Physical Simulation For Probabilistic Motion Tracking
CVPR(2008)
摘要
Human motion tracking is an important problem in computer vision. Most prior approaches have concentrated on efficient inference algorithms and prior motion models; however, few can explicitly account for physical plausibility of recovered motion. The primary purpose of this work is to enforce physical plausibility in the tracking of a single articulated human subject. Towards this end, we propose a full-body 3D physical simulation-based prior that explicitly incorporates motion control and dynamics into the Bayesian filtering framework. We consider the human's motion to be generated by a "control loop". In this control loop, Newtonian physics approximates the rigid-body motion dynamics of the human and the environment through the application and integration of forces. Collisions generate interaction forces to prevent physically impossible hypotheses. This allows us to properly model human motion dynamics, ground contact and environment interactions. For efficient inference in the resulting high-dimensional state space, we introduce exemplar-based control strategy to reduce the effective search space. As a result we are able to recover the physically-plausible kinematic and dynamic state of the body from monocular and multi-view imagery. We show, both quantitatively and qualitatively, that our approach performs favorably with respect to standard Bayesian filtering methods.
更多查看译文
关键词
filtering theory,image motion analysis,inference mechanisms,motion control,3D physical simulation,Bayesian filtering,Newtonian physics,computer vision,exemplar-based control strategy,human motion tracking,inference algorithms,motion control,physical plausibility,probabilistic motion tracking,rigid-body motion dynamics,search space,single articulated human subject,
AI 理解论文
溯源树
样例
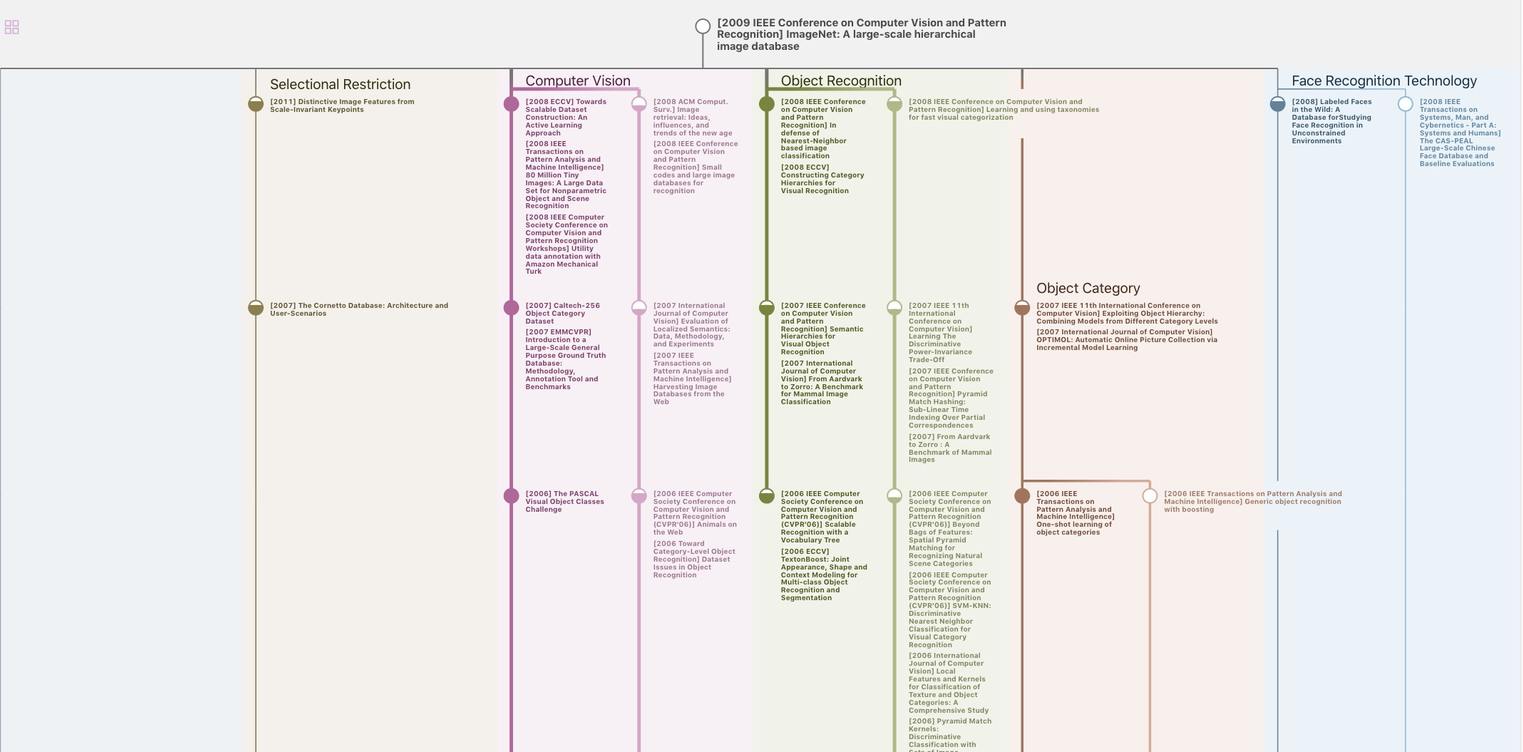
生成溯源树,研究论文发展脉络
Chat Paper
正在生成论文摘要