Towards an optimal stochastic alternating direction method of multipliers.
ICML'14: Proceedings of the 31st International Conference on International Conference on Machine Learning - Volume 32(2014)
摘要
We study regularized stochastic convex optimization subject to linear equality constraints. This class of problems was recently also studied by Ouyang et al. (2013) and Suzuki (2013); both introduced similar stochastic alternating direction method of multipliers (SADMM) algorithms. However, the analysis of both papers led to suboptimal convergence rates. This paper presents two new SADMM methods: (i) the first attains the minimax optimal rate of O (1/ k ) for nonsmooth strongly-convex stochastic problems; while (ii) the second progresses towards an optimal rate by exhibiting an O (1/ k 2 ) rate for the smooth part. We present several experiments with our new methods; the results indicate improved performance over competing ADMM methods.
更多查看译文
AI 理解论文
溯源树
样例
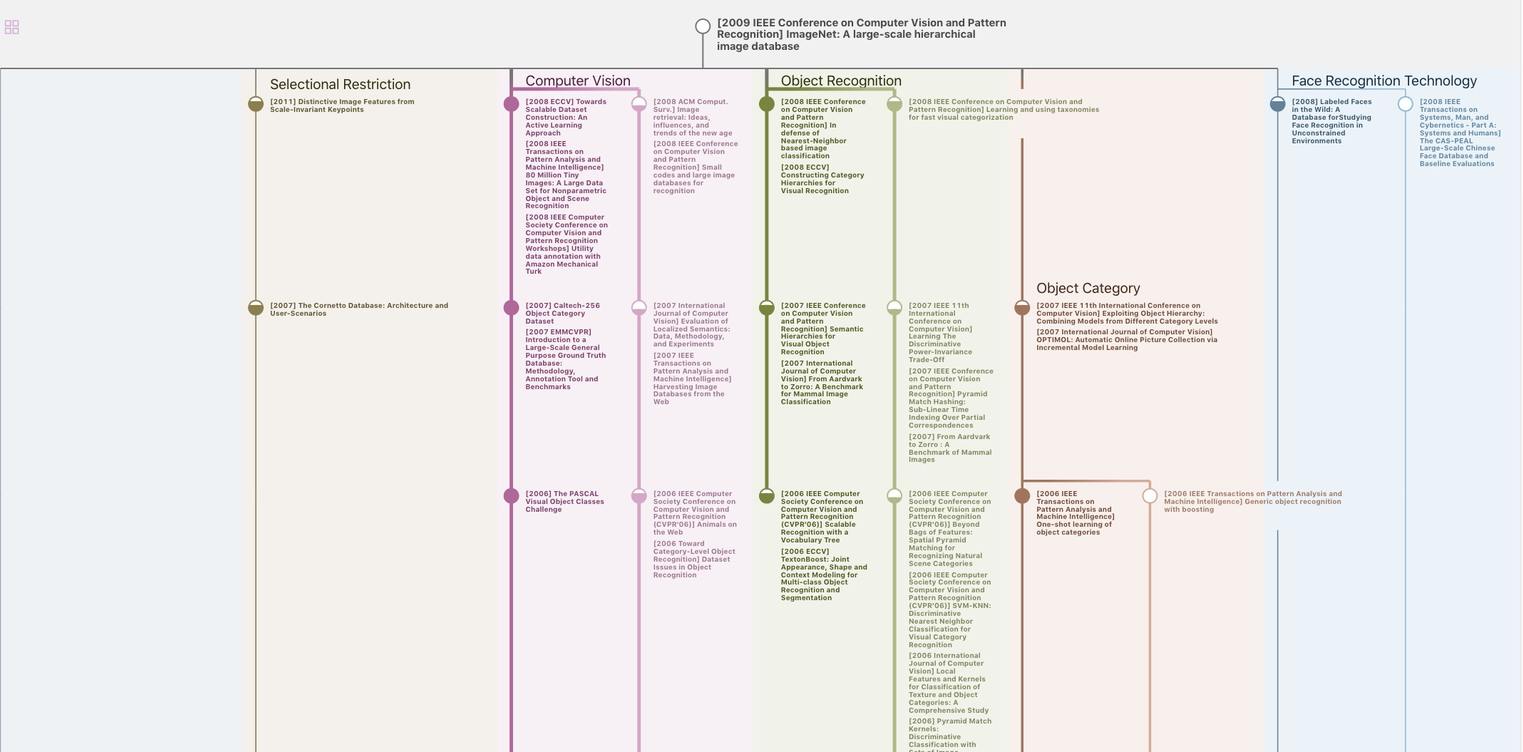
生成溯源树,研究论文发展脉络
Chat Paper
正在生成论文摘要