanodet-plus, YOLOv4-tiny, YOLOv5s and YOLOX-s, the proposed algorithm has higher detection accuracy and localization accuracy than the other algorithms. The model size is 17.1 MB, and the average time to detect each image on the test platform is about 0.03 s, providing a new theoretical basis and research ideas for disease detection.
更多查看译文
关键词
Vegetable disease detection,Deep learning,Transformer encoder,Inception module,Intelligent agriculture
AI 理解论文
溯源树
样例
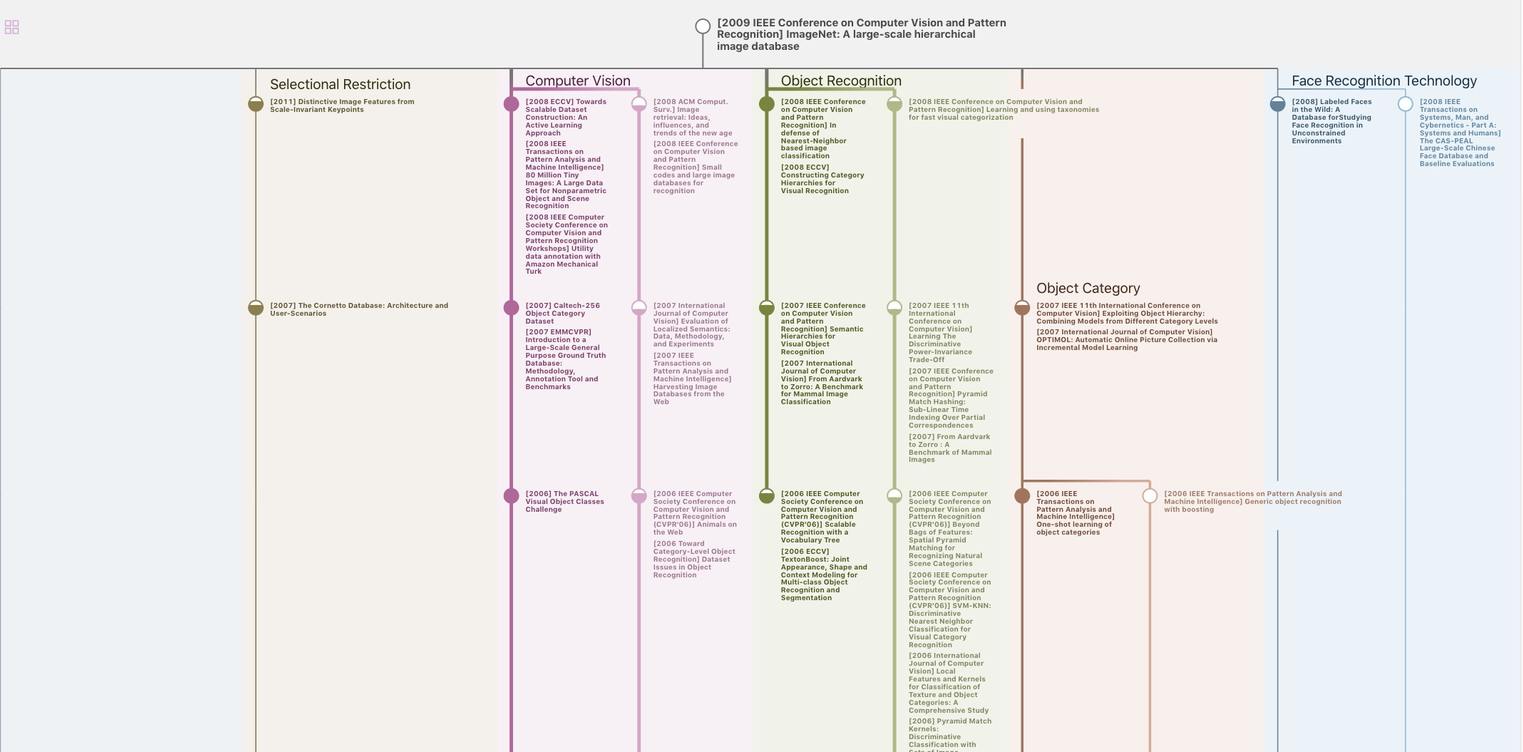
生成溯源树,研究论文发展脉络
Chat Paper
正在生成论文摘要