LA-LDA: a limited attention topic model for social recommendation
SBP'13 Proceedings of the 6th international conference on Social Computing, Behavioral-Cultural Modeling and Prediction(2013)
摘要
Social media users have finite attention which limits the number of incoming messages from friends they can process. Moreover, they pay more attention to opinions and recommendations of some friends more than others. In this paper, we propose $\mathcal LA$-LDA, a latent topic model which incorporates limited, non-uniformly divided attention in the diffusion process by which opinions and information spread on the social network. We show that our proposed model is able to learn more accurate user models from users' social network and item adoption behavior than models which do not take limited attention into account. We analyze voting on news items on the social news aggregator Digg and show that our proposed model is better able to predict held out votes than alternative models. Our study demonstrates that psycho-socially motivated models have better ability to describe and predict observed behavior than models which only consider topics.
更多查看译文
关键词
social media user,social network,social news aggregator,finite attention,psycho-socially motivated model,social recommendation,latent topic model,alternative model,accurate user model,limited attention topic model,limited attention,social media,diffusion,link analysis
AI 理解论文
溯源树
样例
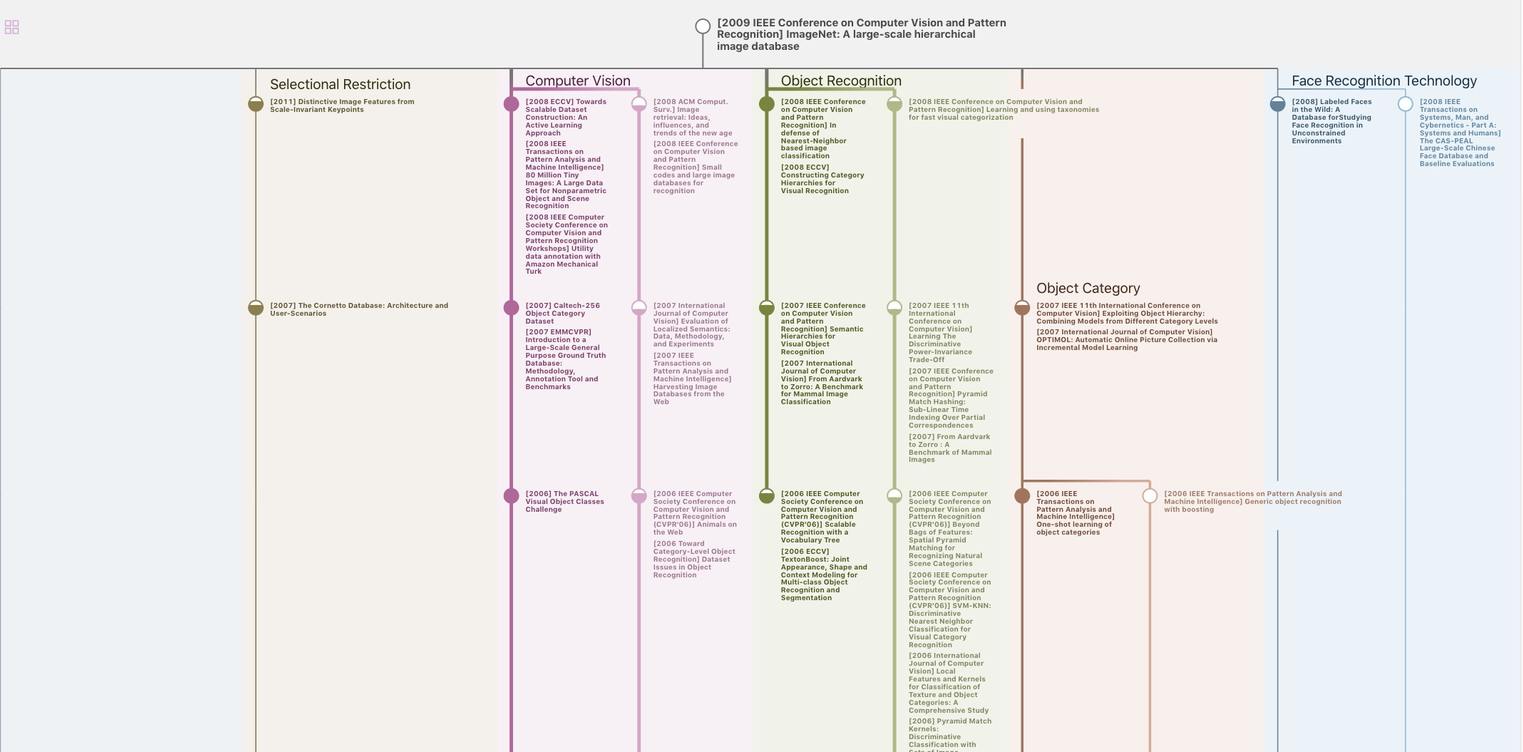
生成溯源树,研究论文发展脉络
Chat Paper
正在生成论文摘要