Effective detection of sophisticated online banking fraud on extremely imbalanced data
World Wide Web(2012)
摘要
Sophisticated online banking fraud reflects the integrative abuse of resources in social, cyber and physical worlds. Its detection is a typical use case of the broad-based Wisdom Web of Things (W2T) methodology. However, there is very limited information available to distinguish dynamic fraud from genuine customer behavior in such an extremely sparse and imbalanced data environment, which makes the instant and effective detection become more and more important and challenging. In this paper, we propose an effective online banking fraud detection framework that synthesizes relevant resources and incorporates several advanced data mining techniques. By building a contrast vector for each transaction based on its customer’s historical behavior sequence, we profile the differentiating rate of each current transaction against the customer’s behavior preference. A novel algorithm, ContrastMiner, is introduced to efficiently mine contrast patterns and distinguish fraudulent from genuine behavior, followed by an effective pattern selection and risk scoring that combines predictions from different models. Results from experiments on large-scale real online banking data demonstrate that our system can achieve substantially higher accuracy and lower alert volume than the latest benchmarking fraud detection system incorporating domain knowledge and traditional fraud detection methods.
更多查看译文
关键词
fraud detection,online banking,contrast pattern,neural network,data mining
AI 理解论文
溯源树
样例
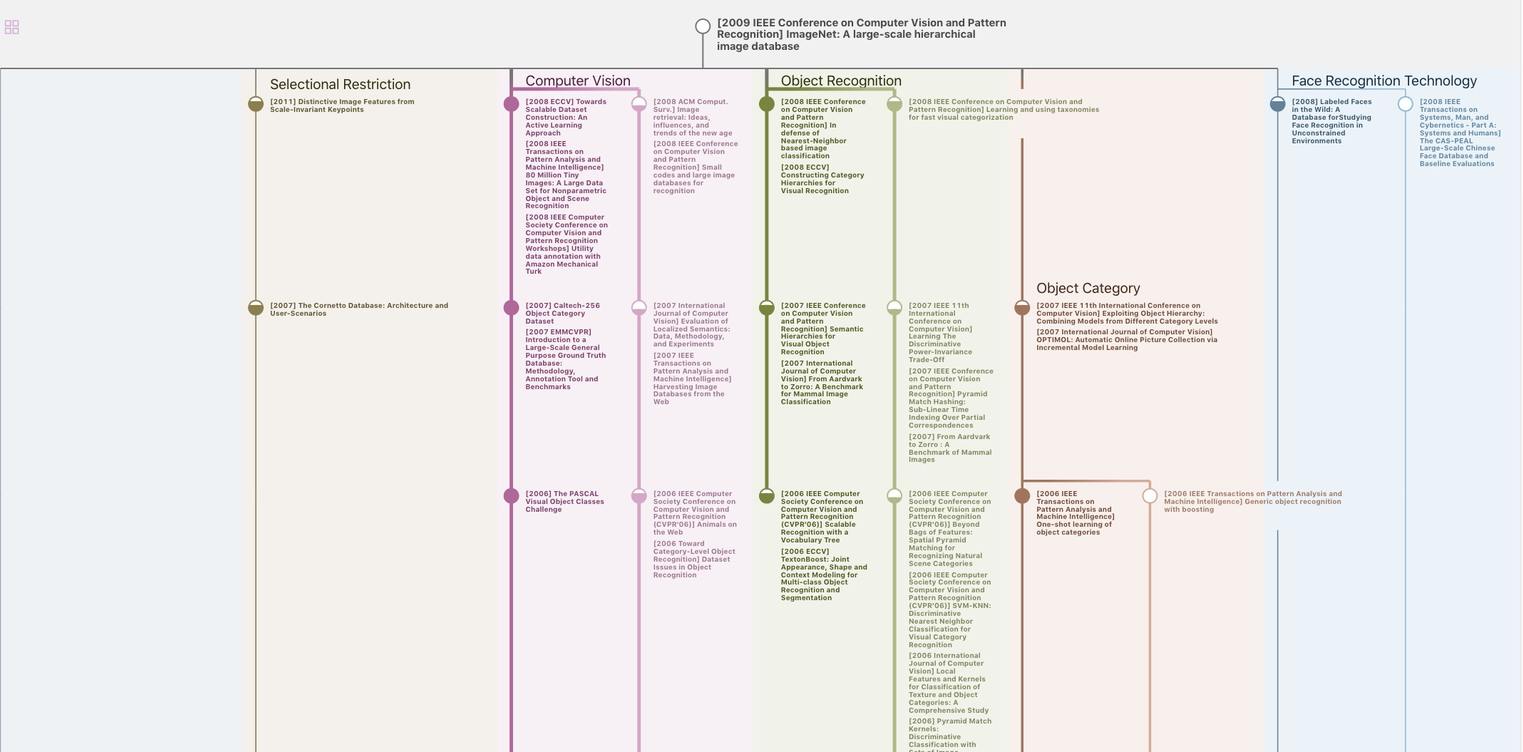
生成溯源树,研究论文发展脉络
Chat Paper
正在生成论文摘要