THE EFFECT OF NOISY BOOTSTRAPPING ON THE ROBUSTNESS OF SUPERVISED CLASSIFICATION OF GENE EXPRESSION DATA
Sao Luis(2004)
摘要
This paper discusses the role of noisy bootstrapping in the analysis of microarray data. We apply linear discriminant anal- ysis, according to Fisher's method, to perform feature selection and classification, creating a linear model which enables clinicians easier interpretation of the results. We present the eects of bootstrap- ping in improvement of the results, and specifically robustifying classification with an increased number of genes. The performance of our method is demonstrated on publicly available datasets, and a comparison with state of the art pub- lished results is included. In particular, we show the eect of the number of features (genes) on the result, as well as the eect of bootstrapping. The results show that our classifier is accurate and quite competitive to other classifiers, although it is simpler, and enables considering a larger set of genes in the classification.
更多查看译文
关键词
arrays,data analysis,genetics,feature selection,gene expression data,linear discriminant analysis,microarray data,noisy bootstrapping,supervised classification
AI 理解论文
溯源树
样例
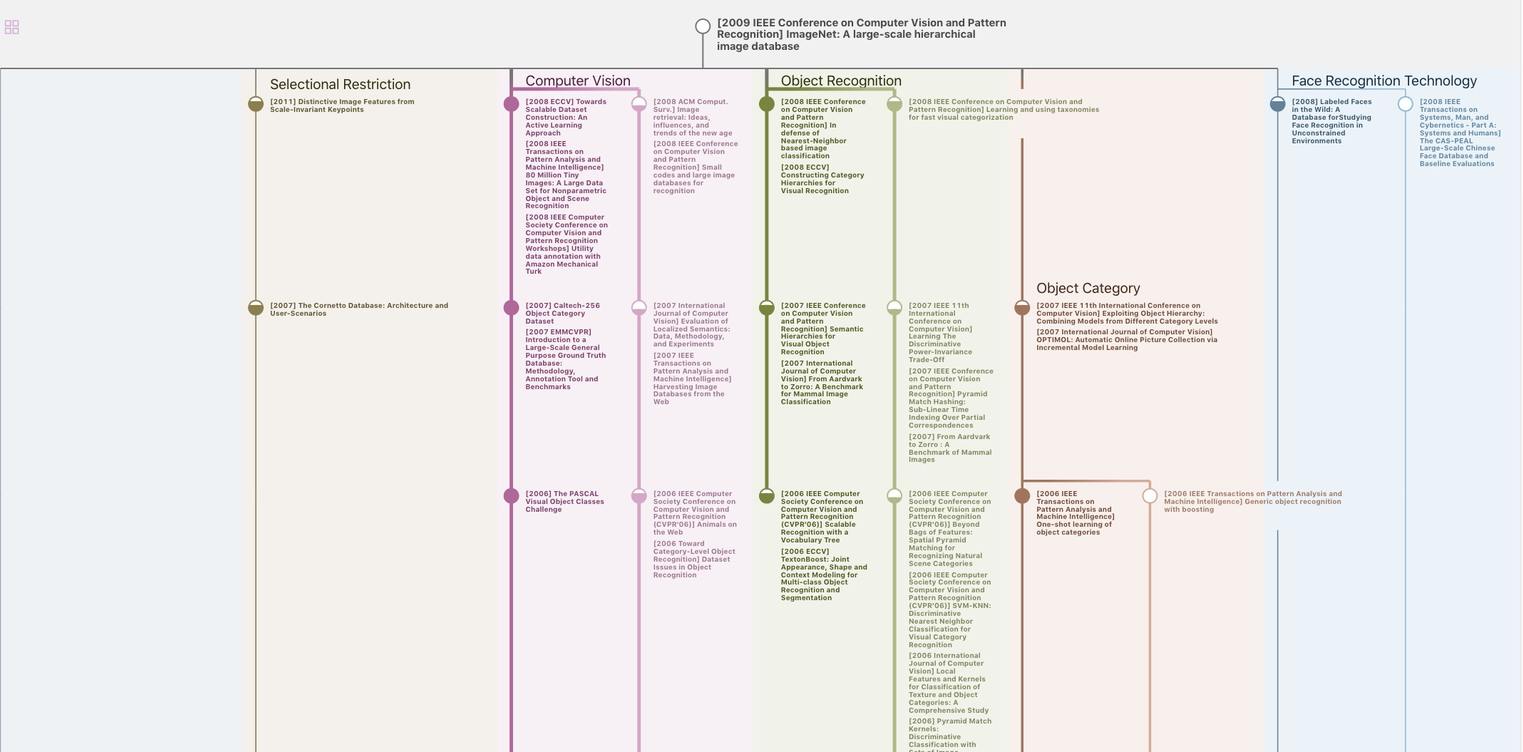
生成溯源树,研究论文发展脉络
Chat Paper
正在生成论文摘要