Configurable hardware integrate and fire neurons for sparse approximation.
Periodicals(2013)
摘要
Sparse approximation is an important optimization problem in signal and image processing applications. A Hopfield-Network-like system of integrate and fire (IF) neurons is proposed as a solution, using the Locally Competitive Algorithm (LCA) to solve an overcomplete L1 sparse approximation problem. A scalable system architecture is described, including IF neurons with a nonlinear firing function, and current-based synapses to provide linear computation. A network of 18 neurons with 12 inputs is implemented on the RASP 2.9v chip, a Field Programmable Analog Array (FPAA) with directly programmable floating gate elements. Said system uses over 1400 floating gates, the largest system programmed on a FPAA to date. The circuit successfully reproduced the outputs of a digital optimization program, converging to within 4.8% RMS, and an objective cost only 1.7% higher on average. The active circuit consumed 559 μA of current at 2.4 V and converges on solutions in 25 μs, with measurement of the converged spike rate taking an additional 1 ms. Extrapolating the scaling trends to a N=1000 node system, the spiking LCA compares favorably with state-of-the-art digital solutions, and analog solutions using a non-spiking approach.
更多查看译文
关键词
Sparse approximation,FPAA,Integrate and fire neurons,Nonlinear optimization,LCA
AI 理解论文
溯源树
样例
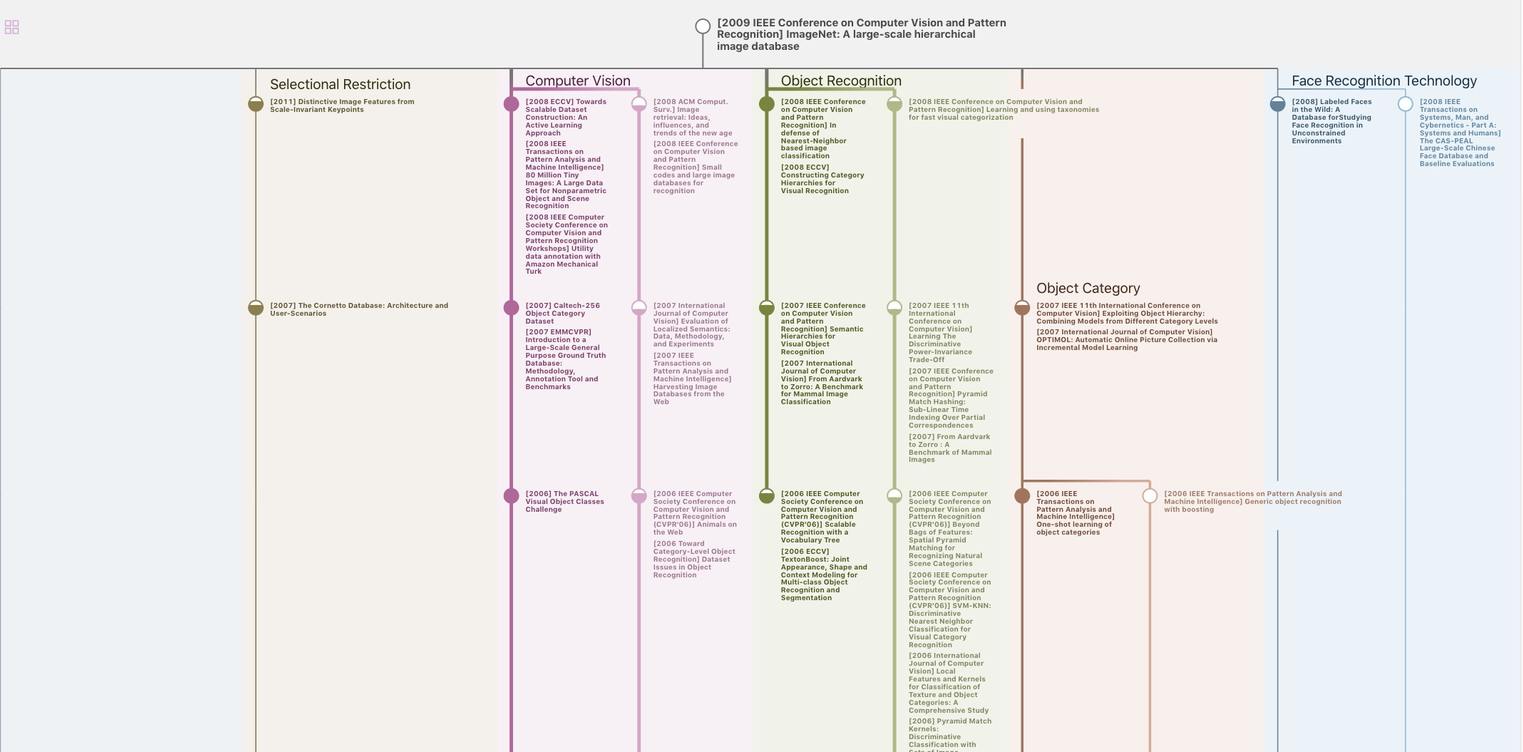
生成溯源树,研究论文发展脉络
Chat Paper
正在生成论文摘要