Weights optimization for multi-instance multi-label RBF neural networks using steepest descent method
Neural Computing and Applications(2012)
摘要
Multi-instance multi-label learning (MIML) is an innovative learning framework where each sample is represented by multiple instances and associated with multiple class labels. In several learning situations, the multi-instance multi-label RBF neural networks (MIMLRBF) can exploit connections between the instances and the labels of an MIML example directly, while most of other algorithms cannot learn that directly. However, the singular value decomposition (SVD) method used to compute the weights of the output layer will cause augmented overall error in network performance when training data are noisy or not easily discernible. This paper presents an improved approach to learning algorithms used for training MIMLRBF. The steepest descent (SD) method is used to optimize the weights after they are initialized by the SVD method. Comparing results employing diverse learning strategies shows interesting outcomes as have come out of this paper.
更多查看译文
关键词
Machine learning,Neural networks,Radial basis function,Multi-instance multi-label learning
AI 理解论文
溯源树
样例
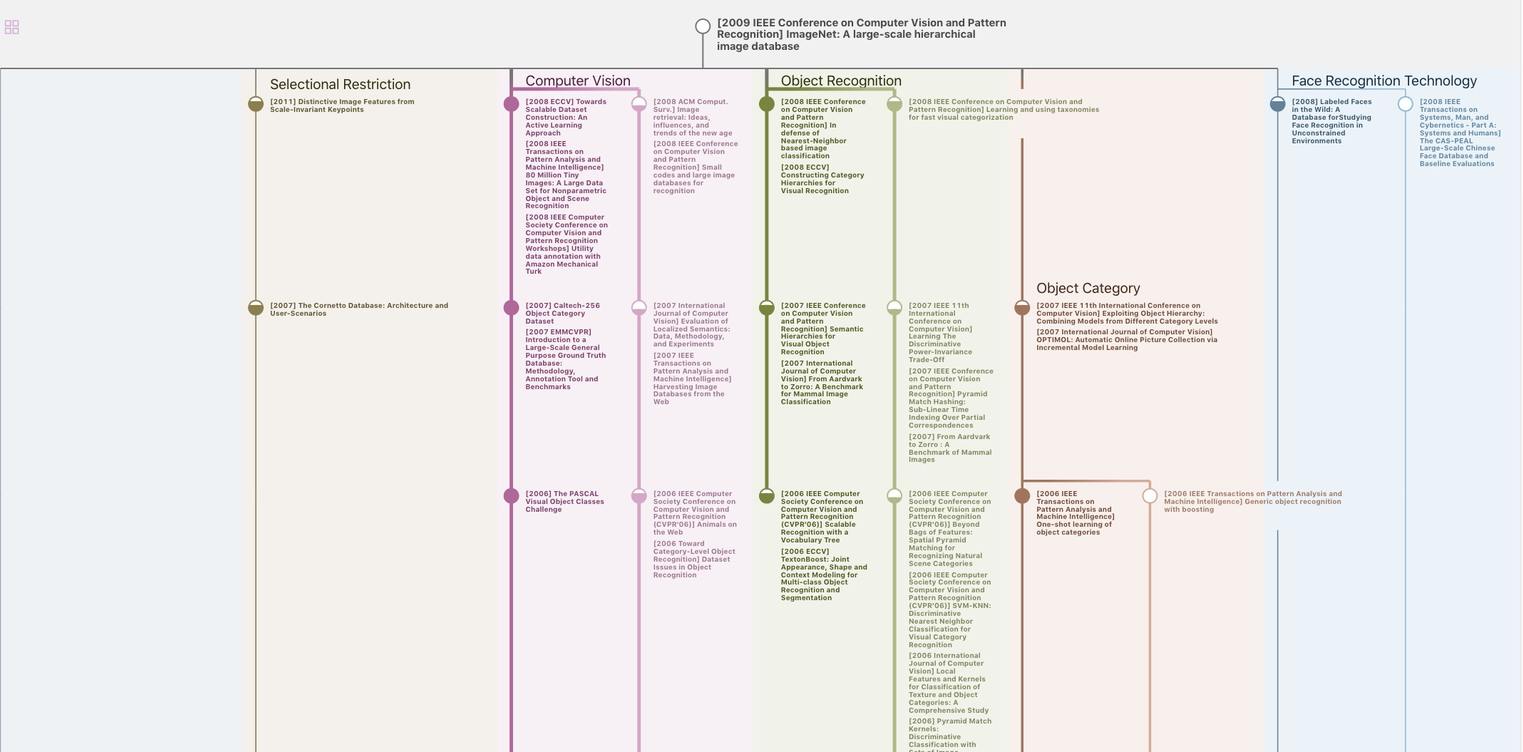
生成溯源树,研究论文发展脉络
Chat Paper
正在生成论文摘要