Decision Tree Based Information Integration for Automated Protein Classification
J. Bioinformatics and Computational Biology(2011)
摘要
We propose a novel technique for automatically generating the SCOP classification of a protein struc- ture with high accuracy. We achieve accurate classification by combining the decisions of multiple methods using the consensus of a committee (or an ensemble) classifier. Our technique, based on de- cision trees, is rooted in machine learning which shows that by judicially employing component clas- sifiers, an ensemble classifier can be constructed to outperform its components. We use two sequence- and three structure-comparison tools as component classifiers. Given a protein structure, using the joint hypothesis, we first determine if the protein belongs to an existing category (family, superfamily, fold) in the SCOP hierarchy. For the proteins that are predicted as members of the existing categories, we compute their family-, superfamily-, and fold-level classifications using the consensus classifier. We show that we can significantly improve the classification accuracy compared to the individual component classifiers. In particular, we achieve error rates that are 3-12 times less than the individual classifiers' error rates at the family level, 1.5-4.5 times less at the superfamily level, and 1.1-2.4 times less at the fold level.
更多查看译文
AI 理解论文
溯源树
样例
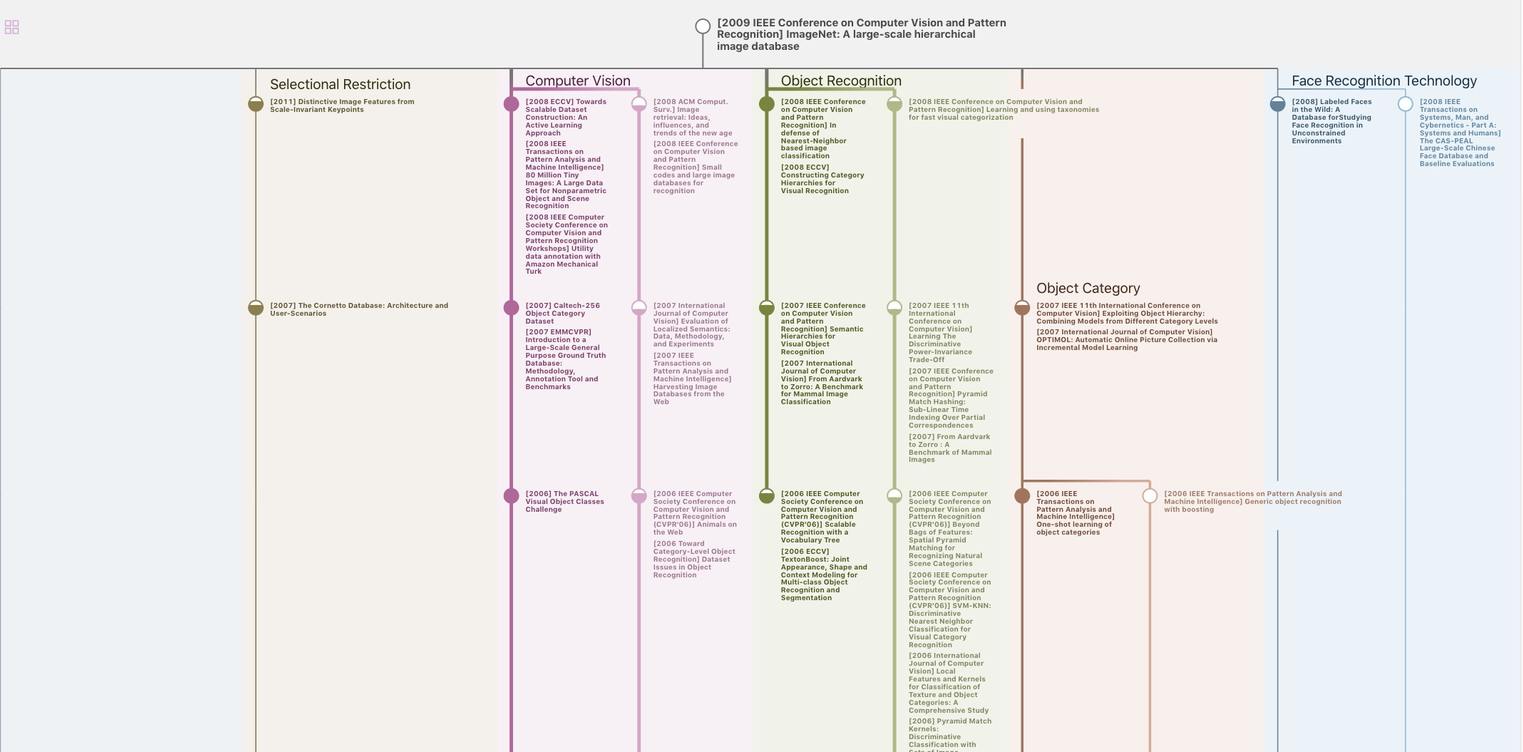
生成溯源树,研究论文发展脉络
Chat Paper
正在生成论文摘要