Transfer Learning across Networks for Collective Classification
Data Mining(2014)
摘要
This paper addresses the problem of transferring useful knowledge from a source network to predict node labels in a newly formed target network. While existing transfer learning research has primarily focused on vector-based data, in which the instances are assumed to be independent and identically distributed, how to effectively transfer knowledge across different information networks has not been well studied, mainly because networks may have their distinct node features and link relationships between nodes. In this paper, we propose a new transfer learning algorithm that attempts to transfer common latent structure features across the source and target networks. The proposed algorithm discovers these latent features by constructing label propagation matrices in the source and target networks, and mapping them into a shared latent feature space. The latent features capture common structure patterns shared by two networks, and serve as domain-independent features to be transferred between networks. Together with domain-dependent node features, we thereafter propose an iterative classification algorithm that leverages label correlations to predict node labels in the target network. Experiments on real-world networks demonstrate that our proposed algorithm can successfully achieve knowledge transfer between networks to help improve the accuracy of classifying nodes in the target network.
更多查看译文
关键词
real-world networks,iterative classification algorithm,source network,label propagation matrices,learning (artificial intelligence),node label prediction,pattern classification,collective classification,vector-based data,node features,network,domain-dependent node features,knowledge transfer,transfer learning algorithm,link relationships,label correlations,node classification accuracy improvement,target network,information networks,transfer learning,shared latent feature space,iterative methods,network theory (graphs),common latent structure feature transfer,learning artificial intelligence
AI 理解论文
溯源树
样例
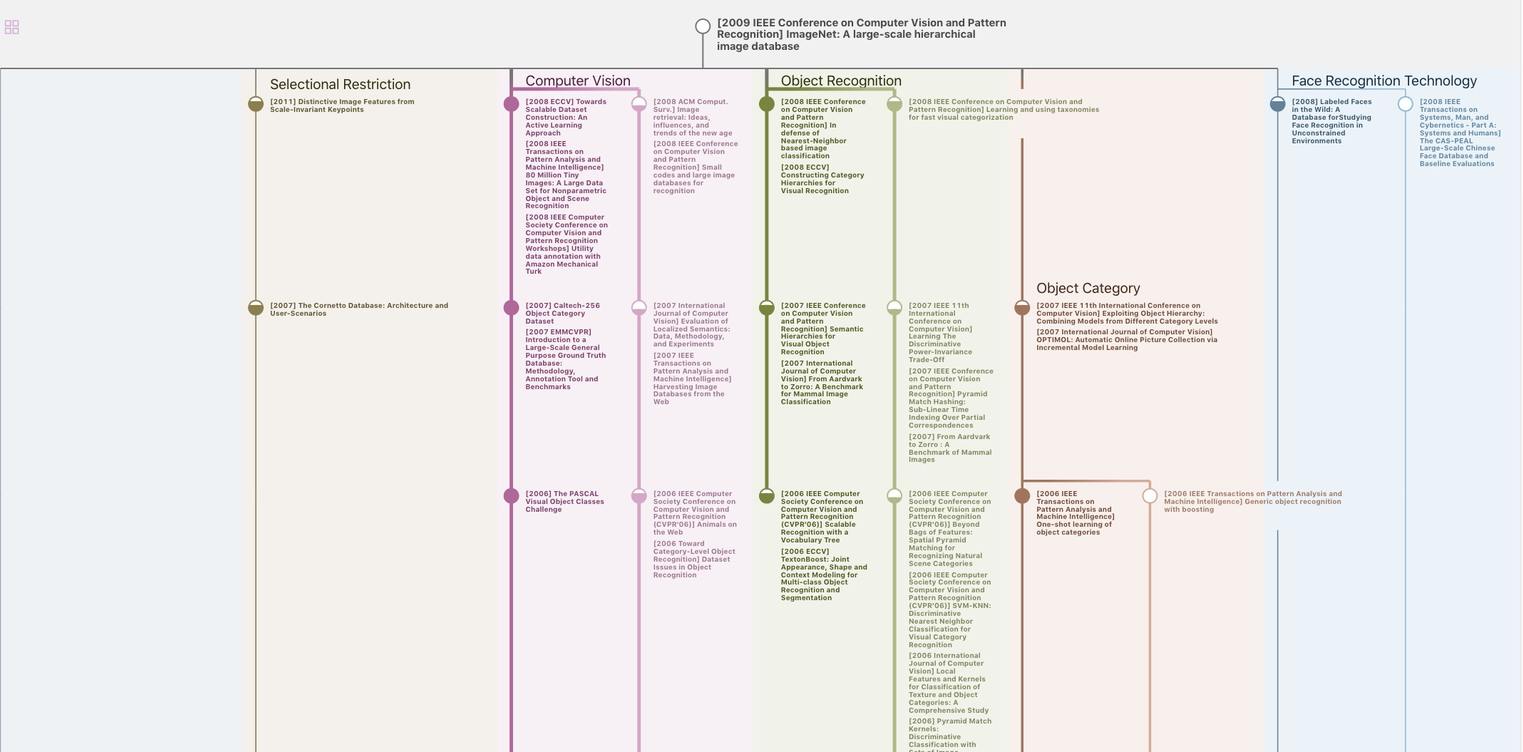
生成溯源树,研究论文发展脉络
Chat Paper
正在生成论文摘要