Artificial intelligence-powered pharmacovigilance: A review of machine and deep learning in clinical text-based adverse drug event detection for benchmark datasets
Journal of Biomedical Informatics(2024)
Abstract
Objective
The primary objective of this review is to investigate the effectiveness of machine learning and deep learning methodologies in the context of extracting adverse drug events (ADEs) from clinical benchmark datasets. We conduct an in-depth analysis, aiming to compare the merits and drawbacks of both machine learning and deep learning techniques, particularly within the framework of named-entity recognition (NER) and relation classification (RC) tasks related to ADE extraction. Additionally, our focus extends to the examination of specific features and their impact on the overall performance of these methodologies. In a broader perspective, our research extends to ADE extraction from various sources, including biomedical literature, social media data, and drug labels, removing the limitation to exclusively machine learning or deep learning methods.
Methods
We conducted an extensive literature review on PubMed using the query “(((machine learning [Medical Subject Headings (MeSH) Terms]) OR (deep learning [MeSH Terms])) AND (adverse drug event [MeSH Terms])) AND (extraction)”, and supplemented this with a snowballing approach to review 275 references sourced from retrieved articles.
Results
In our analysis, we included twelve articles for review. For the NER task, deep learning models outperformed machine learning models. In the RC task, gradient Boosting, multilayer perceptron and random forest models excelled. The Bidirectional Encoder Representations from Transformers (BERT) model consistently achieved the best performance in the end-to-end task. Future efforts in the end-to-end task should prioritize improving NER accuracy, especially for 'ADE' and 'Reason'.
Conclusion
These findings hold significant implications for advancing the field of ADE extraction and pharmacovigilance, ultimately contributing to improved drug safety monitoring and healthcare outcomes.
MoreTranslated text
Key words
Pharmacovigilance,Machine learning/Deep learning,Adverse drug event (ADE) extraction,named-entity recognition (NER),Relation extraction (RE),Natural language processing (NLP)
AI Read Science
Must-Reading Tree
Example
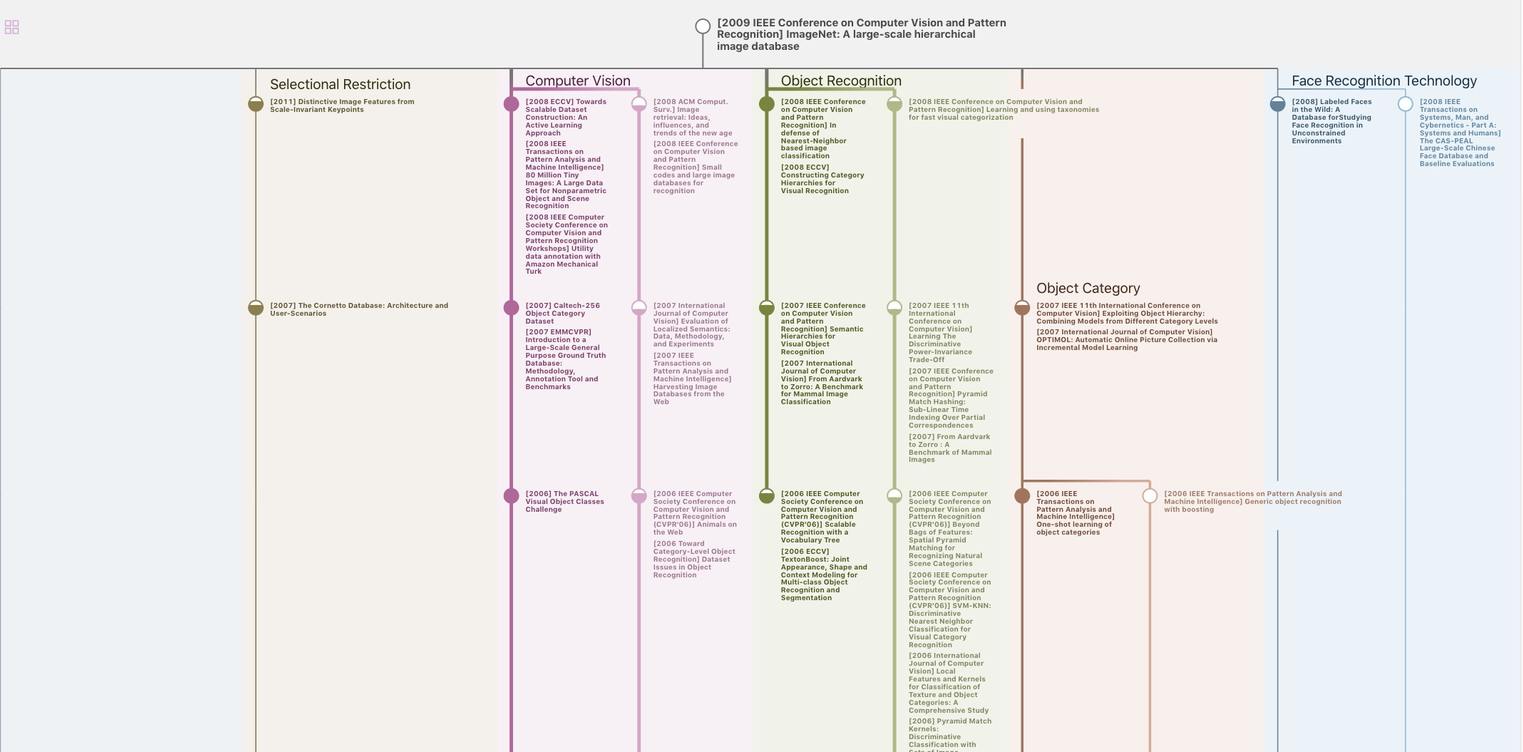
Generate MRT to find the research sequence of this paper
Chat Paper
Summary is being generated by the instructions you defined