Optimal local searching for fast and robust textureless 3D object tracking in highly cluttered backgrounds.
IEEE Transactions on Visualization and Computer Graphics(2014)
摘要
Edge-based tracking is a fast and plausible approach for textureless 3D object tracking, but its robustness is still very challenging in highly cluttered backgrounds due to numerous local minima. To overcome this problem, we propose a novel method for fast and robust textureless 3D object tracking in highly cluttered backgrounds. The proposed method is based on optimal local searching of 3D-2D correspondences between a known 3D object model and 2D scene edges in an image with heavy background clutter. In our searching scheme, searching regions are partitioned into three levels (interior, contour, and exterior) with respect to the previous object region, and confident searching directions are determined by evaluating candidates of correspondences on their region levels; thus, the correspondences are searched among likely candidates in only the confident directions instead of searching through all candidates. To ensure the confident searching direction, we also adopt the region appearance, which is efficiently modeled on a newly defined local space (called a searching bundle). Experimental results and performance evaluations demonstrate that our method fully supports fast and robust textureless 3D object tracking even in highly cluttered backgrounds.
更多查看译文
关键词
confident direction,textureless 3d object tracking,cluttered background,robust textureless,highly cluttered backgrounds,novel method,local minima,numerous local minimum,heavy background clutter,model-based tracking,3d-2d correspondences,3d object model,object model,region knowledge,previous object region,exterior level,searching bundle,optimal local searching,edge-based tracking,local searching,contour level,background clutter,object tracking,edge detection,2d scene edges,solid modelling,local space,image texture,region appearance,interior level
AI 理解论文
溯源树
样例
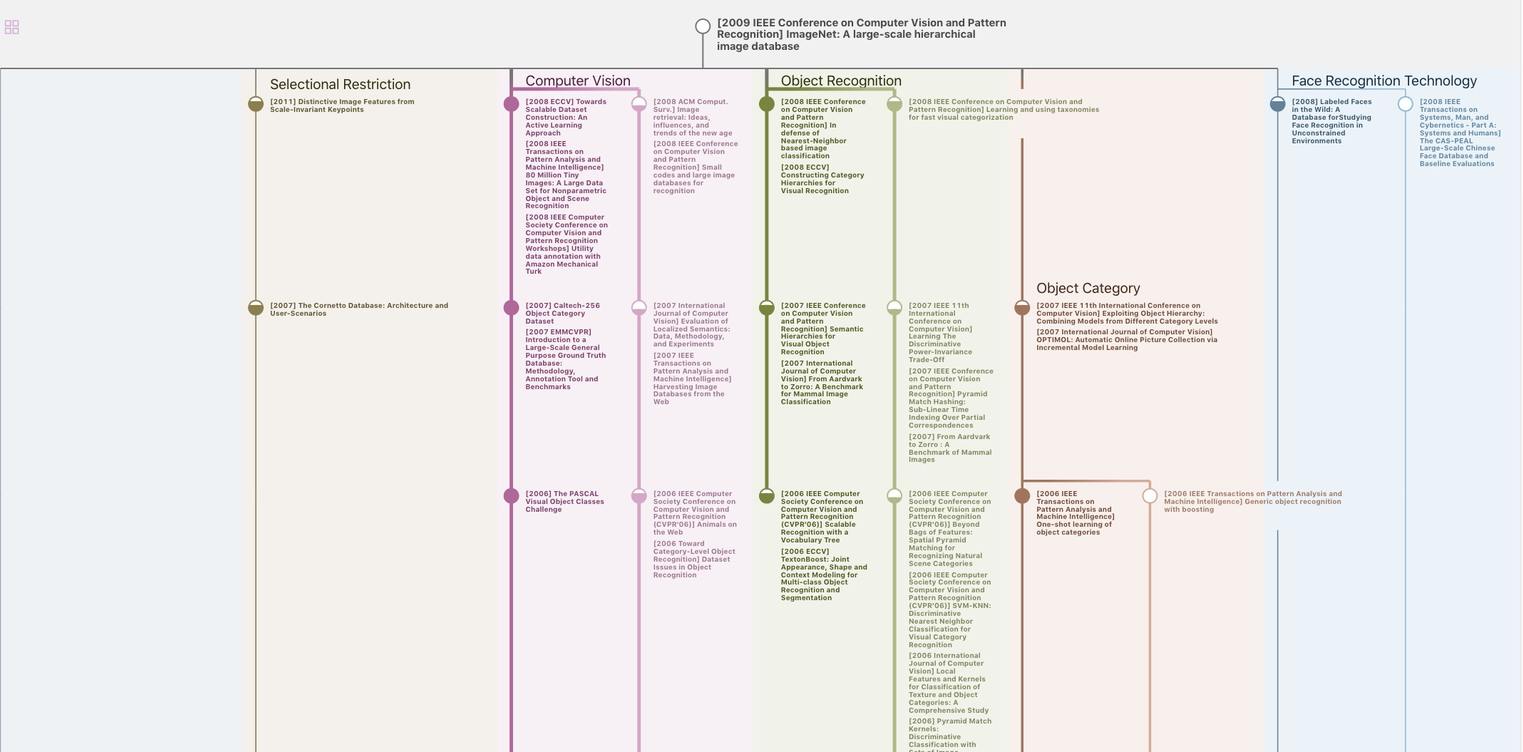
生成溯源树,研究论文发展脉络
Chat Paper
正在生成论文摘要