Online Missing Value Imputation and Correlation Change Detection for Mixed-type Data via Gaussian Copula
AAAI Conference on Artificial Intelligence(2020)
Abstract
Most data science algorithms require complete observations, yet many datasets contain missing values. Hence missing value imputation is crucial for real-world data science workflows. For practical applications, imputation algorithms should produce imputations that match the true data distribution, handle mixed data containing ordinal, boolean, and continuous variables, and scale to large datasets. In this work we develop a new online imputation algorithm for mixed data using the Gaussian copula. The online Gaussian copula model produces meets all the desiderata: its imputations match the data distribution even for mixed data, and it scales well, achieving up to an order of magnitude speedup over its offline counterpart. The online algorithm can handle streaming or sequential data and can adapt to a changing data distribution. By fitting the copula model to online data, we also provide a new method to detect a change in the correlational structure of multivariate mixed data with missing values. Experimental results on synthetic and real world data validate the performance of the proposed methods.
MoreTranslated text
Key words
gaussian copula,correlation change detection,change detection,mixed-type
AI Read Science
Must-Reading Tree
Example
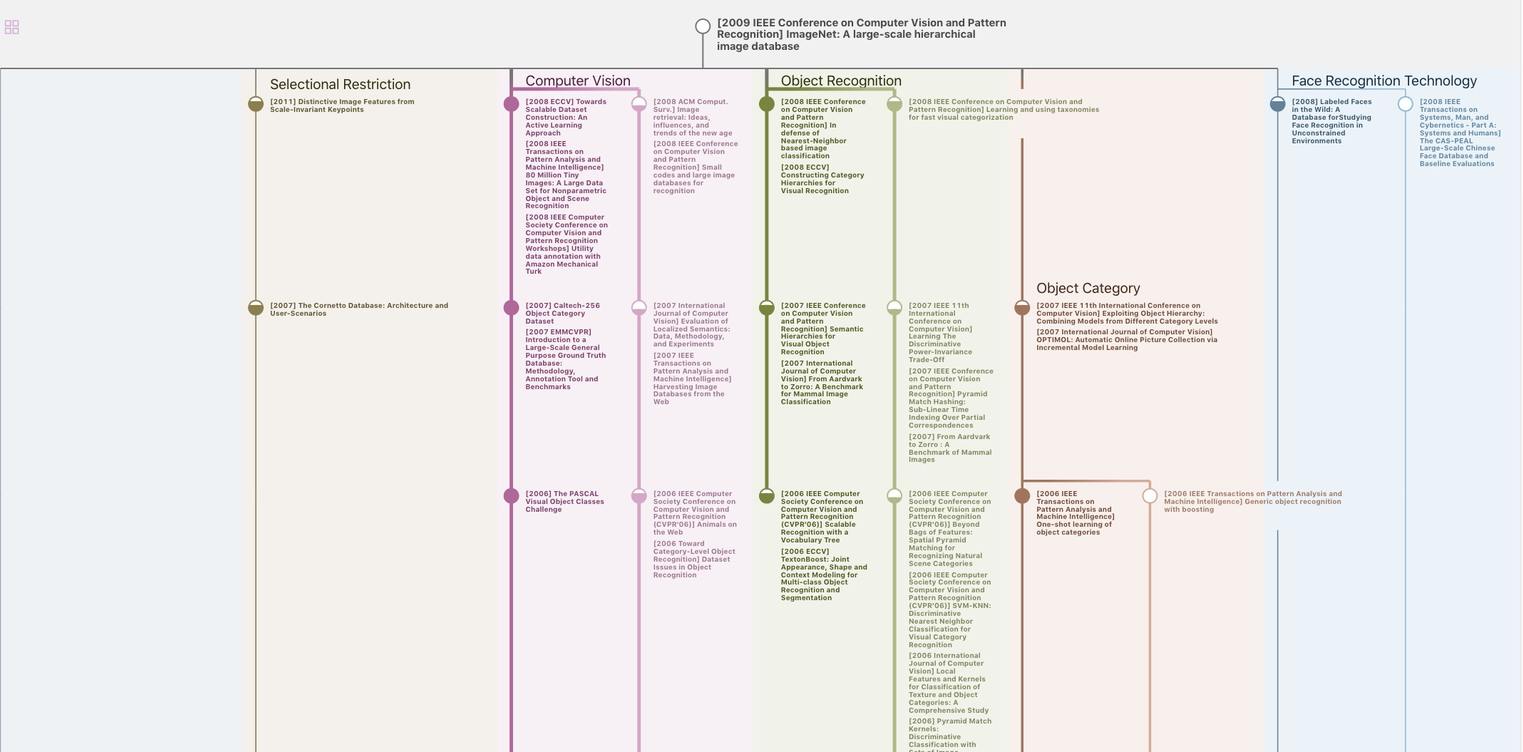
Generate MRT to find the research sequence of this paper
Chat Paper
Summary is being generated by the instructions you defined