Multi-modal estimation with kernel embeddings for learning motion models.
ICRA(2013)
Abstract
We present a novel estimation algorithm for filtering and regression with a number of advantages over existing methods. The algorithm has wide application in robotics as no assumptions are made about the underlying distributions, it can represent non-Gaussian multi-modal posteriors, and learn arbitrary non-linear models from noisy data. Our method is a generalisation of the Kernel Bayes' Rule that produces multi-modal posterior estimates represented as Gaussian mixtures. The algorithm learns non-linear state transition and observation models from data and represents all distributions internally as elements in a reproducing kernel Hilbert space. Inference occurs in the Hilbert space and can be performed recursively. When an estimate of the posterior distribution is required, we apply a quadratic programming pre-image method to determine the Gaussian mixture components of the posterior representation.We demonstrate our algorithm with two filtering experiments and one regression experiment; a multi-modal tracking simulation, a real tracking problem involving a miniature slot-car with an attached inertial measurement unit, and a regression problem of estimating the velocity field of a set of pedestrian paths for robot path-planning. Our algorithm compares favourably with the Gaussian process in the regression case, and a particle filter with learned process and observation models (the "GP-BayesFilter" particle filter).
MoreTranslated text
Key words
Bayes methods,Gaussian processes,estimation theory,filtering theory,mobile robots,path planning,regression analysis,robot vision,tracking,GP-BayesFilter particle filter,Gaussian mixture components,Gaussian mixtures,Gaussian process,arbitrary nonlinear models,filtering experiments,inertial measurement unit,kernel Bayes rule generalisation,kernel Hilbert space,kernel embeddings,miniature slot-car,motion models,multimodal estimation,multimodal posterior estimation,multimodal tracking simulation,nonGaussian multimodal posteriors,nonlinear state transition,observation models,pedestrian paths,posterior distribution estimation,posterior representation,quadratic programming preimage method,regression experiment,regression problem,robot path-planning,velocity field estimation
AI Read Science
Must-Reading Tree
Example
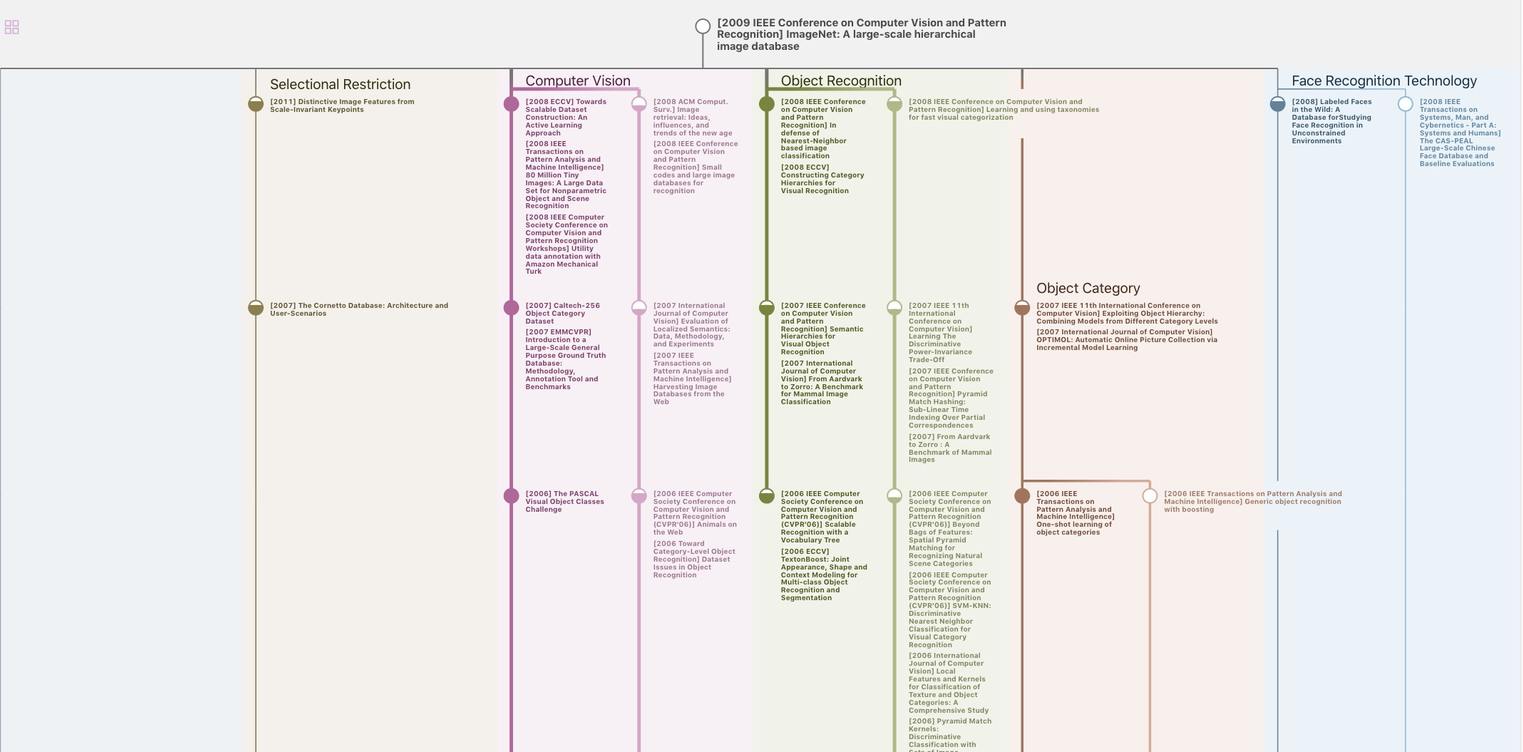
Generate MRT to find the research sequence of this paper
Chat Paper
Summary is being generated by the instructions you defined