代价敏感Boosting算法研究
Journal of Nanjing University of Science and Technology(2013)
摘要
针对代价敏感学习问题,研究boosting算法的代价敏感扩展。提出一种基于代价敏感采样的代价敏感boosting学习方法,通过在原始boosting每轮迭代中引入代价敏感采样,最小化代价敏感损失期望。基于上述学习框架,推导出两种代价敏感boosting算法,同时,揭示并解释已有算法的不稳定本质。在加州大学欧文分校(University of California,Irvine,UCI)数据集和麻省理工学院生物和计算学习中心(Center for Biological&Computational Learning,CBCL)人脸数据集上的实验结果表明,对于代价敏感分类问题,代价敏感采样boosting算法优于原始boosting和已有代价敏感boosting算法。
更多查看译文
关键词
cost-sensitive learning,cost-sensitive boosting,cost-sensitive sampling,boosting
AI 理解论文
溯源树
样例
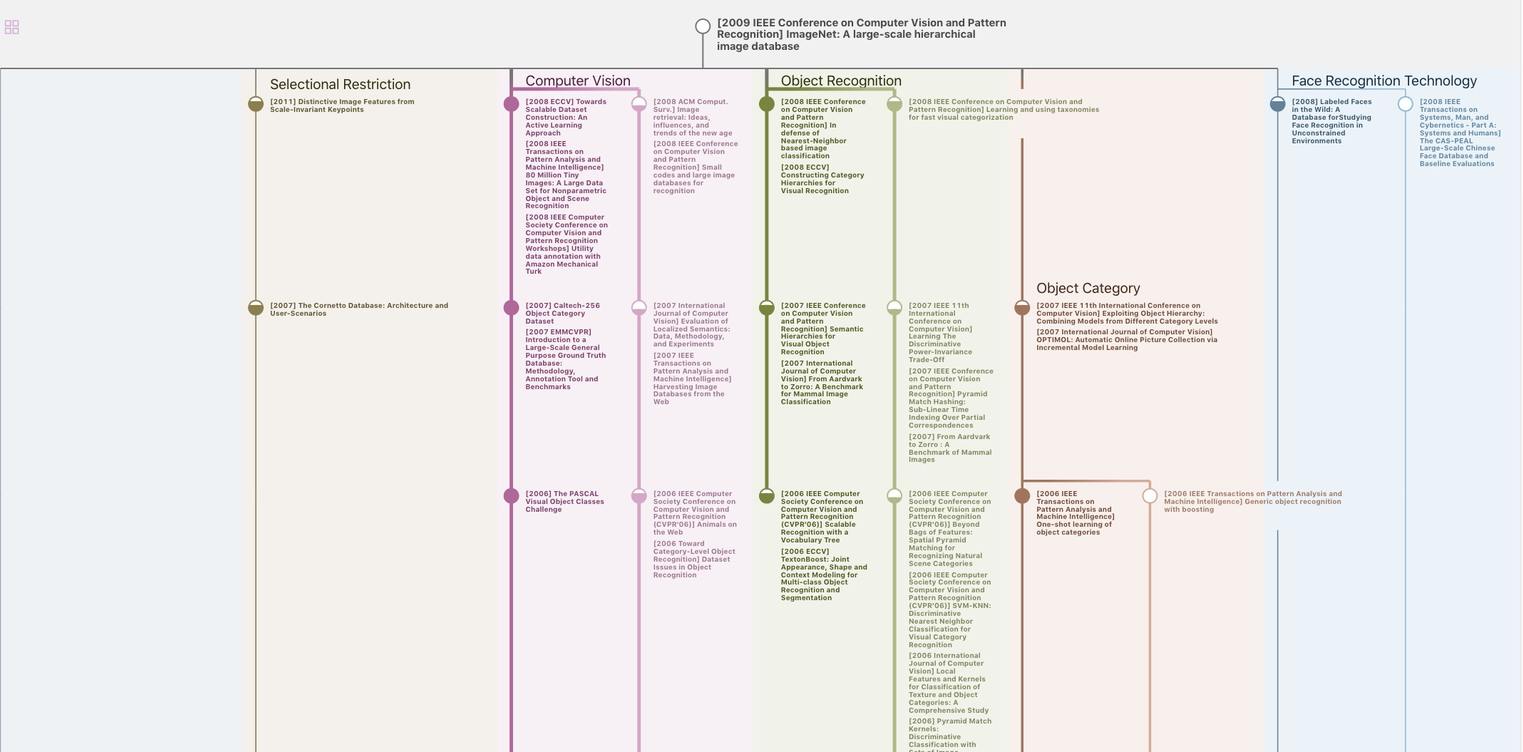
生成溯源树,研究论文发展脉络
Chat Paper
正在生成论文摘要