Modelling species abundance in a river by Negative Binomial hidden Markov models
Computational Statistics & Data Analysis(2014)
摘要
The investigation of species abundance in rivers involves data which are inherently sequential and unlikely to be fully independent. To take these characteristics into account, a Bayesian hierarchical model within the class of hidden Markov models is proposed to map the distribution of freshwater pearl mussels in the River Dee (Scotland). In order to model the overdispersed series of mussel counts, the conditional probability function of each observation, given the hidden state, is assumed to be Negative Binomial. Both the transition probabilities of the hidden Markov chain and the state-dependent means of the observed process depend on covariates obtained from a hydromorphological survey. Bayesian inference, model choice, and covariate selection based on Markov chain Monte Carlo algorithms are presented. The stochastic selection of the explanatory variables which are associated with a reduced chance of finding a local mussel population provides new evidence for the causes of the deterioration of a highly threatened species.
更多查看译文
关键词
Bayesian inference,Margaritifera margaritifera,Non-homogeneous Markov chain,River Dee,Variable selection
AI 理解论文
溯源树
样例
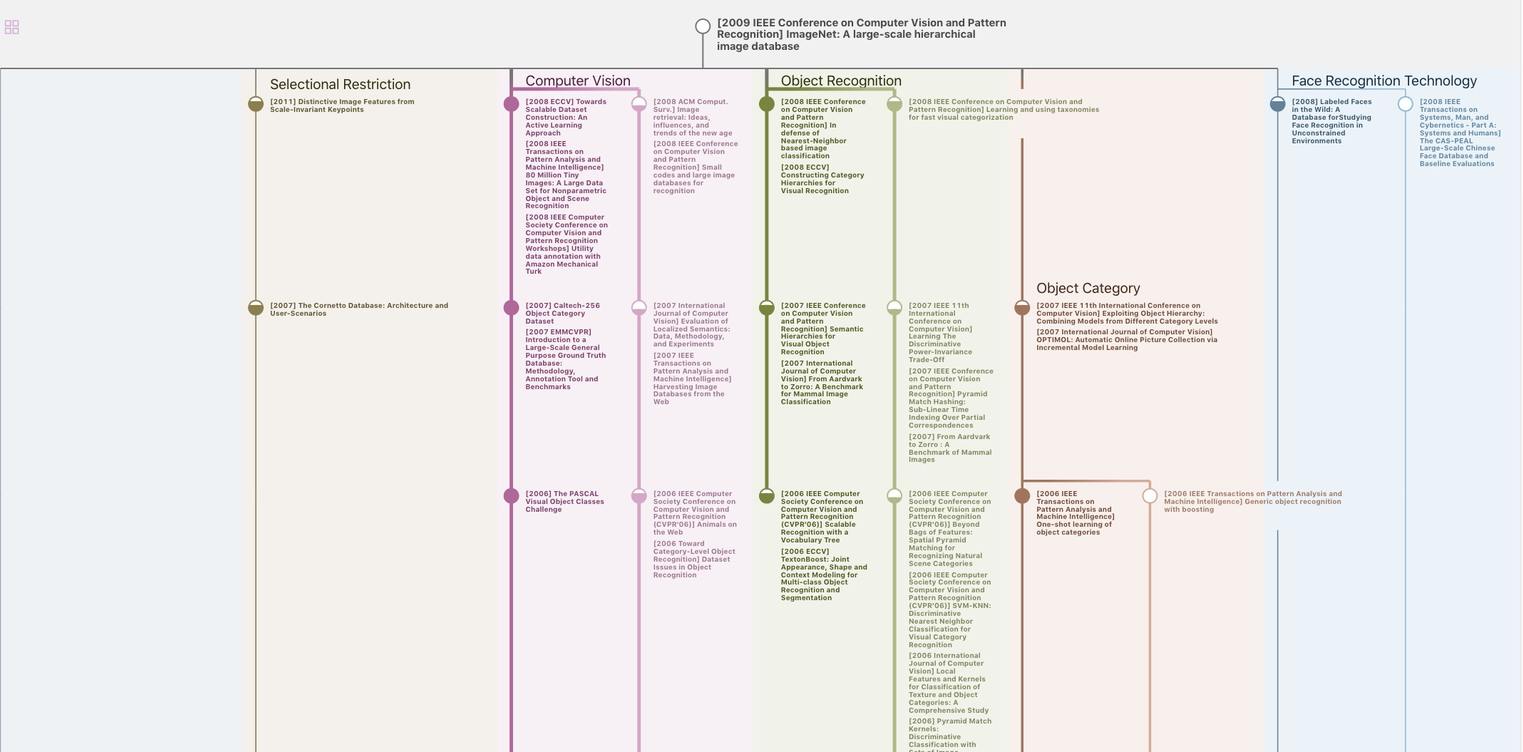
生成溯源树,研究论文发展脉络
Chat Paper
正在生成论文摘要