Face2Exp: Combating Data Biases for Facial Expression Recognition
IEEE Conference on Computer Vision and Pattern Recognition(2022)
摘要
Facial expression recognition (FER) is challenging due to the class imbalance caused by data collection. Existing studies tackle the data bias problem using only labeled facial expression dataset. Orthogonal to existing FER methods, we propose to utilize large unlabeled face recognition (FR) datasets to enhance FER. However, this raises another data bias problem—the distribution mismatch between FR and FER data. To combat the mismatch, we propose the Meta-Face2Exp framework, which consists of a base network and an adaptation network. The base network learns prior expression knowledge on class-balanced FER data while the adaptation network is trained to fit the pseudo labels of FR data generated by the base model. To combat the mismatch between FR and FER data, Meta-Face2Exp uses a circuit feedback mechanism, which improves the base network with the feedback from the adaptation network. Experiments show that our MetaFace2Exp achieves comparable accuracy to state-of-the-art FER methods with 10% of the labeled FER data utilized by the baselines. We also demonstrate that the circuit feedback mechanism successfully eliminates data bias
1
1
Code is available at link: https://github.com/danzeng1990/Face2Exp..
更多查看译文
关键词
Biometrics, Face and gestures
AI 理解论文
溯源树
样例
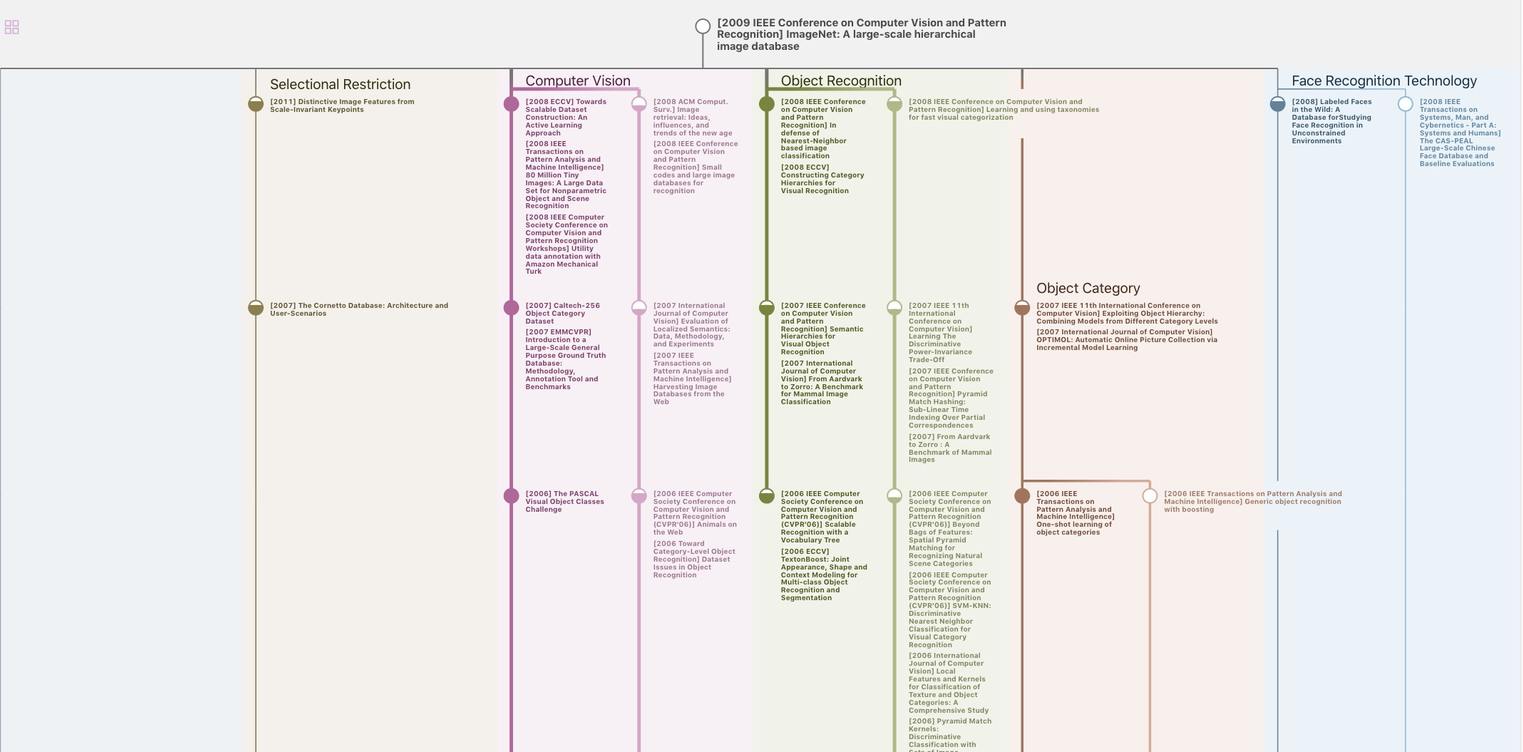
生成溯源树,研究论文发展脉络
Chat Paper
正在生成论文摘要