A modification of the ICOMP criterion for estimation of optimum complexity of PCR models
JOURNAL OF CHEMOMETRICS(2005)
摘要
The predictive ability of a PCR bilinear regression model is highly dependent on the number of latent variables selected. A non-optimal complexity is likely to result in a model yielding unsatisfactory predictions, due to a high bias or high variance of the coefficients of regression. The popular cross-validation methods such as leave-one-out cross-validation (LOOCV) and Monte-Carlo cross-validation (MCCV) are not always able to retain the proper number of latent variables, especially when atypical samples are present in the data. Also, they are computationally intensive, particularly for large data sets. In this study, the information complexity criterion ICOMP is modified in order to select the optimal PCR model. The results obtained demonstrate that this information criterion behaves at least as good as the cross-validation approaches, and usually outperforms them in terms of model selection and computation time, whether atypical samples are present in the data or not. Copyright (C) 2006 John Wiley & Sons, Ltd.
更多查看译文
关键词
complexity optimization,cross-validation,information criterion,atypical samples
AI 理解论文
溯源树
样例
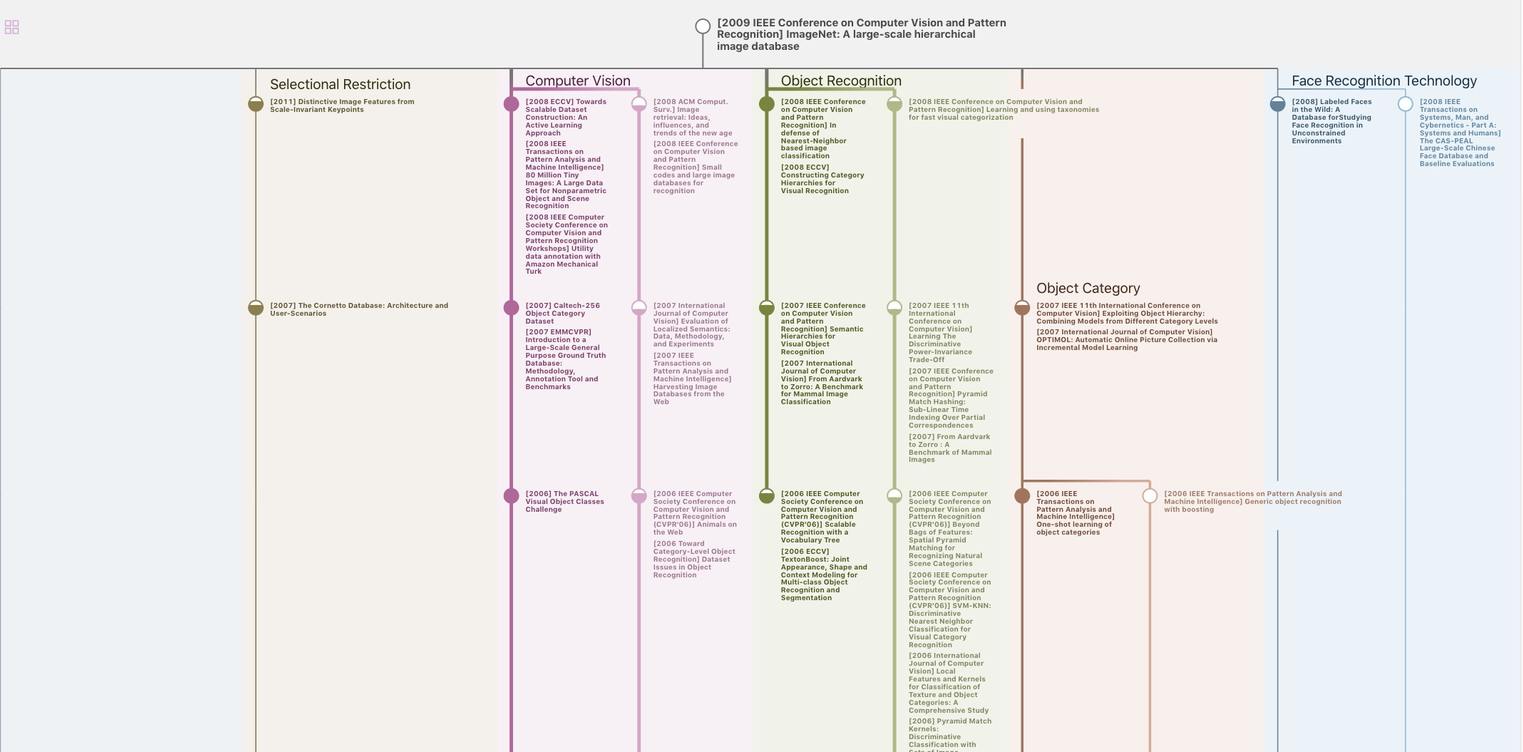
生成溯源树,研究论文发展脉络
Chat Paper
正在生成论文摘要