Semantic Tie Points
WACV '13: Proceedings of the 2013 IEEE Workshop on Applications of Computer Vision (WACV)(2013)
摘要
Images for 3D mapping are always recorded in such a way that relevant scene parts are seen from multiple viewpoints, so as to facilitate camera orientation and 3D point triangulation. Beyond geometric reconstruction, automatic mapping also requires the semantic interpretation of the image content, and for that task the redundancy provided by overlapping images has been exploited much less. Here we address the task of learning a classifier for pixel-wise semantic labeling of the observed scene. The main insight is that the mere fact that two regions in different images depict the same 3D scene point yields a constraint which can be exploited in the learning phase, namely that they should receive the same class label, even if it is not known which one. In analogy to geometric "tie points"-image correspondences with a priori unknown 3D coordinates, which nevertheless constrain camera orientation-we call these correspondences "semantic tie points". We show how to integrate this weaker form of supervision, which is readily available in any multi-view dataset, into a random forest classifier, and demonstrate improved classification performance of the resulting classifier in an aerial dataset.
更多查看译文
关键词
image classification,image reconstruction,3D mapping images,3D point triangulation,aerial dataset classifier,automatic mapping,camera orientation,class label,classification,classifier learning,geometric reconstruction,image correspondences,multiview dataset,pixel-wise semantic scene labeling,random forest classifier,semantic image content interpretation,semantic tie points,
AI 理解论文
溯源树
样例
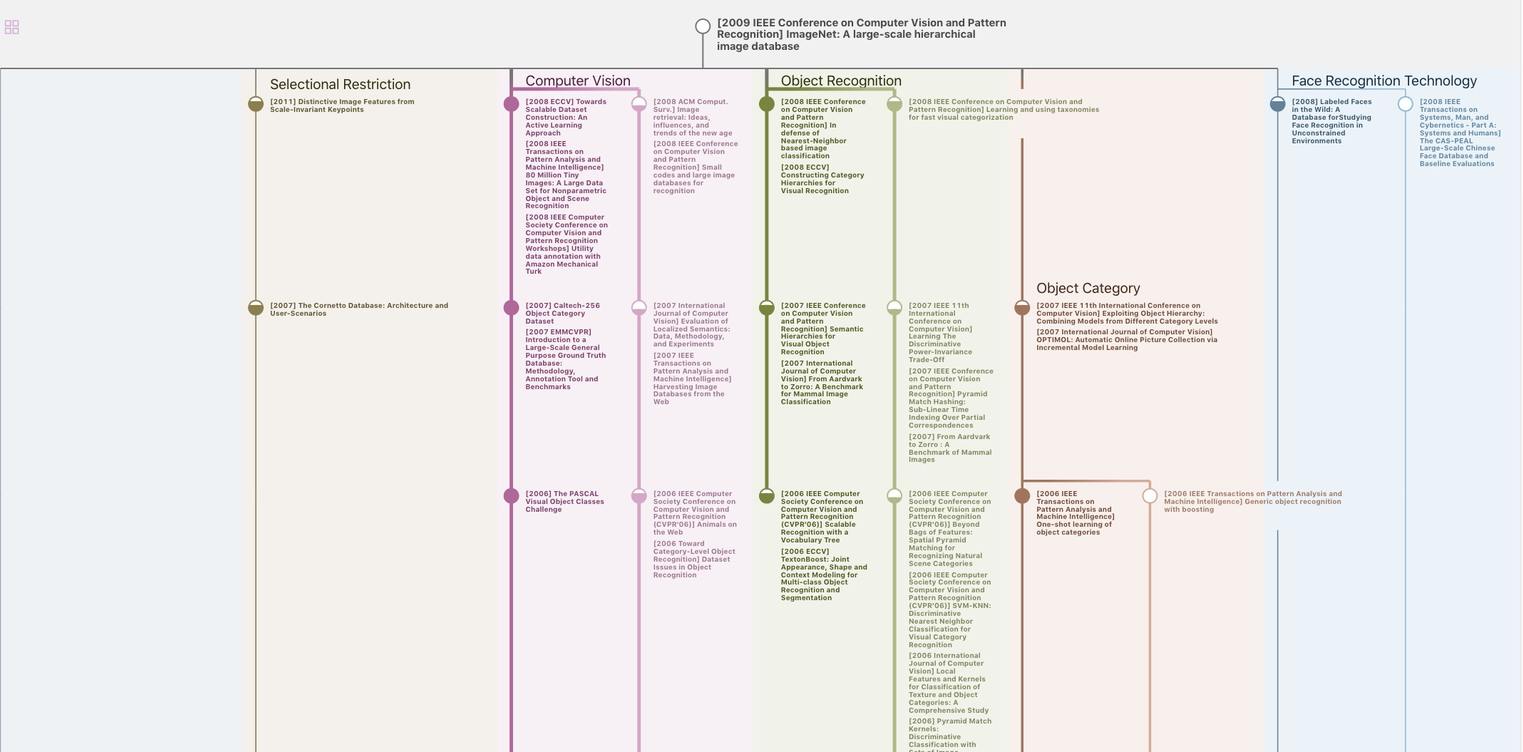
生成溯源树,研究论文发展脉络
Chat Paper
正在生成论文摘要