Algorithmic approaches to detecting interviewer fabrication in surveys
Algorithmic approaches to detecting interviewer fabrication in surveys(2012)
摘要
Surveys are one of the principal means of gathering critical data from low-income regions. Bad data, however, may be no better—or worse—than no data at all. Interviewer data fabrication, one cause of bad data, is an ongoing concern of survey organizations and a constant threat to data quality. In my dissertation work, I build software that automatically identifies interviewer fabrication so that supervisors can act to reduce it. To do so, I draw on two tool sets from computer science, one algorithmic and the other technological. On the algorithmic side, I use two sets of techniques from machine learning, supervised classification and anomaly detection, to automatically identify interviewer fabrication. On the technological side, I modify data collection software running on mobile electronic devices to record user traces that can help to identify fabrication. I show, based on the results of two empirical studies, that the combination of these approaches makes it possible to accurately and robustly identify interviewer fabrication, even when interviewers are aware that the algorithms are being used, have some knowledge of how they work, and are incentivized to avoid detection.
更多查看译文
关键词
anomaly detection,bad data,interviewer fabrication,algorithmic side,critical data,technological side,data quality,data collection software,dissertation work,interviewer data fabrication,machine learning,statistics,computer science
AI 理解论文
溯源树
样例
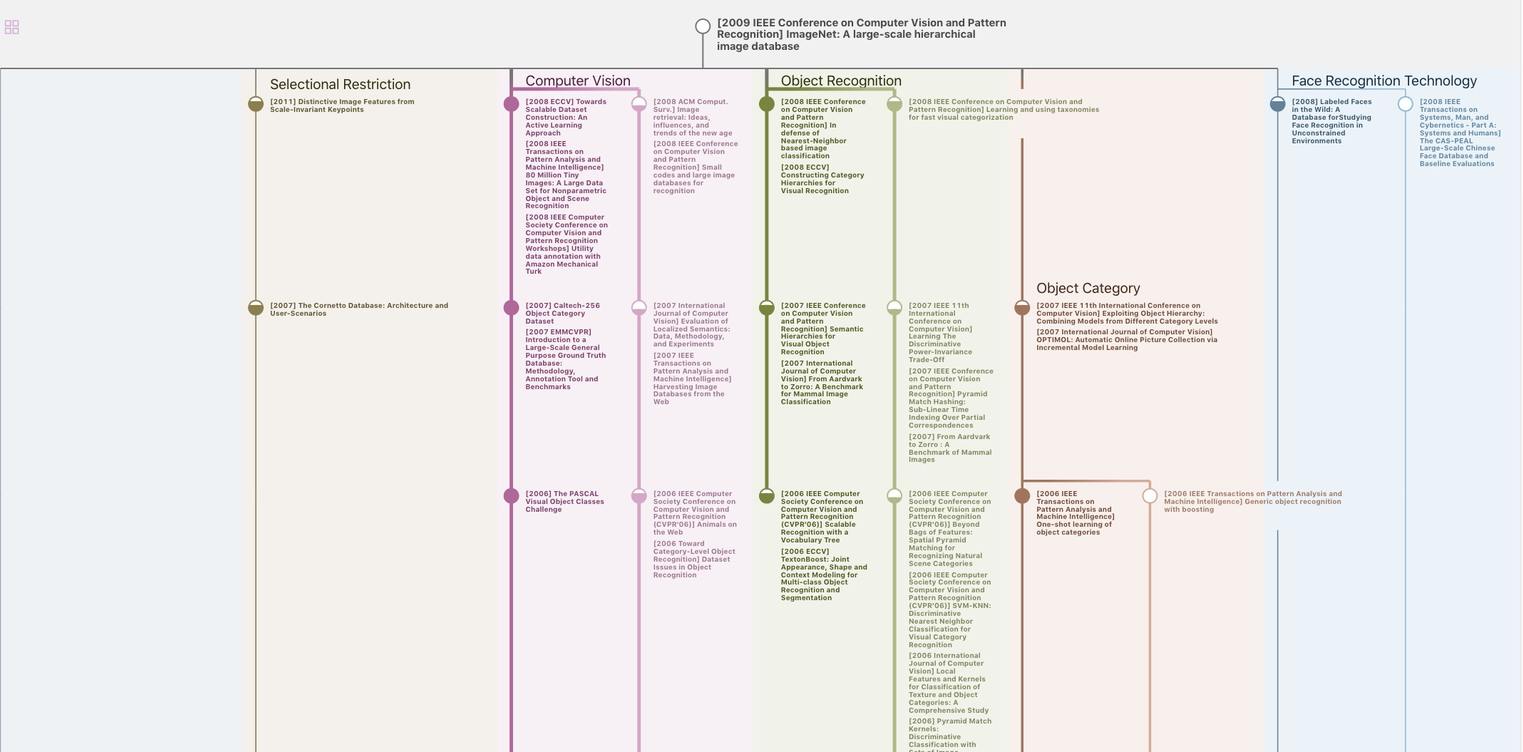
生成溯源树,研究论文发展脉络
Chat Paper
正在生成论文摘要