Dynamic Scene Deblurring
ICCV '13 Proceedings of the 2013 IEEE International Conference on Computer Vision(2013)
摘要
Most conventional single image deblurring methods assume that the underlying scene is static and the blur is caused by only camera shake. In this paper, in contrast to this restrictive assumption, we address the deblurring problem of general dynamic scenes which contain multiple moving objects as well as camera shake. In case of dynamic scenes, moving objects and background have different blur motions, so the segmentation of the motion blur is required for deblurring each distinct blur motion accurately. Thus, we propose a novel energy model designed with the weighted sum of multiple blur data models, which estimates different motion blurs and their associated pixel-wise weights, and resulting sharp image. In this framework, the local weights are determined adaptively and get high values when the corresponding data models have high data fidelity. And, the weight information is used for the segmentation of the motion blur. Non-local regularization of weights are also incorporated to produce more reliable segmentation results. A convex optimization-based method is used for the solution of the proposed energy model. Experimental results demonstrate that our method outperforms conventional approaches in deblurring both dynamic scenes and static scenes.
更多查看译文
关键词
general dynamic scenes,distinct blur motion,deblurring problem,general dynamic scene,motion blur,weights nonlocal regularization,motion blur segmentation,image segmentation,data fidelity,dynamic scene,blur motions,convex programming,image restoration,static scenes,different blur motion,pixel-wise weights,multiple blur data model,energy model,corresponding data model,convex optimization-based method,data models,cameras,dynamic scene deblurring,camera shake,different motion blur,image deblurring methods,multiple blur data models,image motion analysis
AI 理解论文
溯源树
样例
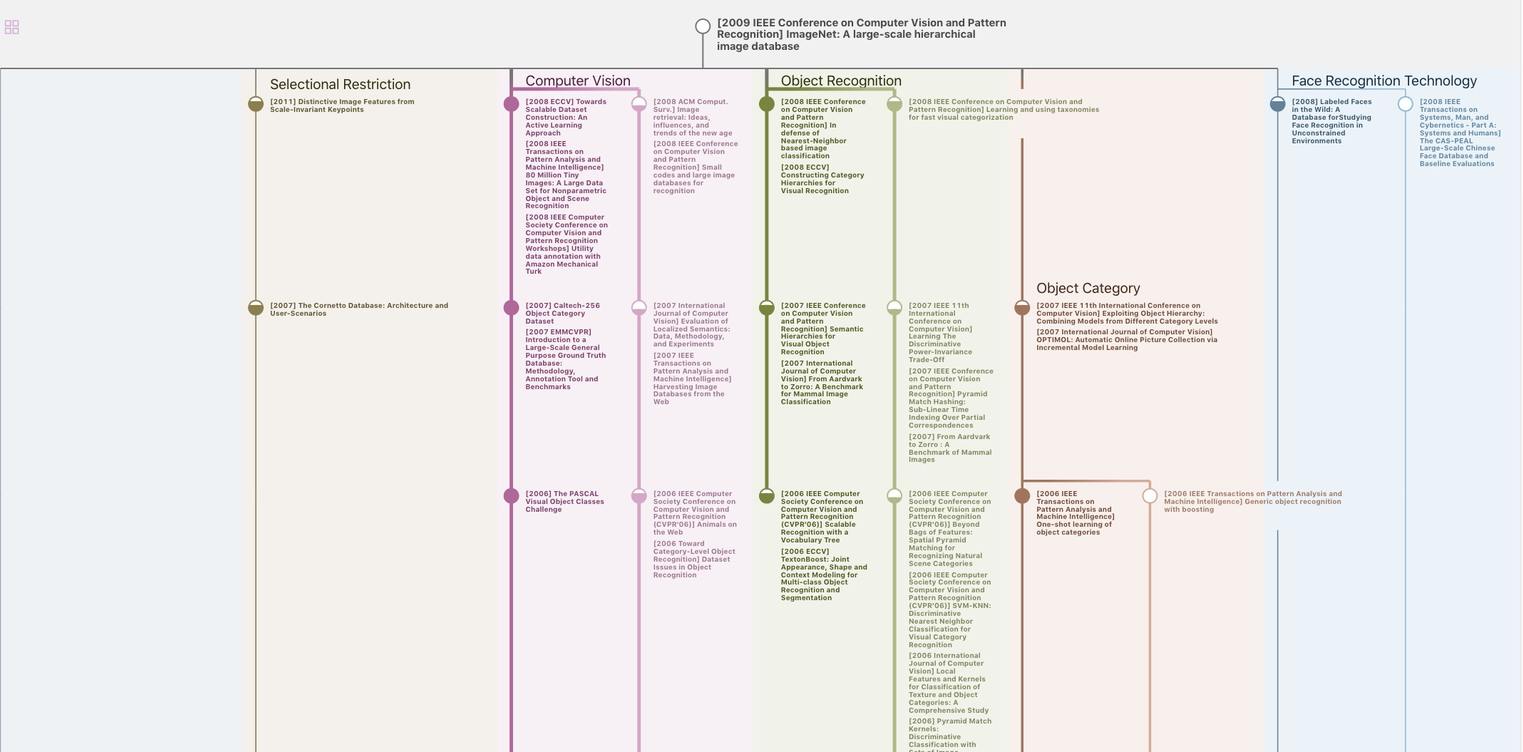
生成溯源树,研究论文发展脉络
Chat Paper
正在生成论文摘要