Text-Dependent Speaker Recognition with long-term features based on functional data analysis
ISCSLP(2012)
摘要
Text-Dependent Speaker Recognition (TDSR) is widely used nowadays. The short-term features like Mel-Frequency Cepstral Coefficient (MFCC) have been the dominant features used in traditional Dynamic Time Warping (DTW) based TDSR systems. The short-term features capture better local portion of the significant temporal dynamics but worse in overall sentence statistical characteristics. Functional Data Analysis (FDA) has been proven to show significant advantage in exploring the statistic information of data, so in this paper, a long-term feature extraction based on MFCC and FDA theory is proposed, where the extraction procedure consists of the following steps: Firstly, the FDA theory is applied after the MFCC feature extraction; Secondly, for the purpose of compressing the redundant data information, new feature based on the Functional Principle Component Analysis (FPCA) is generated; Thirdly, the distance between train features and test features is calculated for the use of the recognition procedure. Compared with the existing MFCC plus DTW method, experimental results show that the new features extracted with the proposed method plus the cosine similarity measure demonstrates better performance.
更多查看译文
关键词
dtw based tdsr systems,text-dependent speaker recognition,distance metrics,data analysis,mfcc feature extraction,speaker recognition,fda,long-term features,dynamic time warping,feature extraction,long-term feature extraction,statistic information,fpca,train features,functional data analysis,functional principle component analysis,test features,text analysis,principal component analysis,mel-frequency cepstral coefficient,tdsr
AI 理解论文
溯源树
样例
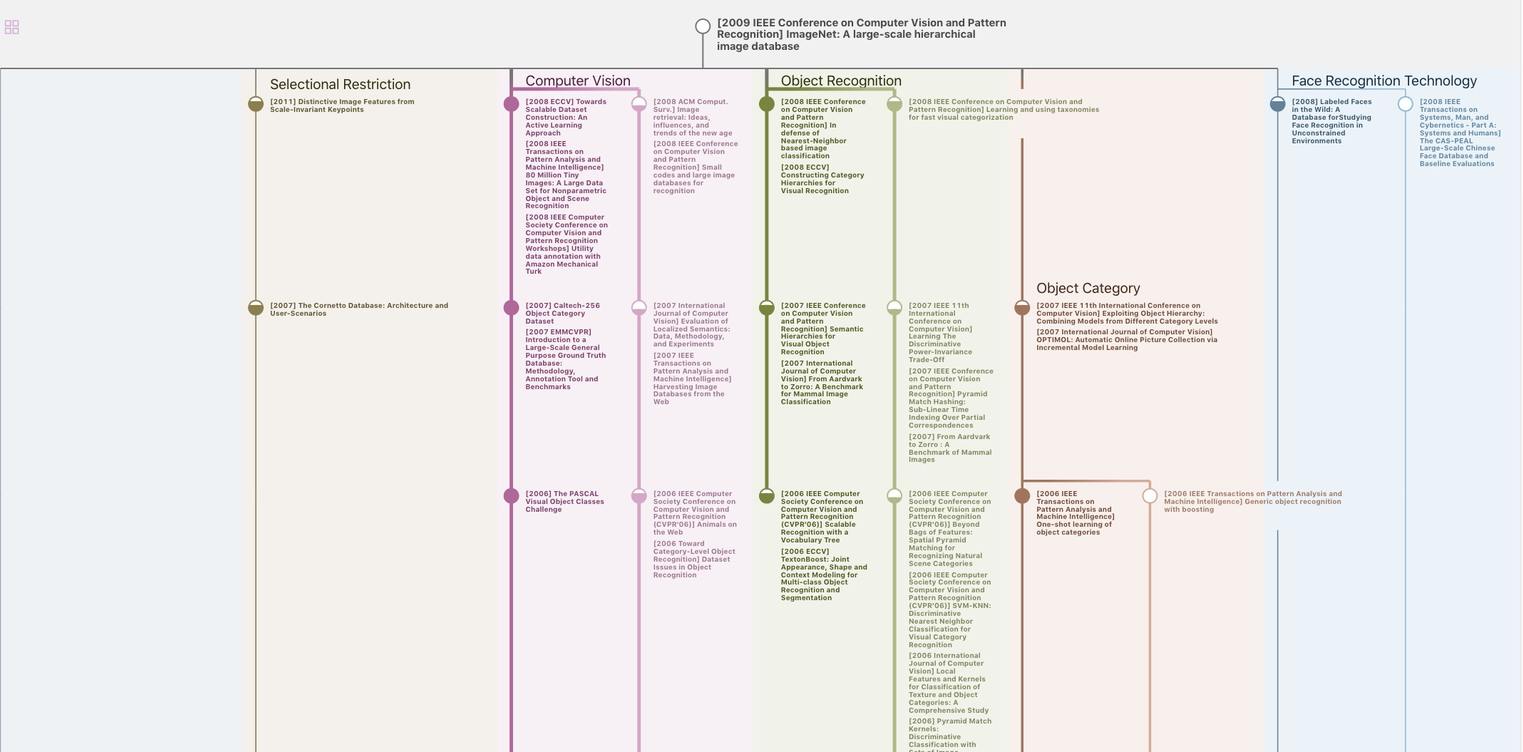
生成溯源树,研究论文发展脉络
Chat Paper
正在生成论文摘要