Improved EATFormer: A Vision Transformer for Medical Image Classification
CoRR(2024)
摘要
The accurate analysis of medical images is vital for diagnosing and
predicting medical conditions. Traditional approaches relying on radiologists
and clinicians suffer from inconsistencies and missed diagnoses. Computer-aided
diagnosis systems can assist in achieving early, accurate, and efficient
diagnoses. This paper presents an improved Evolutionary Algorithm-based
Transformer architecture for medical image classification using Vision
Transformers. The proposed EATFormer architecture combines the strengths of
Convolutional Neural Networks and Vision Transformers, leveraging their ability
to identify patterns in data and adapt to specific characteristics. The
architecture incorporates novel components, including the Enhanced EA-based
Transformer block with Feed-Forward Network, Global and Local Interaction , and
Multi-Scale Region Aggregation modules. It also introduces the Modulated
Deformable MSA module for dynamic modeling of irregular locations. The paper
discusses the Vision Transformer (ViT) model's key features, such as
patch-based processing, positional context incorporation, and Multi-Head
Attention mechanism. It introduces the Multi-Scale Region Aggregation module,
which aggregates information from different receptive fields to provide an
inductive bias. The Global and Local Interaction module enhances the MSA-based
global module by introducing a local path for extracting discriminative local
information. Experimental results on the Chest X-ray and Kvasir datasets
demonstrate that the proposed EATFormer significantly improves prediction speed
and accuracy compared to baseline models.
更多查看译文
AI 理解论文
溯源树
样例
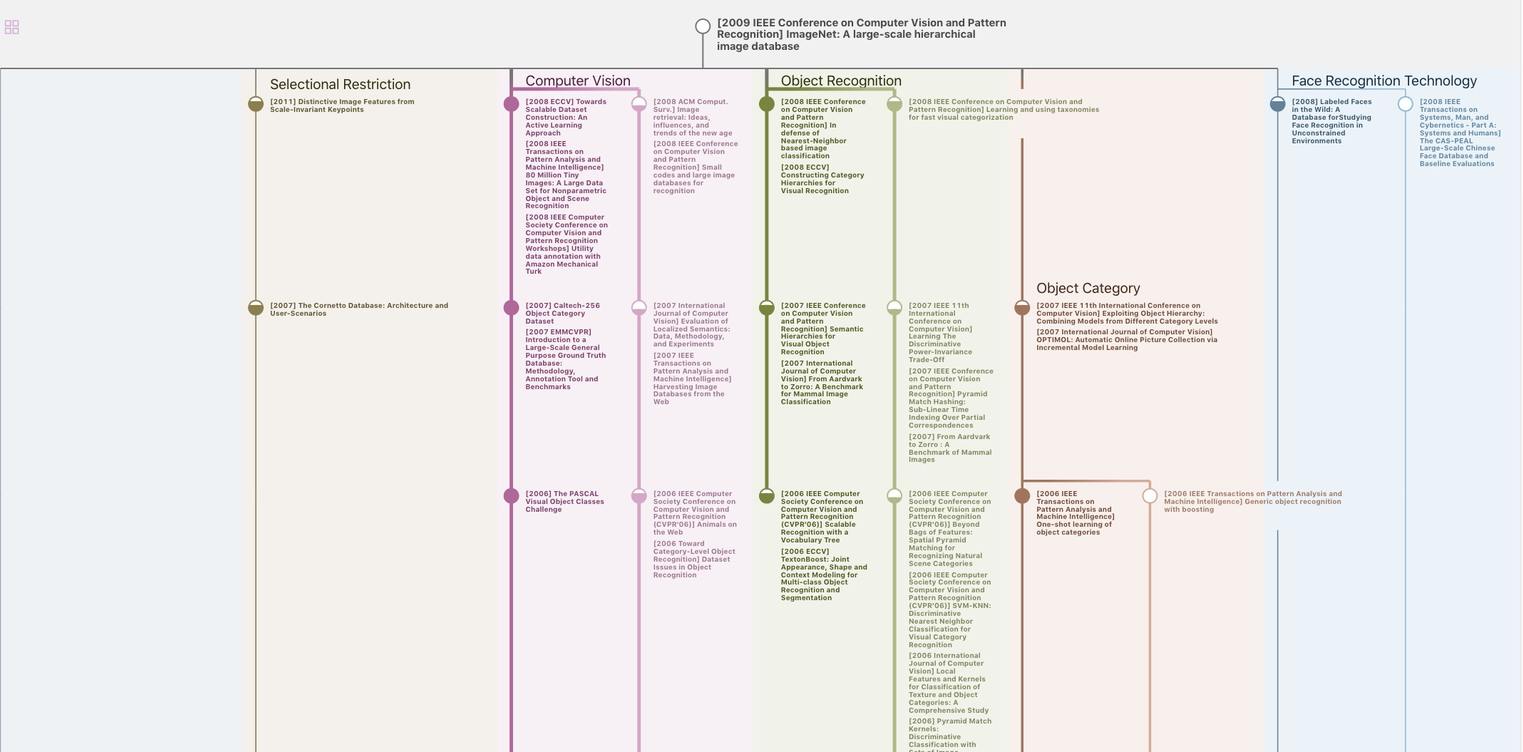
生成溯源树,研究论文发展脉络
Chat Paper
正在生成论文摘要