Employing the Principal Hessian Direction for Building Hinging Hyperplane Models
Data Mining Workshops(2012)
摘要
In this paper we address the problem of identifying a continuous nonlinear model from a set of discrete observations. The goal is to build a compact and accurate model of an underlying process, which is interpretable by the user, and can be also used for prediction purposes. Hinging hyper plane models are well suited to represent continuous piecewise linear models, but the hinge finding algorithm is guaranteed to converge only in local optima, and hence heavily depends on the initialization. We employ the principal Hessian direction to incorporate the geometrical information of the regression surface in the hinge finding process and can thus avoid the several random initializations proposed in the literature.
更多查看译文
关键词
underlying process,accurate model,prediction purpose,principal hessian direction,local optimum,continuous nonlinear model,building hinging hyperplane models,geometrical information,discrete observation,hyper plane model,continuous piecewise linear model,regression analysis,computational geometry,identification
AI 理解论文
溯源树
样例
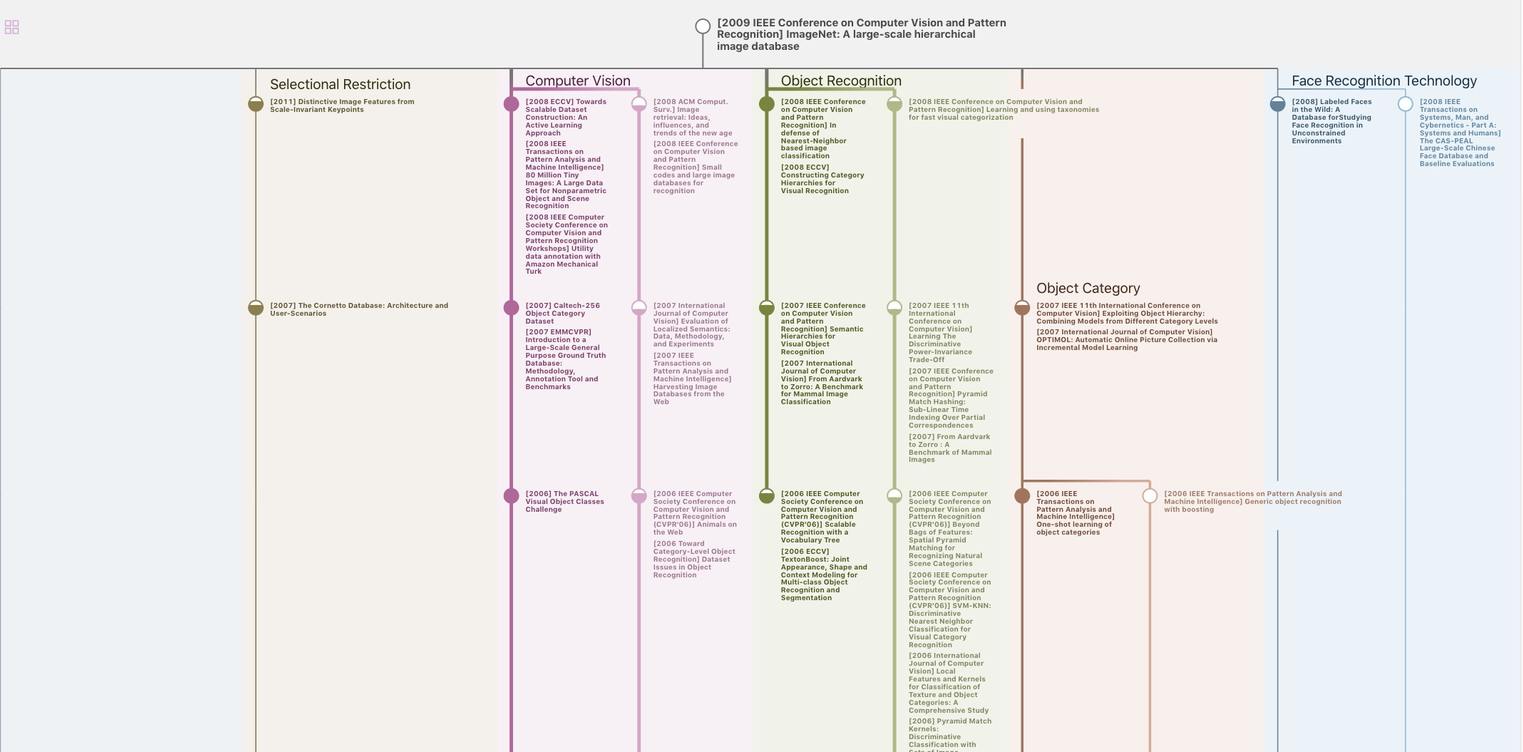
生成溯源树,研究论文发展脉络
Chat Paper
正在生成论文摘要