7.1 A 3.4-to-13.3TOPS/W 3.6TOPS Dual-Core Deep-Learning Accelerator for Versatile AI Applications in 7nm 5G Smartphone SoC
2020 IEEE International Solid- State Circuits Conference - (ISSCC)(2020)
Abstract
Recent advancements in deep learning (DL) have led to the wide adoption of AI applications, such as image recognition [1], image de-noising and speech recognition, in the 5G smartphones. For a satisfactory user experience, there are stringent requirements in the real-time response of smartphone applications. In order to meet the performance expectations for DL, numerous deep learning accelerators (DLA) have been proposed for DL inference on the edge devices [2]–[5]. As depicted in Fig. 7.1.1, the major challenge in designing a DLA for smartphones is achieving the required computing efficiency, while limited by the power budget and memory bandwidth (BW). Since the overall power consumption of a smartphone system-on-a-chip (SoC) is usually constrained to 2 to 3W and the available DRAM BW is around 10-to-30GB/s, the power budget allocated for a DLA must be below 1W with the memory BW limited to 1-to-10GB/s. While operating under such constraints, the DLA is required to support various network topologies and highly precise neural operations in smartphone applications. For instance, the Android neural network APIs currently specify the use of asymmetric quantization (ASYMM-Q), providing better precision than conventional symmetric quantization.
MoreTranslated text
Key words
5G smartphone SoC,smartphone system-on-a-chip,power budget,required computing efficiency,smartphones,DLA,numerous deep learning accelerators,smartphone applications,satisfactory user experience,versatile AI applications,power 3.0 W,power 1.0 W,size 7.0 nm,byte rate 30.0 GByte/s,byte rate 10.0 GByte/s
AI Read Science
Must-Reading Tree
Example
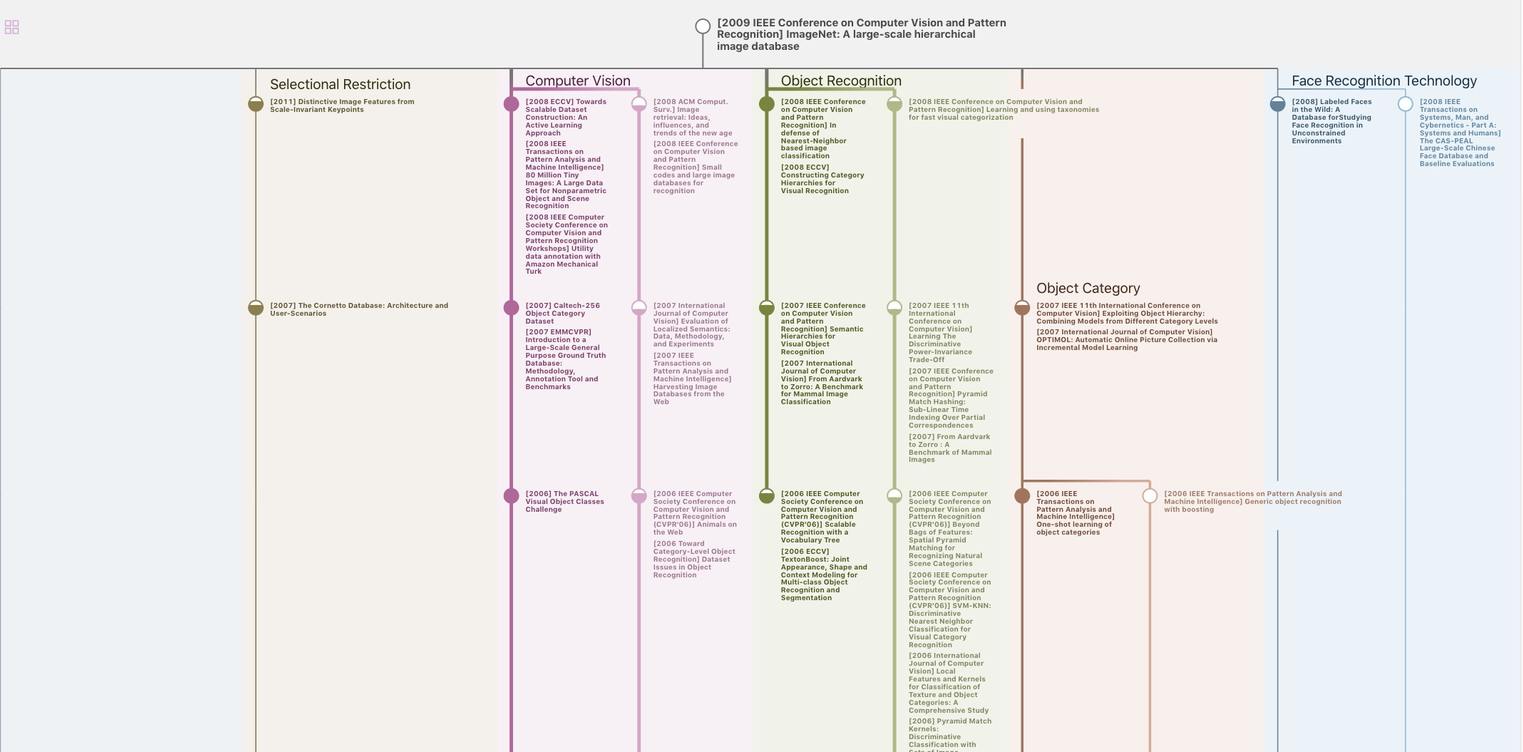
Generate MRT to find the research sequence of this paper
Chat Paper
Summary is being generated by the instructions you defined