Semi-Supervised Learning From Only Positive And Unlabeled Data Using Entropy
WAIM'10: Proceedings of the 11th international conference on Web-age information management(2010)
摘要
The problem of classification from positive and unlabeled examples attracts much attention currently. However, when the number of unlabeled negative examples is very small, the effectiveness of former work has been decreased. This paper propose an effective approach to address this problem, and we firstly use entropy to selects the likely positive and negative examples to build a complete training set; and then logistic regression classifier is applied on this new training set for classification. A series of experiments are conducted. The experimental results illustrate that the proposed approach outperforms previous work in the literature.
更多查看译文
关键词
Unlabeled Data, Negative Class, Positive Class, Negative Instance, Ridge Estimator
AI 理解论文
溯源树
样例
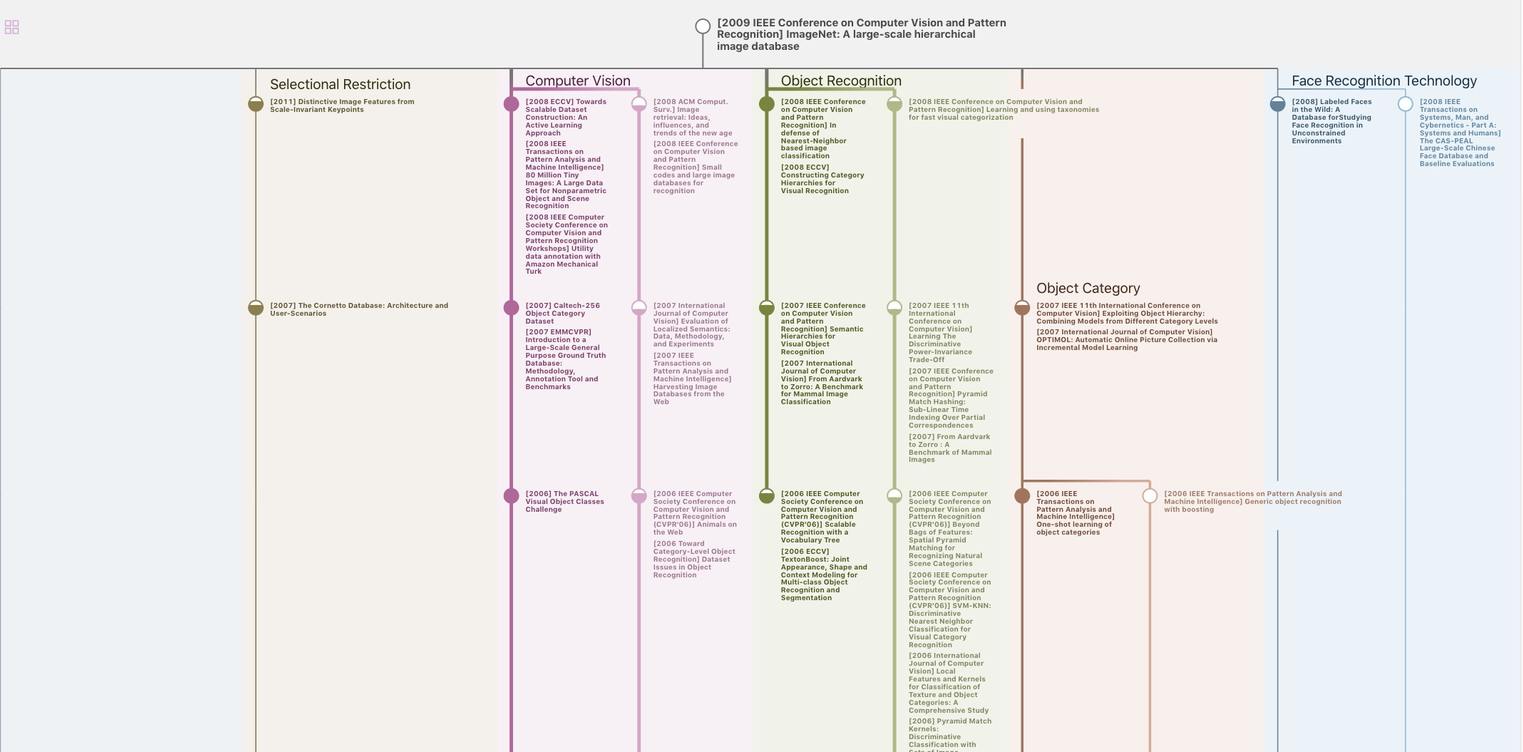
生成溯源树,研究论文发展脉络
Chat Paper
正在生成论文摘要