Testing the robustness of predictive models for chlorophyll generated from spaceborne imaging spectroscopy data for a mixedwood boreal forest canopy
INTERNATIONAL JOURNAL OF REMOTE SENSING(2014)
摘要
The amount of chlorophyll in a leaf influences photosynthetic potential and can be an indicator of the overall condition of a plant, including its stress level and nutritional status. Hence, it is important to understand the spatial and temporal variation of chlorophyll concentration. Imaging spectroscopy IS has made it possible to estimate chlorophyll at leaf and canopy levels. Spaceborne imaging spectrometers offer the possibility of estimating chlorophyll concentration at larger spatial scales and at lower cost than from direct sampling. We undertook this study in a mixedwood boreal forest to test the robustness of predictive models generated using Hyperion data for predicting chlorophyll concentration of data sets from different locations collected in different years. Among the group of indices tested, the derivative chlorophyll index DCI DCI = D705/D722 and the maximum derivative of the red-edge divided by the derivative of 703 nm Dmax680–750/D703 emerged as the best predictors of chlorophyll concentration across space and through time. When the canopy level chlorophyll predictive models of DCI and Dmax680–750/D703 derived from Hyperion data were applied to other years’ remote-sensing data acquired by airborne and spaceborne sensors, DCI explained 71%, 63%, and 6% and Dmax680–750/D703 explained 61%, 54%, and 8% of the variation in chlorophyll in 2002, 2004, and 2008, respectively, with prediction errors ranging from 11.7% to 14.6%. Two-variable models generated using 2005 Hyperion data were not as robust for predicting chlorophyll concentration from other years. Two models were found to explain more than half of the variance in chlorophyll concentration for the 2004 data only. Single and two-variable models applied to 2008 chlorophyll data provided poor predictions. The presence of multiple species creates a gradient in the chlorophyll concentration, which makes it possible to predict chlorophyll concentration. The gradient also affects the performance of predictive models generated using data from a different year. However, differences in sensors may also affect model performance. Our results suggest that predictive models obtained from Hyperion data are robust in predicting chlorophyll concentration within the same site through time and also at different sites across sensors.
更多查看译文
关键词
remote-sensing data,chlorophyll concentration,predictive model,chlorophyll data,spaceborne imaging spectroscopy data,hyperion data,two-variable model,canopy level chlorophyll,different year,derivative chlorophyll index,mixedwood boreal forest canopy
AI 理解论文
溯源树
样例
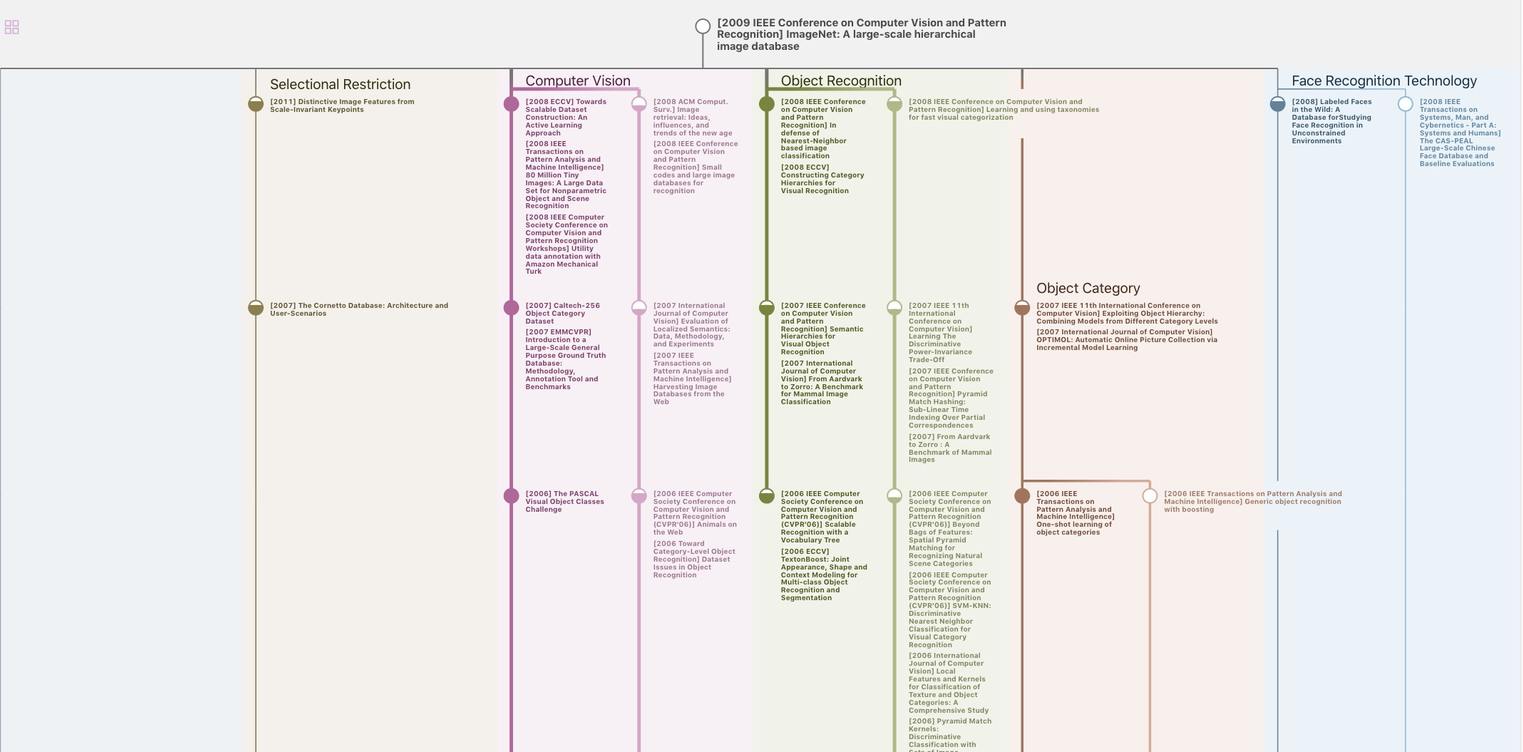
生成溯源树,研究论文发展脉络
Chat Paper
正在生成论文摘要