E-HowNet: the Expansion of HowNet
msra(2008)
Abstract
HowNet is an on-line common-sense knowledge base unveiling inter-conceptual relations and inter-attribute relations of concepts as connoting in lexicons of the Chinese and their English equivalents (1). Each concept is represented and understood by their definition and association links to other concepts. To Compare with WordNet, HowNet's architecture provides richer information apart from hyponymy relations. It also enriches relational links between words via encoded feature relations. The advantages of the HowNet are a) inherent properties of concepts are derived from encoded feature relations in addition to hypernym concepts, and b) information regarding conceptual differences between different concepts and information regarding morph-semantic structure are encoded. HowNet's advantages make it an effective electronic dictionary in NLP area. In recent years, HowNet has been applied to the researches of word similarity calculation(2), machine translation(3), and Information Retrieval(4) etc. However, what make us interest here is how to use HowNet to achieve mechanical natural language understanding. When we say that a sentence is 'understood', which means that the concepts and the conceptual relationships expressed by the sentence is unambiguously identified and we can make right inferences and/or responses. Similarly, computer understanding required a representational framework which suffices to represent knowledge about lexical concepts and to perform semantic composition and sense disambiguation processes. At present, HowNet has not focused on the aspect of semantic composition. We therefore propose a framework extended from HowNet, called E-HowNet, to deal with this problem. E-HowNet is a frame-based entity-relation model (5) which intends to achieve following goals: a. Word senses (concepts) can be defined by not only primitives but also any
MoreTranslated text
Key words
well-defined senses and sense relations. b. near canonical representations.
AI Read Science
Must-Reading Tree
Example
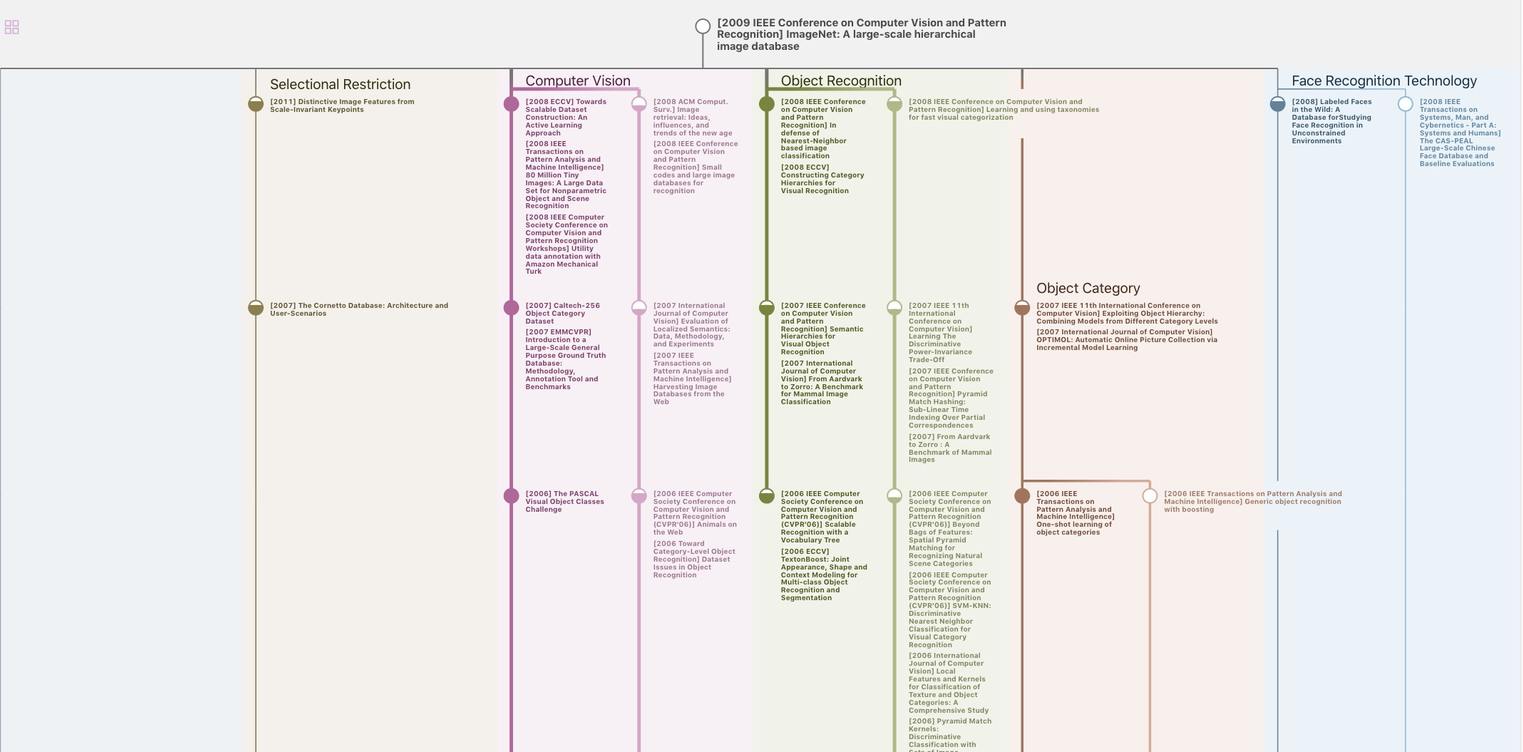
Generate MRT to find the research sequence of this paper
Chat Paper
Summary is being generated by the instructions you defined