Multi-view hypergraph learning by patch alignment framework
Neurocomputing(2013)
摘要
Graph-based methods are currently popular for dimensionality reduction. However, most of them suffer from over-simplified assumption of pairwise relationships among data. Especially for multi-view data, different relationships from different views are hard to be integrated into a single graph. In this paper, we propose a novel semi-supervised dimensionality reduction method for multi-view data. First, we assume the hyperedges in hypergraph as patches and apply hypergraph to the patch alignment framework. Second, the weights of the hyperedges are computed with statistics of distances between neighboring pairs and the patches from different views are integrated. In this way, we construct Multi-view Hypergraph Laplacian matrix and we get the dimensionality-reduced data by solving the standard eigen-decomposition to obtain the projection matrix. The experimental results demonstrate the effectiveness of the proposed method on retrieval performance.
更多查看译文
关键词
patch alignment framework,different view,multi-view hypergraph,projection matrix,different relationship,dimensionality reduction method,graph-based method,multi-view data,multi-view hypergraph laplacian matrix,dimensionality reduction,dimensionality-reduced data
AI 理解论文
溯源树
样例
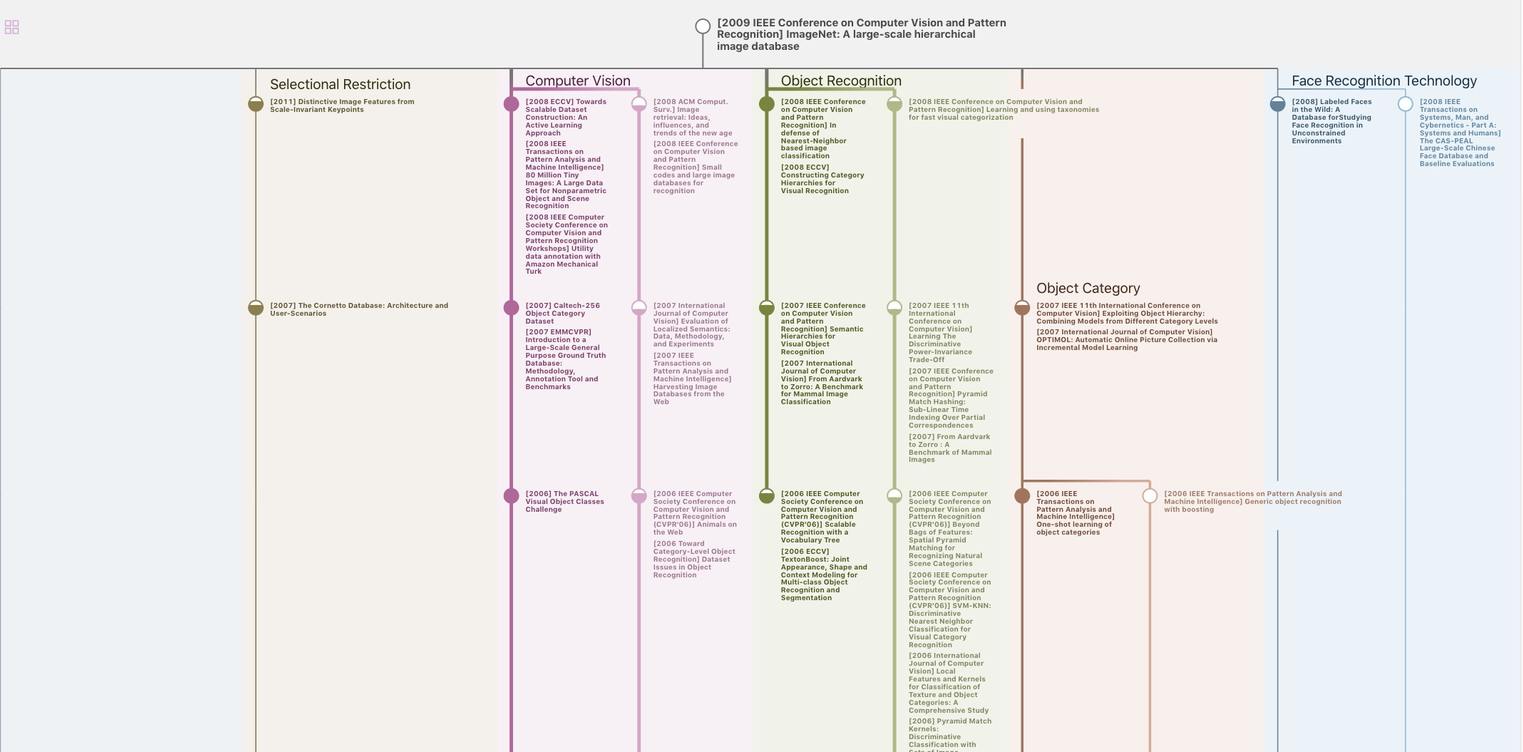
生成溯源树,研究论文发展脉络
Chat Paper
正在生成论文摘要