A granular computing-based approach to credit scoring modeling
Neurocomputing(2013)
Abstract
The credit card industry has been growing rapidly and thus huge numbers of consumers' credit data are collected by the credit department of the banks. The credit scoring managers often evaluate the consumer's credit with intuitive experience. However, with the support of the credit classification models, the managers can accurately evaluate the applicants' credit score. In this study, a neurocomputing-based granular approach is proposed to model credit scoring. Granular computing is used to compute the size of training and testing groups. Artificial neural networks (ANN) and data envelopment analysis (DEA) are used to model credit lending decisions in the online and offline manner, respectively. Proposed method is composed of three distinct stages based on trust and credibility concept. Trust is introduced and modeled via ANN in online module. Also credibility is modeled via DEA in offline module in present study. This paper is a pioneer in examining the concept of granularity for selecting the optimum size of testing and training group in machine learning area. In addition, proposing flexible trust ranges comparing to the current constant ones will support the importance of customers with higher credit scores to financial markets. To show the applicability and superiority of the proposed algorithm, it is applied to a credit-card data set obtained from the UCI repository.
MoreTranslated text
Key words
credit card industry,credit classification model,credit data,model credit scoring,higher credit score,credit score,scoring modeling,credit department,data envelopment analysis,credit-card data,granular computing-based approach,model credit lending decision,granular computing,trust
AI Read Science
Must-Reading Tree
Example
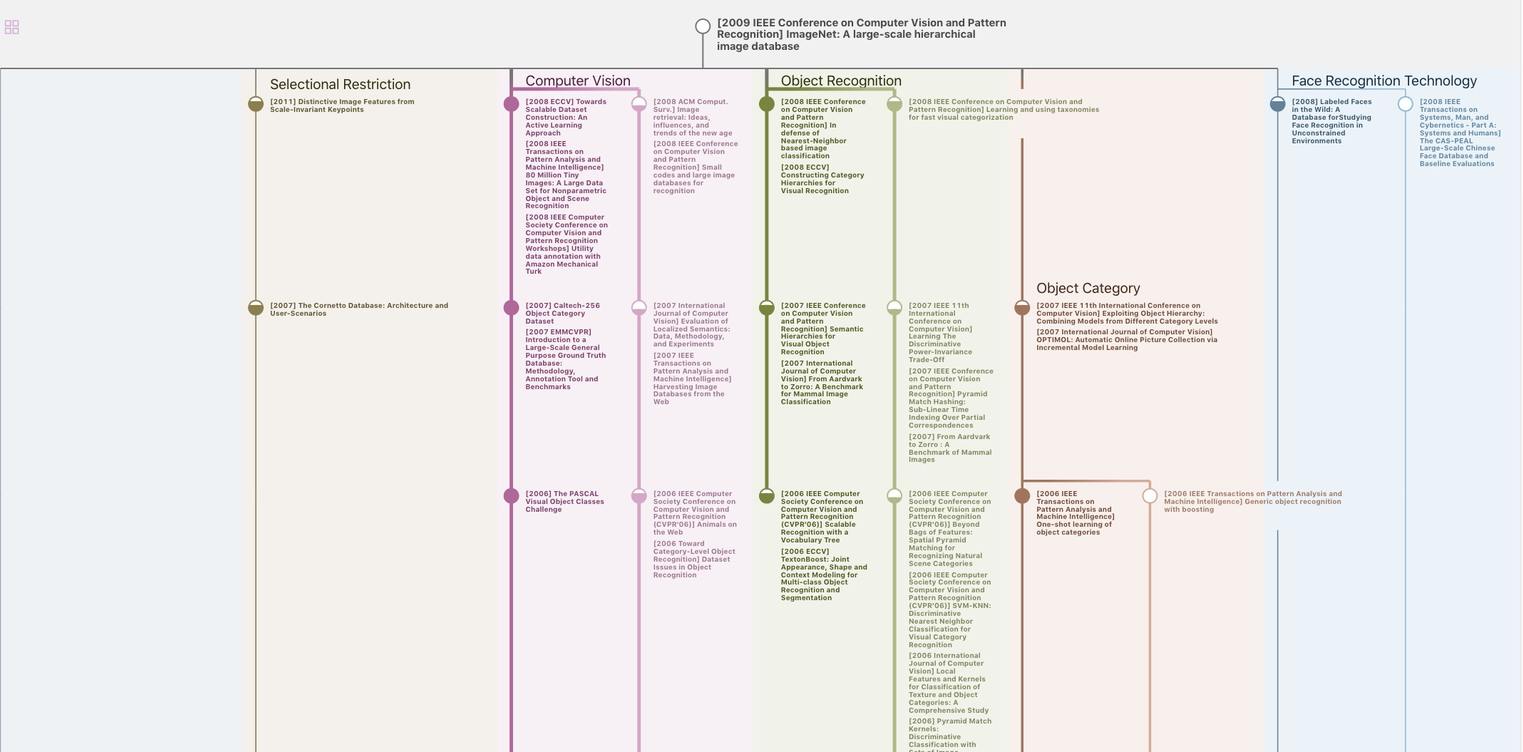
Generate MRT to find the research sequence of this paper
Chat Paper
Summary is being generated by the instructions you defined