Understanding System Design For Big Data Workloads
IBM JOURNAL OF RESEARCH AND DEVELOPMENT(2013)
摘要
This paper explores the design and optimization implications for systems targeted at Big Data workloads. We confirm that these workloads differ from workloads typically run on more traditional transactional and data-warehousing systems in fundamental ways, and, therefore, a system optimized for Big Data can be expected to differ from these other systems. Rather than only studying the performance of representative computational kernels, and focusing on central-processing-unit performance, this paper studies the system as a whole. We identify three major phases in a typical Big Data workload, and we propose that each of these phases should be represented in a Big Data systems benchmark. We implemented our ideas on two distinct IBM POWER7 (R) processor-based systems that target different market sectors, and we analyze their performance on a sort benchmark. In particular, this paper includes an evaluation of POWER7 processor-based systems using MapReduce TeraSort, which is a workload that can be a "stress test" for multiple dimensions of system performance. We combine this work with a broader perspective on Big Data workloads and suggest a direction for a future benchmark definition effort. A number of methods to further improve system performance are proposed.
更多查看译文
关键词
Information management,Data handling,Data storage systems,Benchmark testing,System performance,Best practices,Optimization
AI 理解论文
溯源树
样例
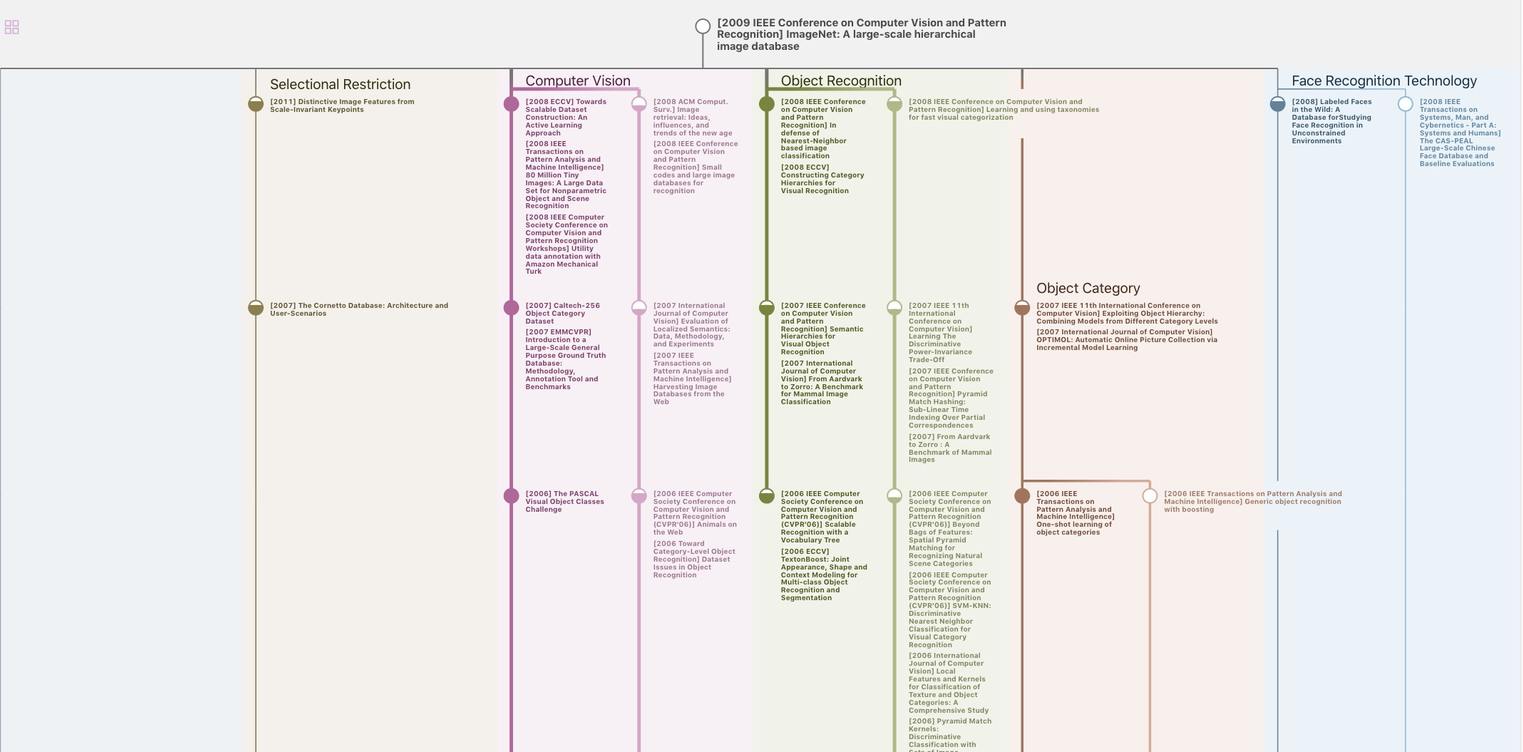
生成溯源树,研究论文发展脉络
Chat Paper
正在生成论文摘要